Classification Of Short Messages Initiated By Mobile Malware
2016 11th International Conference on Availability, Reliability and Security (ARES)(2016)
摘要
In this paper we show that supervised machine learning algorithms can reliably detect short messages initiated by mobile malware based on features derived from the content of short messages. In particular, we compare the detection capabilities of the classifiers Support Vector Machines, K-Nearest Neighbor, Decision Trees, Random Forests, and Multinomial Naive Bayes in three different evaluation scenarios. The first scenario is the standard k-fold cross validation, treating all short messages as independent from each other. In the second scenario, we evaluate, how the classifiers perform if only a certain portion of malware families are known during training. Here, we are able to show that training with only 50% of the the malware families already lead to an accuracy of over 90%. Finally, in the third scenario we evaluate the performance chronologically, i.e. the classifiers are trained with the short messages available at a certain point in time and tested on the newly arriving messages. Here, we show that classifiers can detect the majority of new short messages initiated by mobile malware even months after the training.
更多查看译文
关键词
machine learning,short messages,detection,mobile malware
AI 理解论文
溯源树
样例
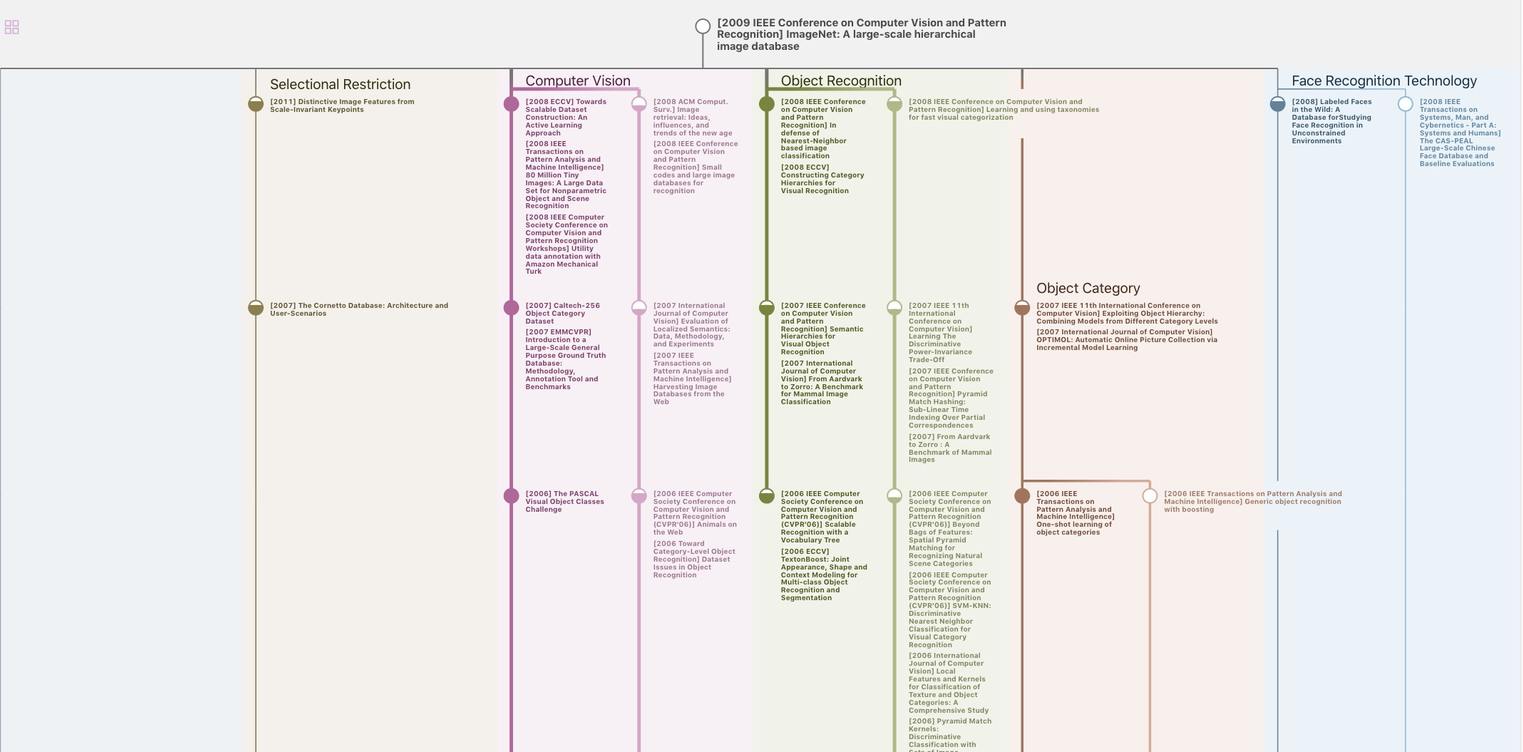
生成溯源树,研究论文发展脉络
Chat Paper
正在生成论文摘要