An Effective Pso-Tlbo Algorithm For Multi-Objective Optimization
2016 IEEE Congress on Evolutionary Computation (CEC)(2016)
摘要
In this study, we present an effective hybrid algorithm based on particle swarm optimization (PSO) and teaching-learning-based optimization (TLBO), called PSO-TLBO, to solve multi-objective optimization (MOO) problems. In PSO-TLBO, teacher phase and learner phase in TLBO are incorporated to a PSO to improve the exploration and exploitation ability. Another feature of PSO-TLBO is the improved circular crowed sorting (CCS) to truncate the external archive which stores the nondominated solutions found during the search. Moreover, the polynomial mutation is used only when the velocities of all solutions are very small. The performance of PSO-TLBO algorithm for MOO is tested on ZDT and DTLZ benchmarks functions and compared with some powerful MOO algorithms in terms of both convergence and spread performance. Also, the contribution of CCS is compared with crowded sorting in NSGA-II. Comparison results reveal the powerful ability of PSO-TLBO to obtain remarkable solutions in terms of inverted generational distance (IGD) metrics.
更多查看译文
关键词
Multi-objective optimization,particle swarm optimization,teaching-learning-based optimization
AI 理解论文
溯源树
样例
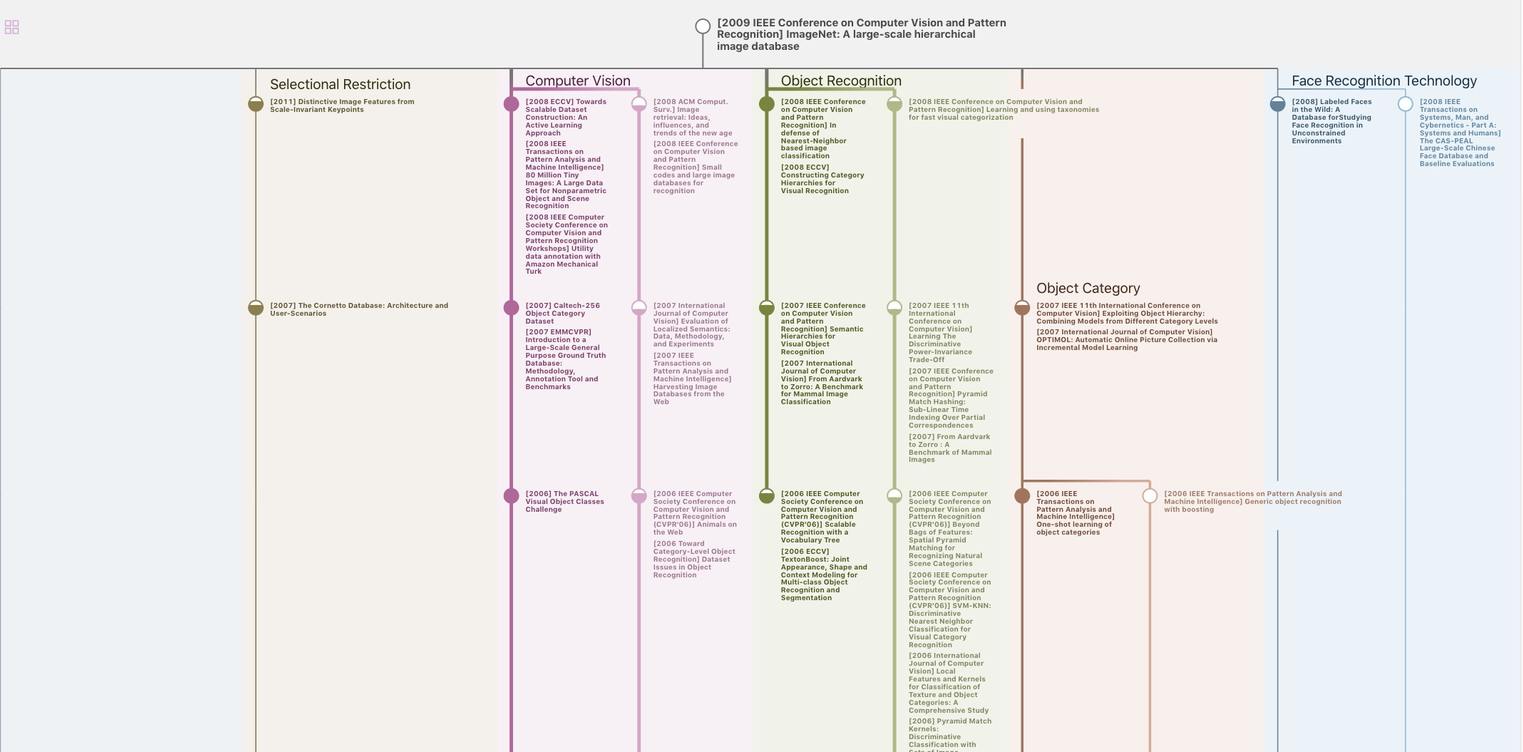
生成溯源树,研究论文发展脉络
Chat Paper
正在生成论文摘要