A Proactive Workflow Model for Healthcare Operation and Management.
IEEE Trans. Knowl. Data Eng.(2017)
摘要
Advances in real-time location systems have enabled us to collect massive amounts of fine-grained semantically rich location traces, which provide unparalleled opportunities for understanding human activities and generating useful knowledge. This, in turn, delivers intelligence for real-time decision making in various fields, such as workflow management. Indeed, it is a new paradigm to model workflows through knowledge discovery in location traces. To that end, in this paper, we provide a focused study of workflow modeling by integrated analysis of indoor location traces in the hospital environment. In particular, we develop a workflow modeling framework that automatically constructs the workflow states and estimates the parameters describing the workflow transition patterns. More specifically, we propose effective and efficient regularizations for modeling the indoor location traces as stochastic processes. First, to improve the interpretability of the workflow states, we use the geography relationship between the indoor rooms to define a prior of the workflow state distribution. This prior encourages each workflow state to be a contiguous region in the building. Second, to further improve the modeling performance, we show how to use the correlation between related types of medical devices to reinforce the parameter estimation for multiple workflow models. In comparison with our preliminary work [11] , we not only develop an integrated workflow modeling framework applicable to general indoor environments, but also improve the modeling accuracy significantly. We reduce the average log-loss by up to 11 percent.
更多查看译文
关键词
Hidden Markov models,Hospitals,Real-time systems,Analytical models,Data models,Buildings
AI 理解论文
溯源树
样例
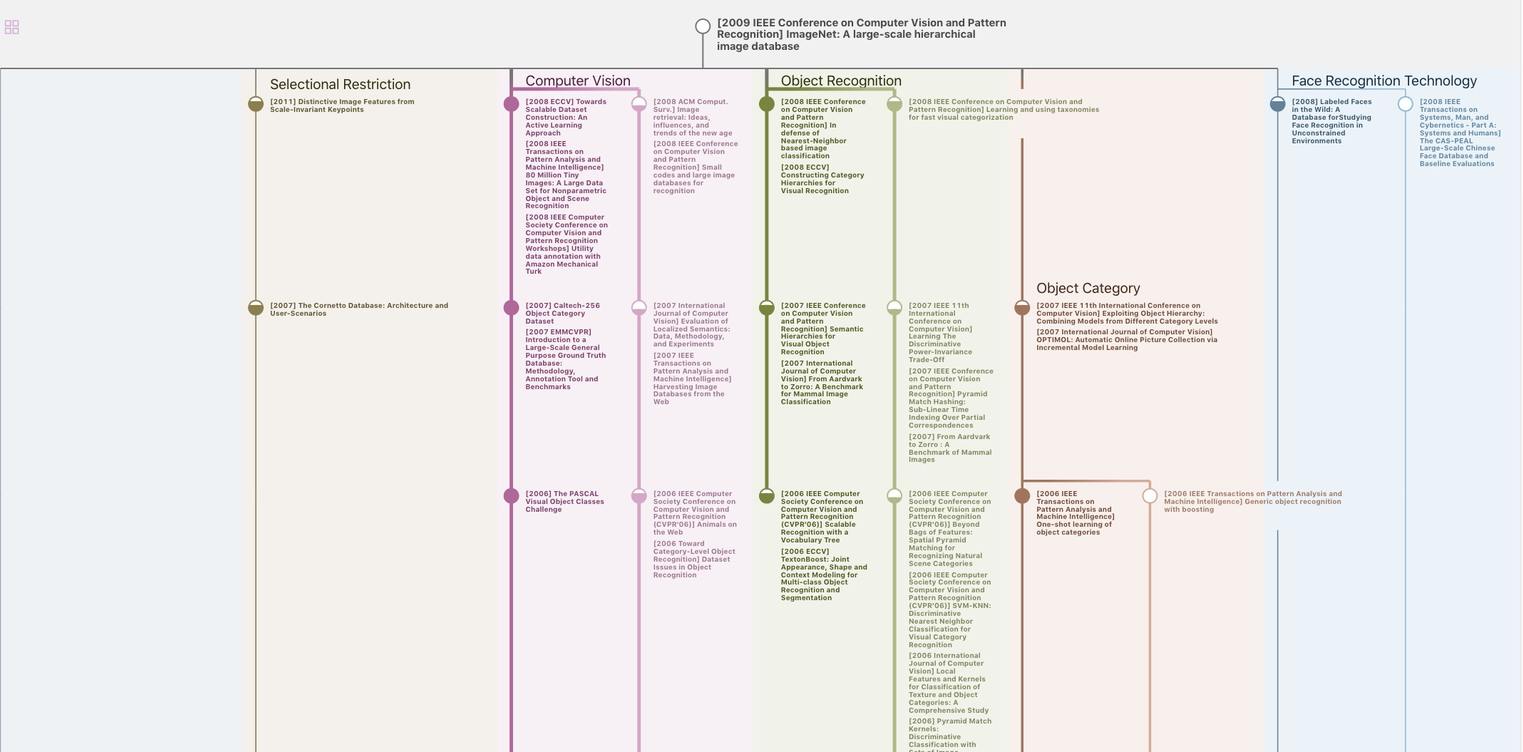
生成溯源树,研究论文发展脉络
Chat Paper
正在生成论文摘要