Online evaluation of bid prediction models in a large-scale computational advertising platform: decision making and insights
Knowl. Inf. Syst.(2016)
摘要
Online media provides opportunities for marketers through which they can deliver effective brand messages to a wide range of audiences at scale. Advertising technology platforms enable advertisers to reach their target audience by delivering ad impressions to online users in real time. In order to identify the best marketing message for a user and to purchase impressions at the right price, we rely heavily on bid prediction and optimization models. Even though the bid prediction models are well studied in the literature, the equally important subject of model evaluation is usually overlooked or not discussed in detail. Effective and reliable evaluation of an online bidding model is crucial for making faster model improvements as well as for utilizing the marketing budgets more efficiently. In this paper, we present an experimentation framework for bid prediction models where our focus is on the practical aspects of model evaluation. Specifically, we outline the unique challenges we encounter in our platform due to a variety of factors such as heterogeneous goal definitions, varying budget requirements across different campaigns, high seasonality and the auction-based environment for inventory purchasing. Then, we introduce return on investment as a unified model performance (i.e., success) metric and explain its merits over more traditional metrics such as click-through rate or conversion rate. Most importantly, we discuss commonly used evaluation and metric summarization approaches in detail and propose a more accurate method for online evaluation of new experimental models against the baseline. Our meta-analysis -based approach addresses various shortcomings of other methods and yields statistically robust conclusions that allow us to conclude experiments more quickly in a reliable manner. We demonstrate the effectiveness of our evaluation strategy on real campaign data through some experiments.
更多查看译文
关键词
A/B test,Experimentation framework,Meta-analysis,Real-time bidding,Computational advertising
AI 理解论文
溯源树
样例
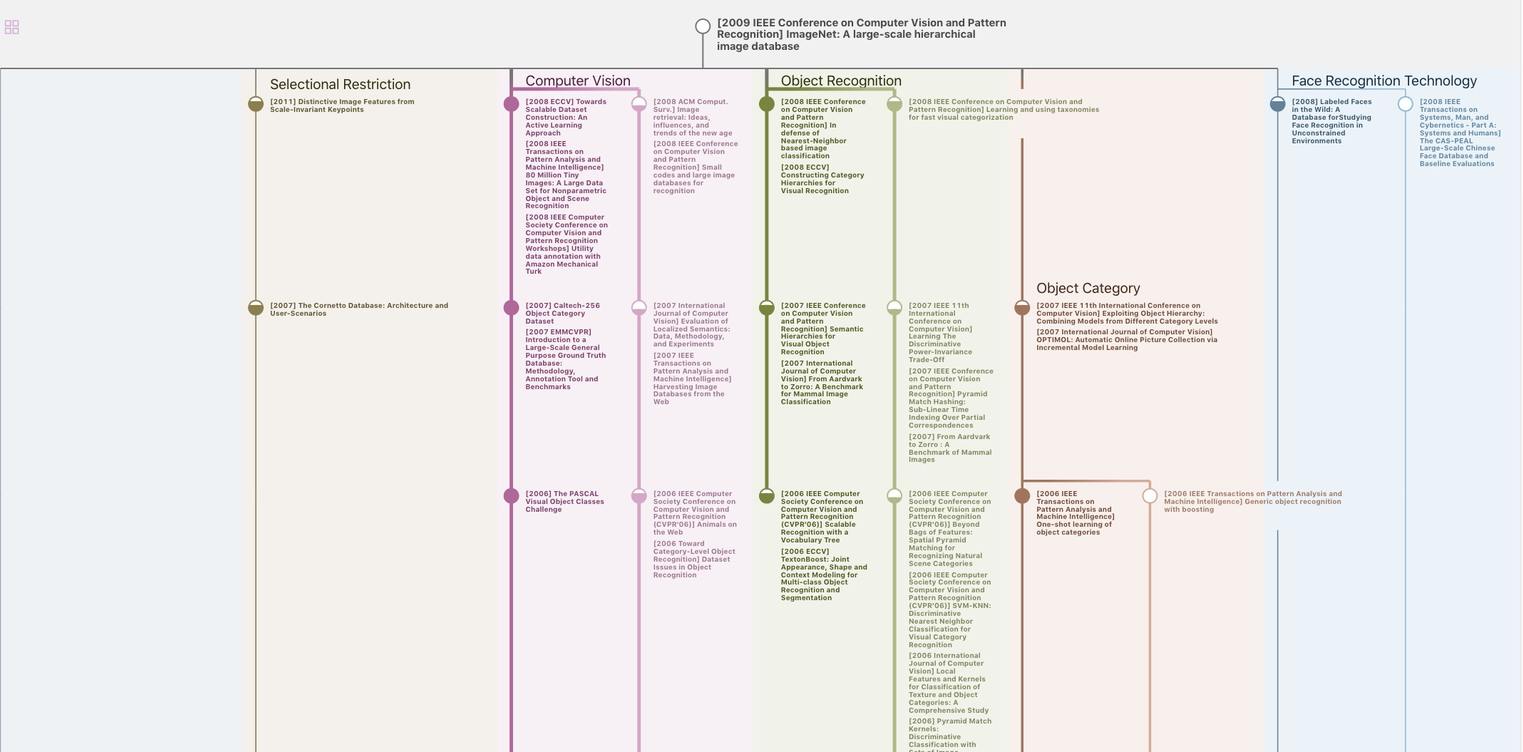
生成溯源树,研究论文发展脉络
Chat Paper
正在生成论文摘要