Automatic Video Description Generation Via Lstm With Joint Two-Stream Encoding
2016 23RD INTERNATIONAL CONFERENCE ON PATTERN RECOGNITION (ICPR)(2016)
摘要
In this paper, we propose a novel two-stream framework based on combinational deep neural networks. The framework is mainly composed of two components: one is a parallel two-stream encoding component which learns video encoding from multiple sources using 3D convolutional neural networks and the other is a long-short-term-memory (LSTM)-based decoding language model which transfers the input encoded video representations to text descriptions. The merits of our proposed model are: 1) It extracts both temporal and spatial features by exploring the usage of 3D convolutional networks on both raw RGB frames and motion history images. 2) Our model can dynamically tune the weights of different feature channels since the network is trained end-to-end from learning combinational encoding of multiple features to LSTM-based language model. Our model is evaluated on three public video description datasets: one YouTube clips dataset (Microsoft Video Description Corpus) and two large movie description datasets (MPII Corpus and Montreal Video Annotation Dataset) and achieves comparable or better performance than the state-of-the-art approaches in video caption generation.
更多查看译文
关键词
motion history images,RGB frames,spatial feature extraction,temporal feature extraction,text descriptions,video representations,LSTM-based decoding language model,long-short-term-memory,3D convolutional neural networks,video encoding,parallel two-stream encoding component,combinational deep neural networks,joint two-stream encoding,automatic video description generation
AI 理解论文
溯源树
样例
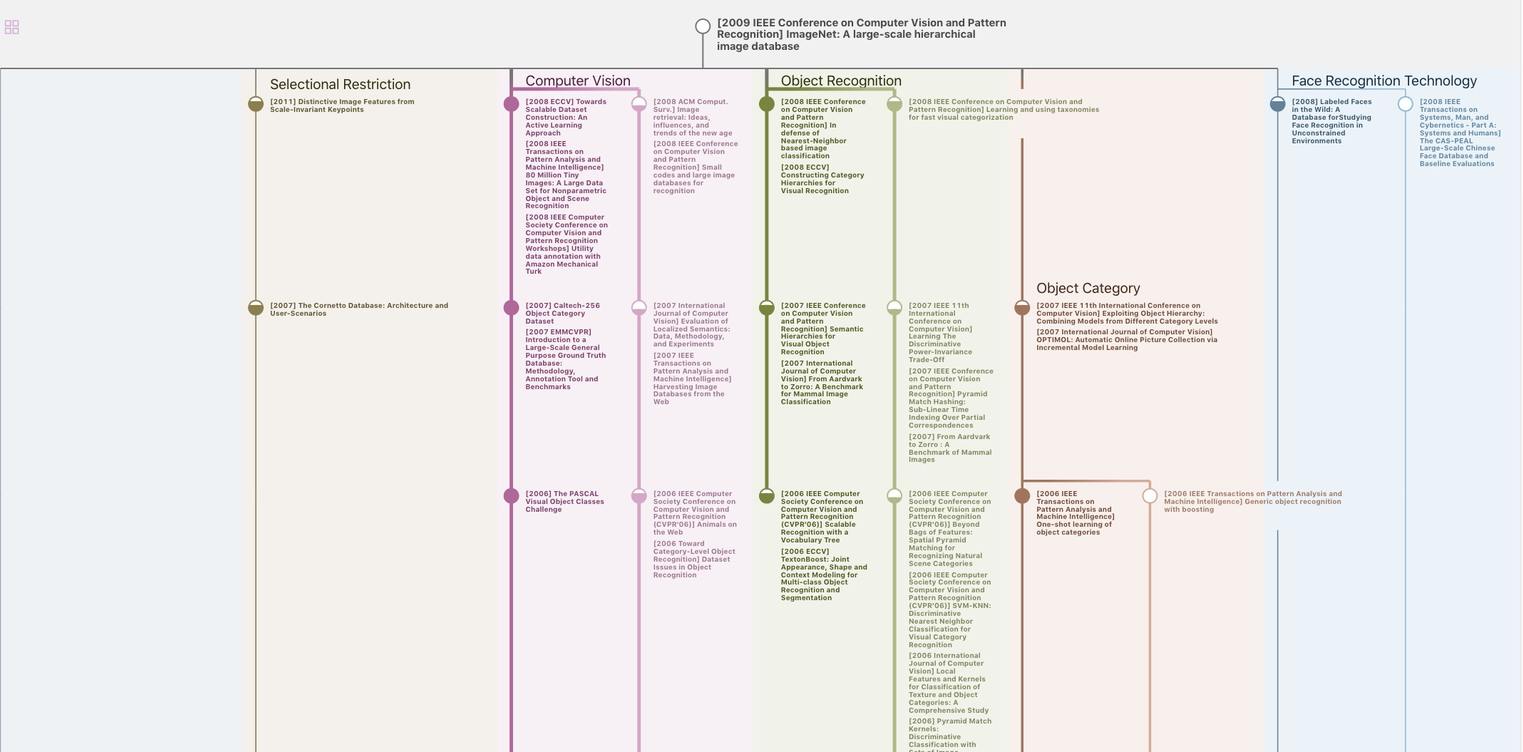
生成溯源树,研究论文发展脉络
Chat Paper
正在生成论文摘要