Revisiting enumerative two-part crude MDL for Bernoulli and multinomial distributions (Extended version).
arXiv: Information Theory(2016)
摘要
We leverage the Minimum Description Length (MDL) principle as a model selection technique for Bernoulli distributions and compare several types of MDL codes. We first present a simplistic crude two-part MDL code and a Normalized Maximum Likelihood (NML) code. We then focus on the enumerative two-part crude MDL code, suggest a Bayesian interpretation for finite size data samples, and exhibit a strong connection with the NML approach. We obtain surprising impacts on the estimation of the model complexity together with superior compression performance. This is then generalized to the case of the multinomial distributions. Both the theoretical analysis and the experimental comparisons suggest that one might use the enumerative code rather than NML in practice, for Bernoulli and multinomial distributions.
更多查看译文
AI 理解论文
溯源树
样例
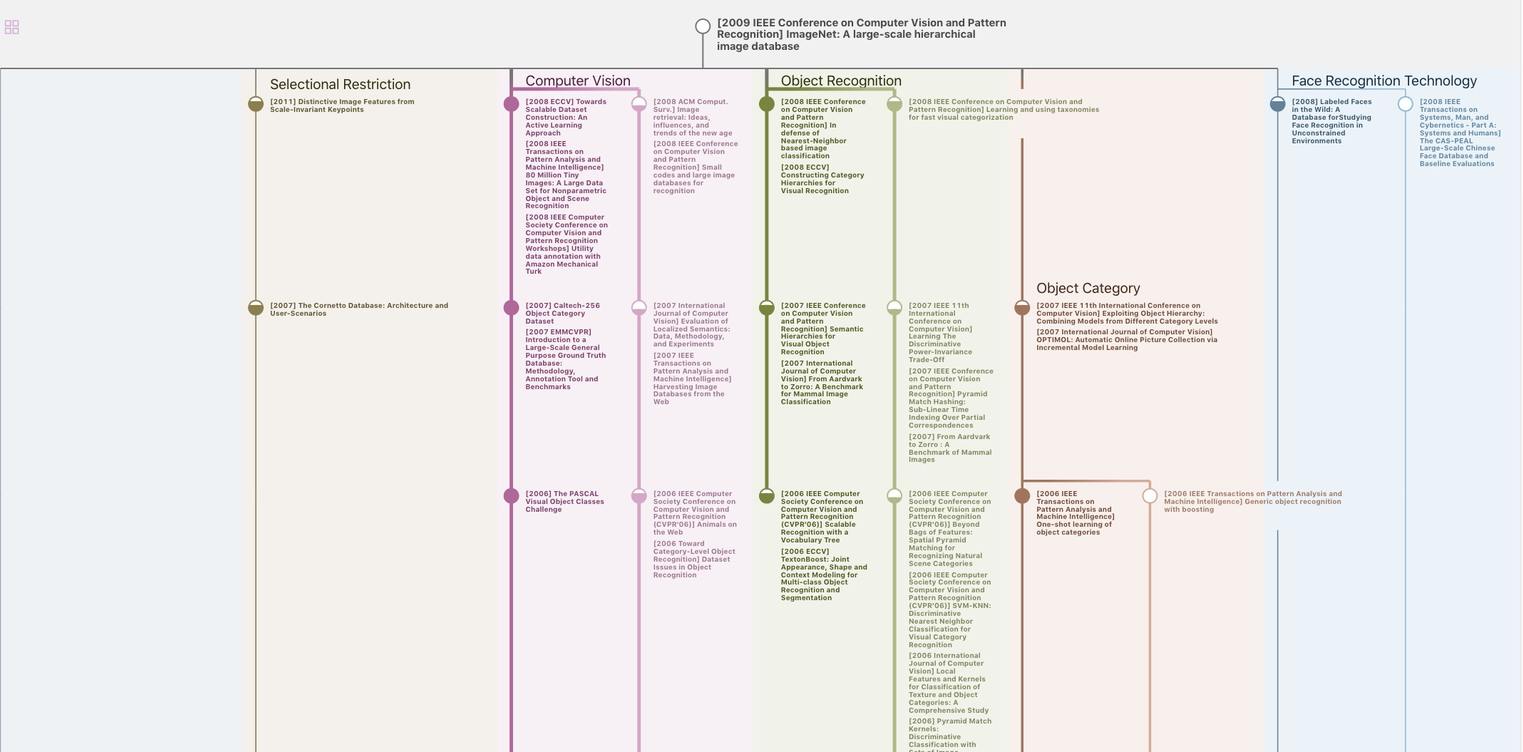
生成溯源树,研究论文发展脉络
Chat Paper
正在生成论文摘要