On the Effect of Scalarising Norm Choice in a ParEGO implementation.
EMO(2017)
摘要
Computationally expensive simulations play an increasing role in engineering design, but their use in multi-objective optimization is heavily resource constrained. Specialist optimizers, such as ParEGO, exist for this setting, but little knowledge is available to guide their configuration. This paper uses a new implementation of ParEGO to examine three hypotheses relating to a key configuration parameter: choice of scalarising norm. Two hypotheses consider the theoretical trade-off between convergence speed and ability to capture an arbitrary Pareto front geometry. Experiments confirm these hypotheses in the bi-objective setting but the trade-off is largely unseen in many-objective settings. A third hypothesis considers the ability of dynamic norm scheduling schemes to overcome the trade-off. Experiments using a simple scheme offer partial support to the hypothesis in the bi-objective setting but no support in many-objective contexts. Norm scheduling is tentatively recommended for bi-objective problems for which the Pareto front geometry is concave or unknown.
更多查看译文
关键词
Expensive optimization, Surrogate-based optimization, Performance evaluation
AI 理解论文
溯源树
样例
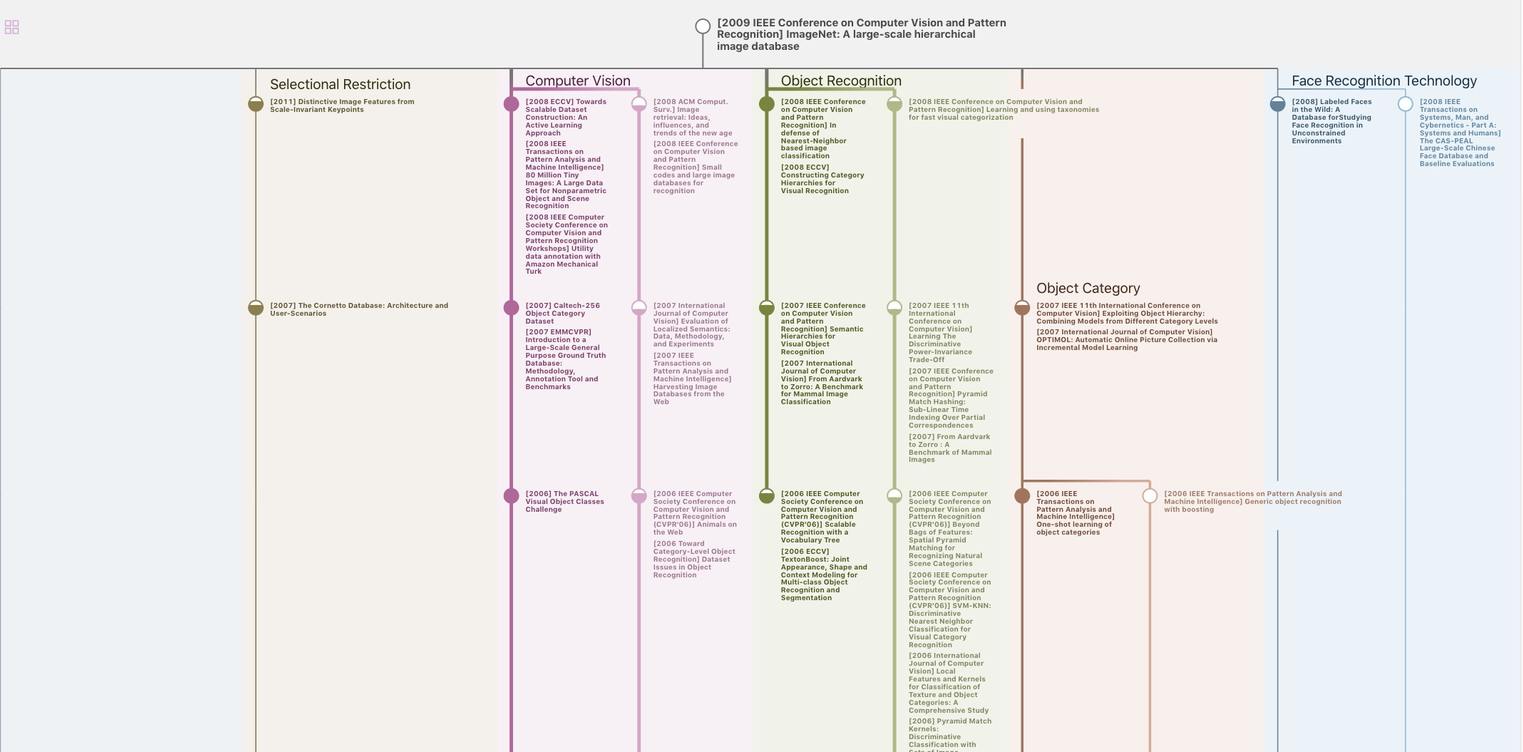
生成溯源树,研究论文发展脉络
Chat Paper
正在生成论文摘要