A novel hybrid teaching learning based multi-objective particle swarm optimization.
Neurocomputing(2017)
摘要
How to obtain a good convergence and well-spread optimal Pareto front is still a major challenge for most meta-heuristic multi-objective optimization (MOO) methods. In this paper, a novel hybrid teaching learning based particle swarm optimization (HTL-PSO) with circular crowded sorting (CCS), named HTL-MOPSO, is proposed for solving MOO problems. Specifically, the new HTL-MOPSO combines the canonical PSO search with a teaching-learning-based optimization (TLBO) algorithm in order to promote the diversity and improve search ability. Also, CCS technique is developed to improve the diversity and spread of solutions when truncating the external elitism archive. The performance of HTL-MOPSO algorithm was tested on several well-known benchmarks problems and compared with other state-of-the-art MOO algorithms in respect of convergence and spread of final solutions to the true Pareto front. Also, the individual contributions made by the strategies of HTL-PSO and CCS are analyzed. Experimental results validate the effectiveness of HTL-MOPSO and demonstrate its superior ability to find solutions of better spread and diversity, while assuring a good convergence.
更多查看译文
关键词
Multi-objective optimization,Particle swarm optimization,Teaching learning based optimization,Crowded sorting
AI 理解论文
溯源树
样例
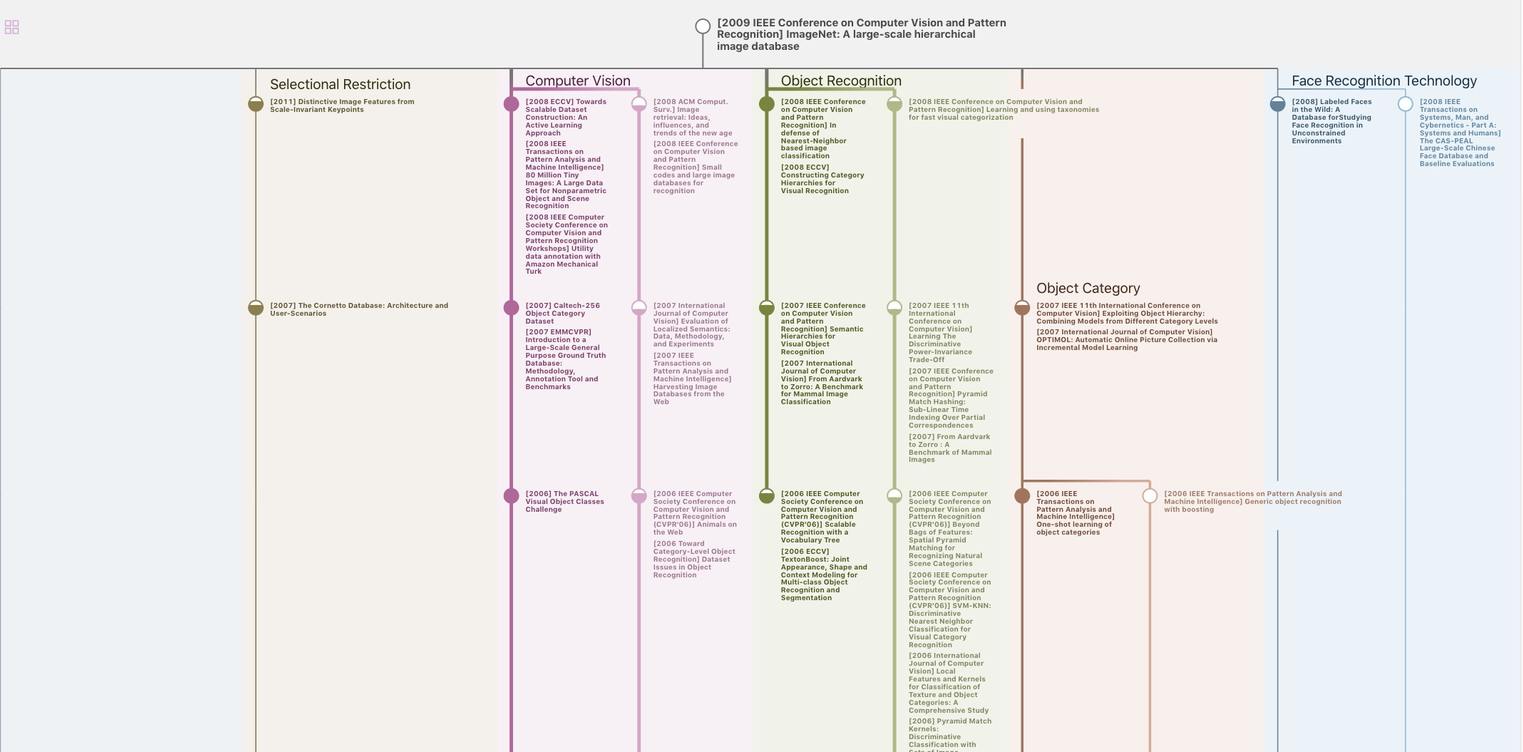
生成溯源树,研究论文发展脉络
Chat Paper
正在生成论文摘要