Comparison of machine learning methods for stationary wavelet entropy-based multiple sclerosis detection
Simulation(2016)
摘要
In order to detect multiple sclerosis MS subjects from healthy controls HCs in magnetic resonance imaging, we developed a new system based on machine learning. The MS imaging data was downloaded from the eHealth laboratory at the University of Cyprus, and the HC imaging data was scanned in our local hospital with volunteers enrolled from community advertisement. Inter-scan normalization was employed to remove the gray-level difference. We adjust the misclassification costs to alleviate the effect of unbalanced class distribution to the classification performance. We utilized two-level stationary wavelet entropy SWE to extract features from brain images. Then, we compared three machine learning based classifiers: the decision tree, k-nearest neighbors kNN, and support vector machine. The experimental results showed the kNN performed the best among all three classifiers. In addition, the proposed SWE+kNN approach is superior to four state-of-the-art approaches. Our proposed MS detection approach is effective.
更多查看译文
关键词
Multiple sclerosis,stationary wavelet entropy,decision tree,k-nearest neighbors,support vector machine,machine learning
AI 理解论文
溯源树
样例
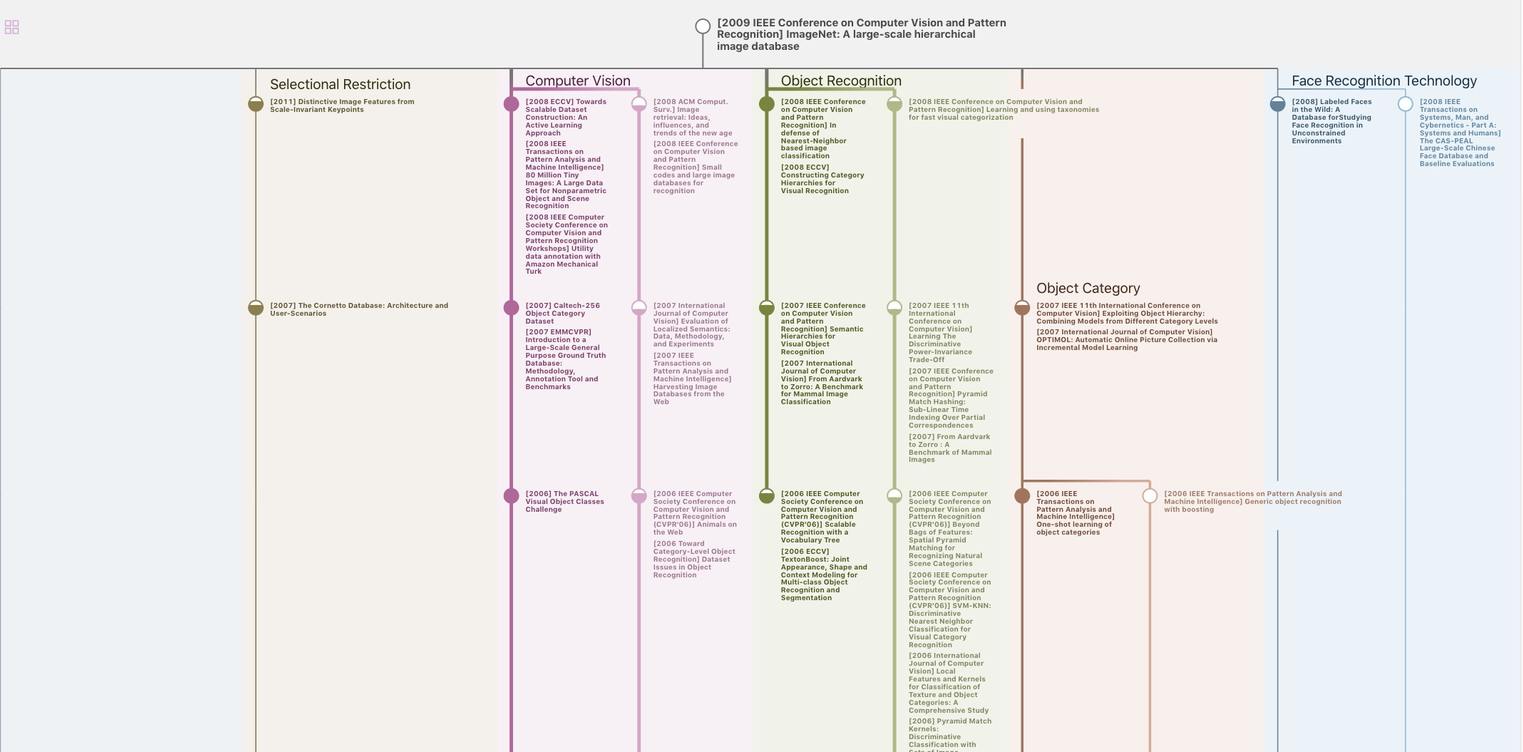
生成溯源树,研究论文发展脉络
Chat Paper
正在生成论文摘要