EEG-based driver fatigue detection using hybrid deep generic model.
2016 38th Annual International Conference of the IEEE Engineering in Medicine and Biology Society (EMBC)(2016)
摘要
Classification of electroencephalography (EEG)-based application is one of the important process for biomedical engineering. Driver fatigue is a major case of traffic accidents worldwide and considered as a significant problem in recent decades. In this paper, a hybrid deep generic model (DGM)-based support vector machine is proposed for accurate detection of driver fatigue. Traditionally, a probabilistic DGM with deep architecture is quite good at learning invariant features, but it is not always optimal for classification due to its trainable parameters are in the middle layer. Alternatively, Support Vector Machine (SVM) itself is unable to learn complicated invariance, but produces good decision surface when applied to well-behaved features. Consolidating unsupervised high-level feature extraction techniques, DGM and SVM classification makes the integrated framework stronger and enhance mutually in feature extraction and classification. The experimental results showed that the proposed DBN-based driver fatigue monitoring system achieves better testing accuracy of 73.29 % with 91.10 % sensitivity and 55.48 % specificity. In short, the proposed hybrid DGM-based SVM is an effective method for the detection of driver fatigue in EEG.
更多查看译文
关键词
Accidents, Traffic,Algorithms,Electroencephalography,Fatigue,Humans,Models, Statistical,Models, Theoretical,Sensitivity and Specificity,Support Vector Machine
AI 理解论文
溯源树
样例
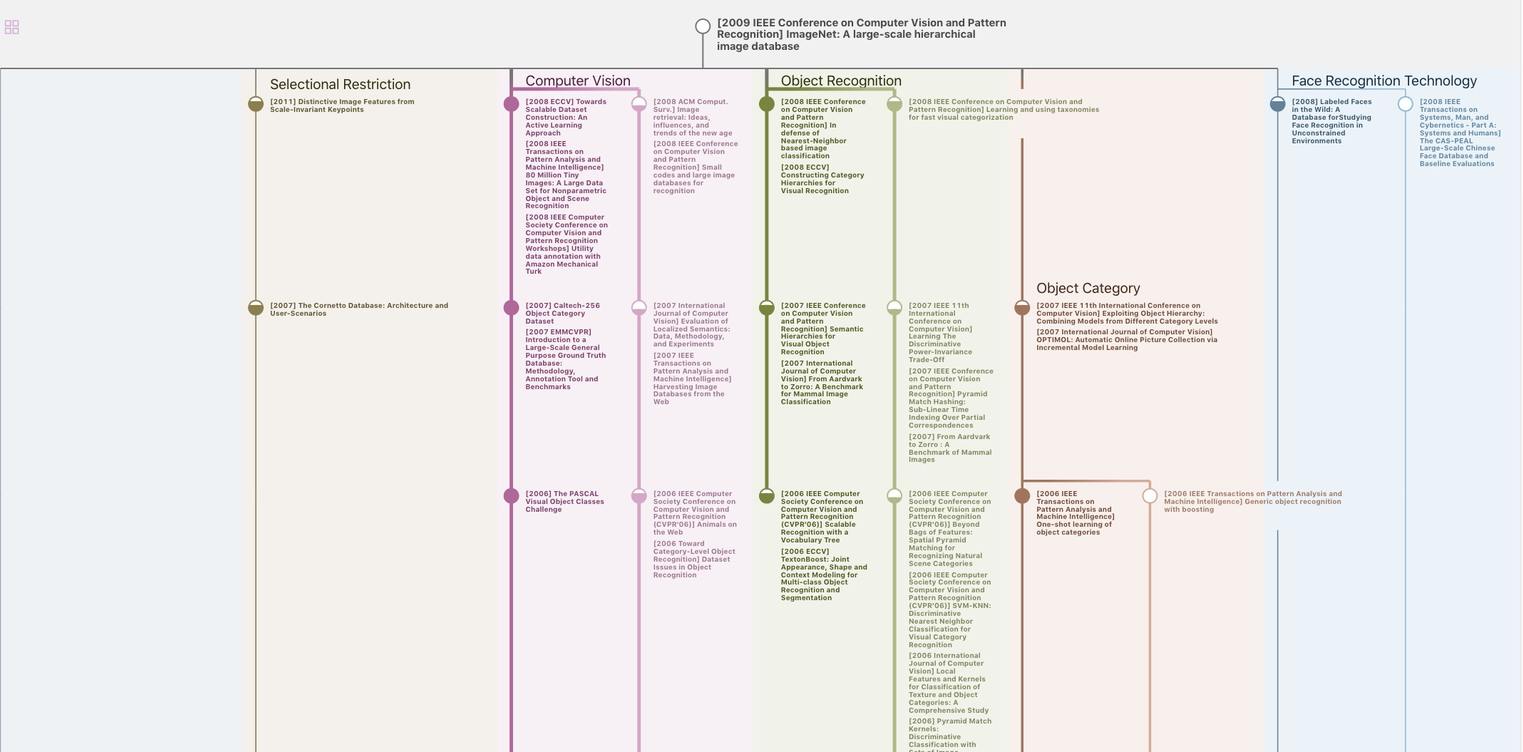
生成溯源树,研究论文发展脉络
Chat Paper
正在生成论文摘要