An Empirical Exploration Of Ctc Acoustic Models
2016 IEEE International Conference on Acoustics, Speech and Signal Processing (ICASSP)(2016)
摘要
The connectionist temporal classification (CTC) loss function has several interesting properties relevant for automatic speech recognition (ASR): applied on top of deep recurrent neural networks (RNNs), CTC learns the alignments between speech frames and label sequences automatically, which removes the need for pre-generated frame-level labels. CTC systems also do not require context decision trees for good performance, using context-independent (CI) phonemes or characters as targets. This paper presents an extensive exploration of CTC-based acoustic models applied to a variety of ASR tasks, including an empirical study of the optimal configuration and architectural variants for CTC. We observe that on large amounts of training data, CTC models tend to outperform state-of-the-art hybrid approach. Further experiments reveal that CTC can be readily ported to syllable-based languages, and can be enhanced by employing improved feature front-ends.
更多查看译文
关键词
CTC,LSTMs,RNNs,acoustic modeling,speech recognition
AI 理解论文
溯源树
样例
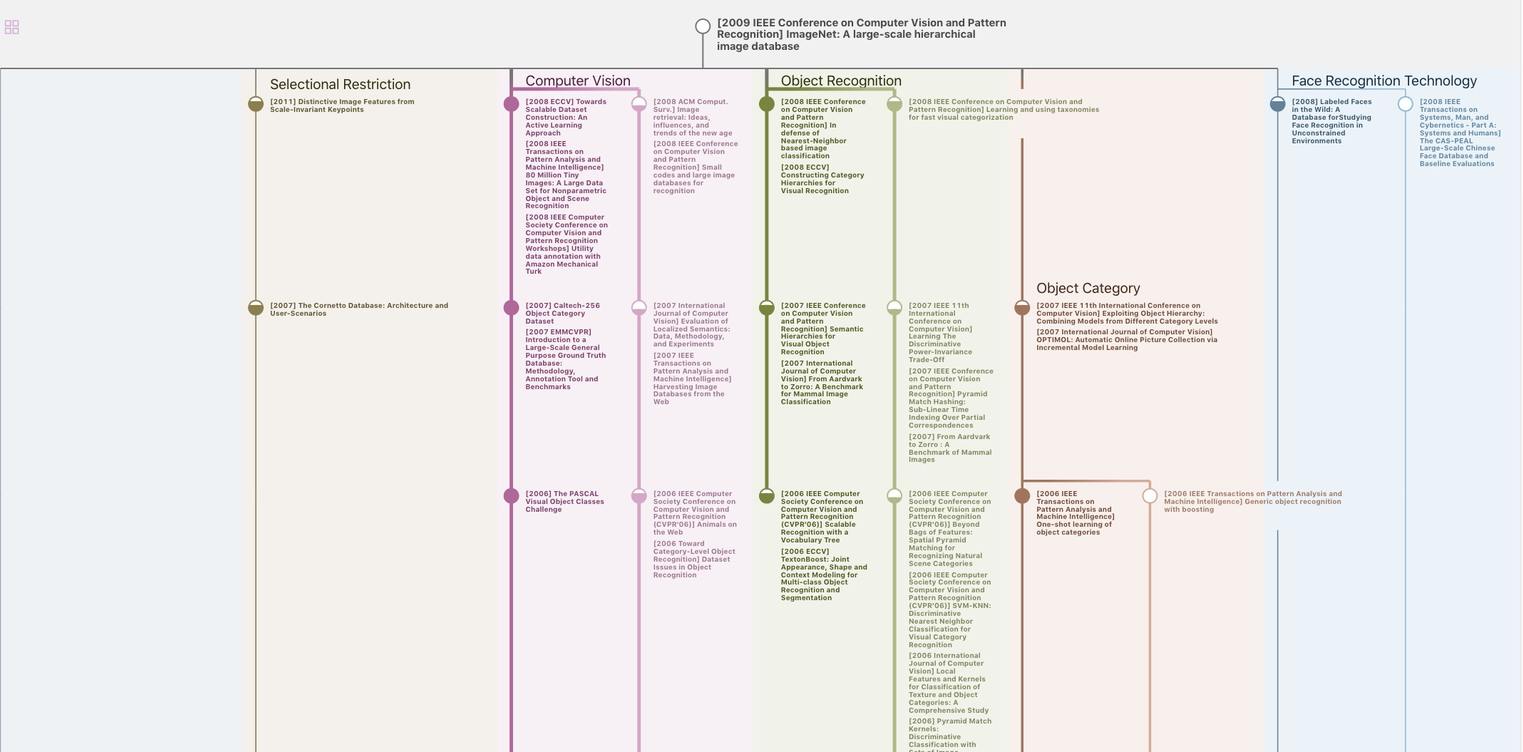
生成溯源树,研究论文发展脉络
Chat Paper
正在生成论文摘要