Crossing The Ks Threshold In The Stochastic Block Model With Information Theory
2016 IEEE INTERNATIONAL SYMPOSIUM ON INFORMATION THEORY(2016)
摘要
Decelle et al. conjectured that community detection in the symmetric stochastic block model has a computational threshold given by the so-called Kesten-Stigum (KS) threshold, and that information-theoretic methods can cross this threshold for a large enough number of communities (4 or 5 depending on the regime of the parameters). This paper shows that at k = 5, it is possible to cross the KS threshold in the disassortative regime with a non-efficient algorithm that samples a clustering having typical cluster volumes. Further, the gap between the KS and information-theoretic threshold is shown to be large in some cases. In the case where edges are drawn only across clusters with an average degree of b, and denoting by k the number of communities, the KS threshold reads b greater than or similar to k(2) whereas our information-theoretic bound reads b greater than or similar to kln(k).
更多查看译文
关键词
Kesten-Stigum threshold,stochastic block model,information theory,community detection,information-theoretic methods
AI 理解论文
溯源树
样例
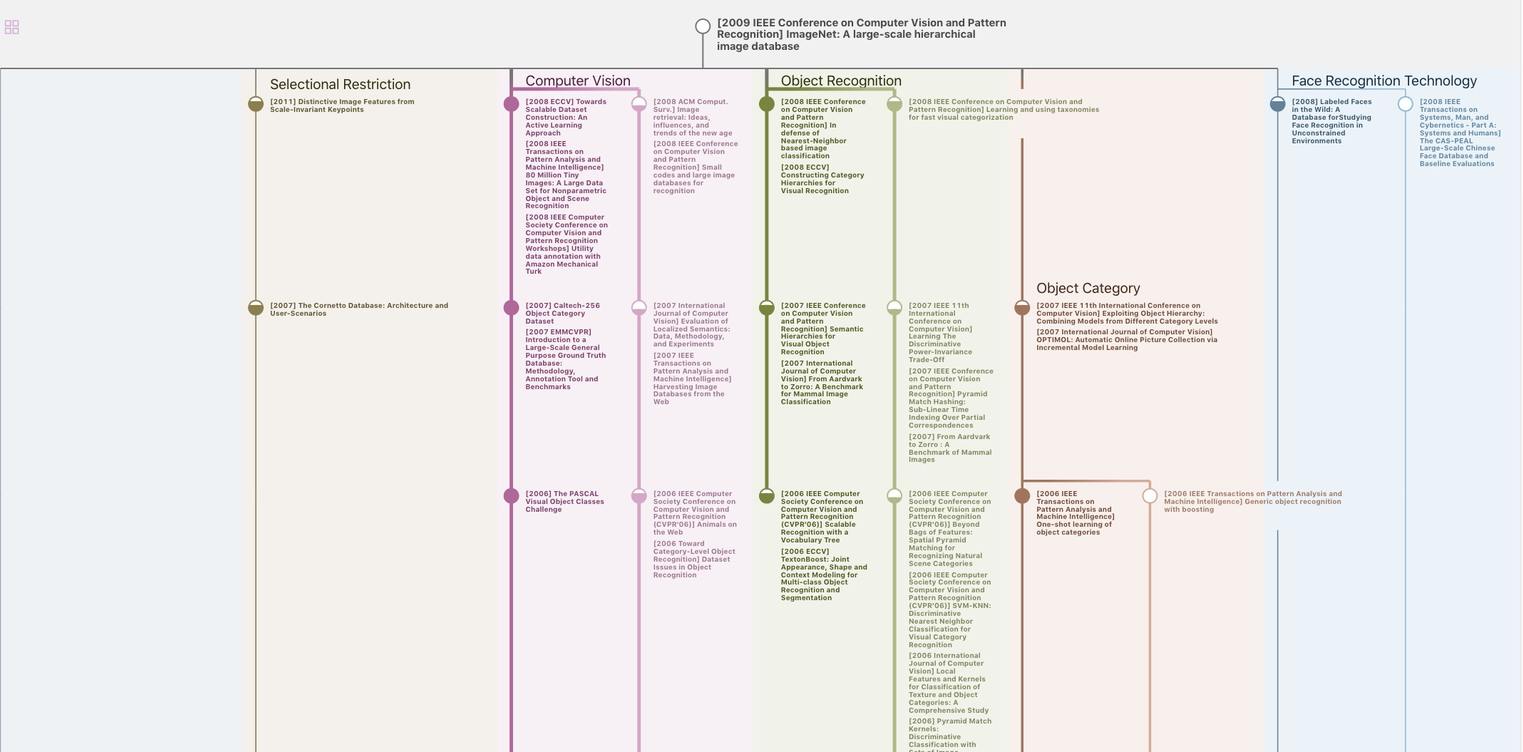
生成溯源树,研究论文发展脉络
Chat Paper
正在生成论文摘要