Polyhedral Conic Classifiers For Visual Object Detection And Classification
30TH IEEE CONFERENCE ON COMPUTER VISION AND PATTERN RECOGNITION (CVPR 2017)(2017)
摘要
We propose a family of quasi-linear discriminants that outperform current large-margin methods in sliding window visual object detection and open set recognition tasks. In these tasks the classification problems are both numerically imbalanced-positive (object class) training and test windows are much rarer than negative (non-class) ones and geometrically asymmetric-the positive samples typically form compact, visually-coherent groups while negatives are much more diverse, including anything at all that is not a well-centred sample from the target class. It is difficult to cover such negative classes using training samples, and doubly so in 'open set' applications where run-time negatives may stem from classes that were not seen at all during training. So there is a need for discriminants whose decision regions focus on tightly circumscribing the positive class, while still taking account of negatives in zones where the two classes overlap. This paper introduces a family of quasi-linear "polyhedral conic" discriminants whose positive regions are distorted L1 balls. The methods have properties and run-time complexities comparable to linear Support Vector Machines (SVMs), and they can be trained from either binary or positive-only samples using constrained quadratic programs related to SVMs. Our experiments show that they significantly outperform both linear SVMs and existing one-class discriminants on a wide range of object detection, open set recognition and conventional closed-set classification tasks.
更多查看译文
关键词
quasilinear discriminants,large-margin methods,window visual object detection,open set recognition tasks,positive training,object class,test windows,positive samples,visually-coherent groups,well-centred sample,target class,negative classes,training samples,open set applications,runtime negatives,positive class,quasilinear polyhedral conic discriminants whose positive regions,classification tasks,polyhedral conic classifiers,classification problems,decision regions,linear SVM,one-class discriminants
AI 理解论文
溯源树
样例
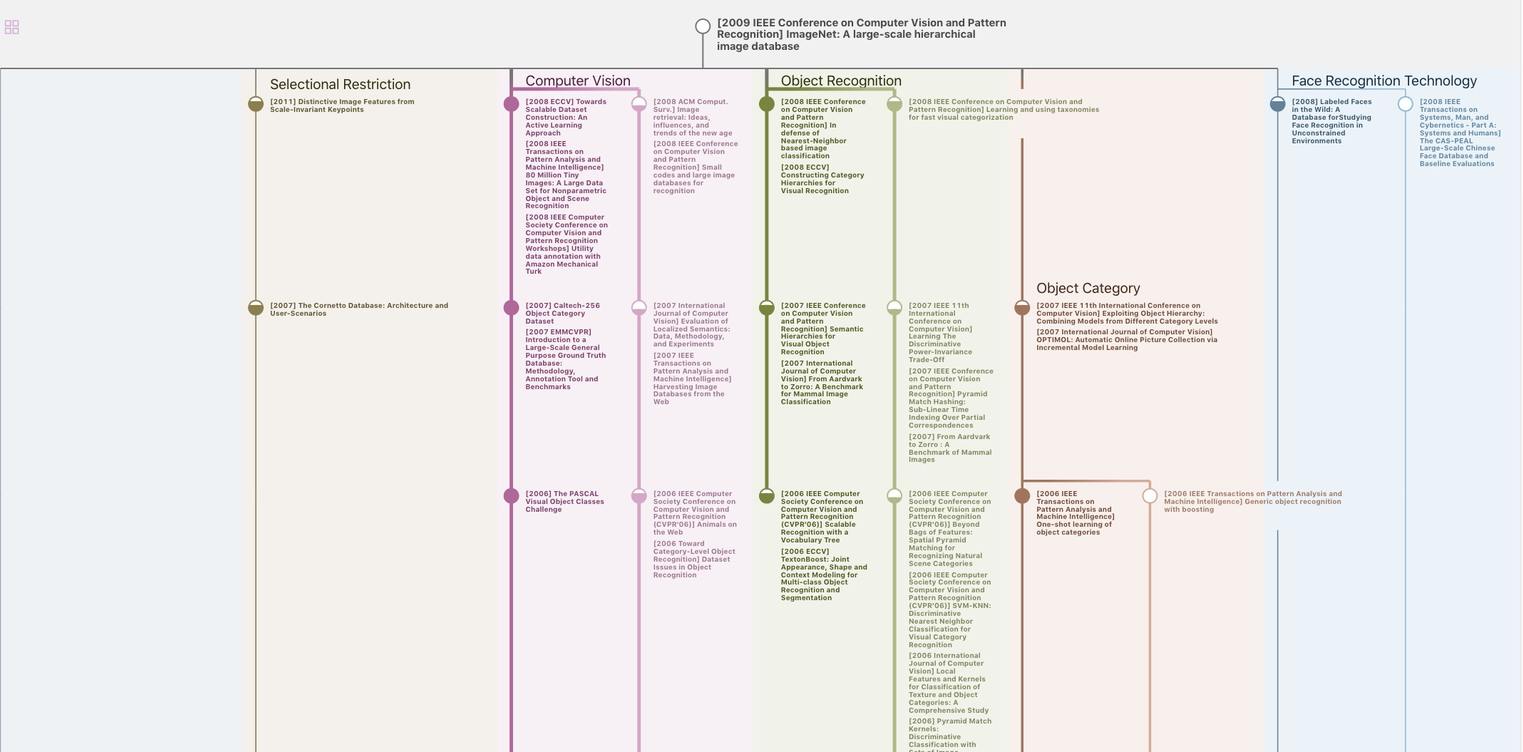
生成溯源树,研究论文发展脉络
Chat Paper
正在生成论文摘要