Routability Optimization for Industrial Designs at Sub-14nm Process Nodes Using Machine Learning.
ISPD(2017)
摘要
Design rule check (DRC) violations after detailed routing prevent a design from being taped out. To solve this problem, state-of-the-art commercial EDA tools global-route the design to produce a global-route congestion map; this map is used by the placer to optimize the placement of the design to reduce detailed-route DRC violations. However, in sub-14nm processes and beyond, DRCs arising from multiple patterning and pin-access constraints drastically weaken the correlation between global-route congestion and detailed-route DRC violations. Hence, the placer|based on the global-route congestion map|may leave too many detailed-route DRC violations to be fixed manually by designers. In this paper, we present a method that employs (1) machine-learning techniques to effectively predict detailed-route DRC violations after global routing and (2) detailed placement techniques to effectively reduce detailed-route DRC violations. We demonstrate on several layouts of a sub-14nm industrial design that this method predicts the locations of 74% of the detailed-route DRCs (with false positive prediction rate below 0.2%) and automatically reduces the number of detailed-route DRC violations by up to 5x. Whereas previous works on machine learning for routability [30] [4] have focused on routability prediction at the floorplanning and placement stages, ours is the first paper that not only predicts the actual locations of detailed-route DRC violations but furthermore optimizes the design to significantly reduce such violations.
更多查看译文
AI 理解论文
溯源树
样例
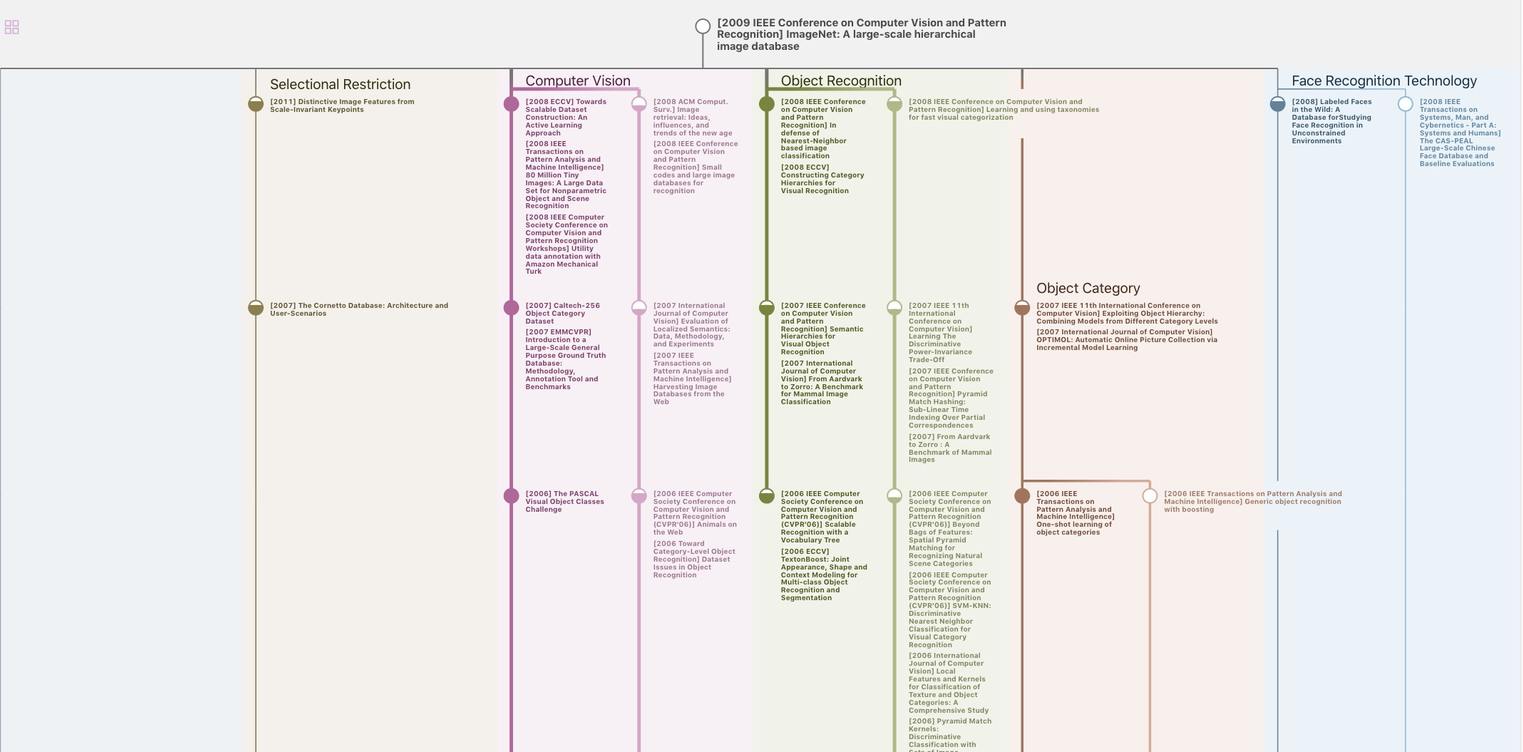
生成溯源树,研究论文发展脉络
Chat Paper
正在生成论文摘要