Coordinating Multiple Disparity Proposals For Stereo Computation
2016 IEEE Conference on Computer Vision and Pattern Recognition (CVPR)(2016)
摘要
While great progress has been made in stereo computation over the last decades, large textureless regions remain challenging. Segment-based methods can tackle this problem properly, but their performances are sensitive to the segmentation results. In this paper, we alleviate the sensitivity by generating multiple proposals on absolute and relative disparities from multi-segmentations. These proposals supply rich descriptions of surface structures. Especially, the relative disparity between distant pixels can encode the large structure, which is critical to handle the large textureless regions. The proposals are coordinated by point-wise competition and pairwise collaboration within a MRF model. During inference, a dynamic programming is performed in different directions with various step sizes, so the long-range connections are better preserved. In the experiments, we carefully analyzed the effectiveness of the major components. Results on the 2014 Middlebury and KITTI 2015 stereo benchmark show that our method is comparable to state-of-the-art.
更多查看译文
关键词
disparity proposals,stereo computation,textureless regions,segment-based methods,multisegmentations,surface structures,relative disparity,point-wise competition,pairwise collaboration,MRF model,dynamic programming,Markov random field
AI 理解论文
溯源树
样例
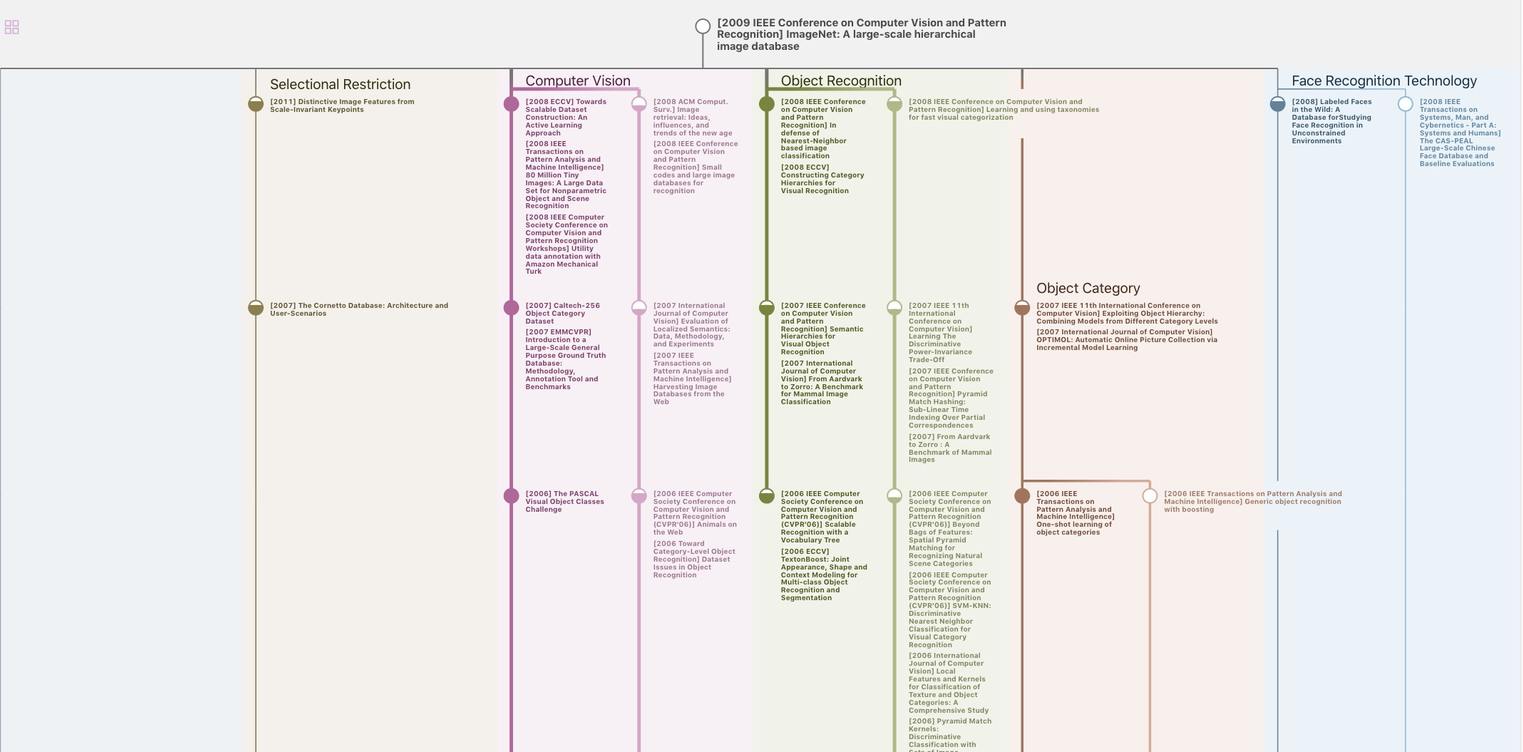
生成溯源树,研究论文发展脉络
Chat Paper
正在生成论文摘要