Estimating topical volume in social media streams.
SAC 2016: Symposium on Applied Computing Pisa Italy April, 2016(2016)
摘要
This paper tackles the problem of estimating the volume of social media posts (e.g., tweets) that pertain to a particular topic. This task differs from related filtering and event detection applications in that the filtered content isn't meant for direct human consumption, but rather we are primarily interested in estimating the cardinality of relevant posts. We present a simple yet effective technique for generating and curating keywords to create what we call "overlap filters", which can be applied to a stream of social media posts. Our approach leverages human labeling and thus a crucial element of the work involves minimizing the cost of human computation. On top of a "day zero" cold start algorithm, we describe a number of optimizations that take advantage of history to further reduce labeling costs. Experimental results show that our overlap filters produce accurate volume estimates at low costs, and our method is simple enough to deploy in practice.
更多查看译文
AI 理解论文
溯源树
样例
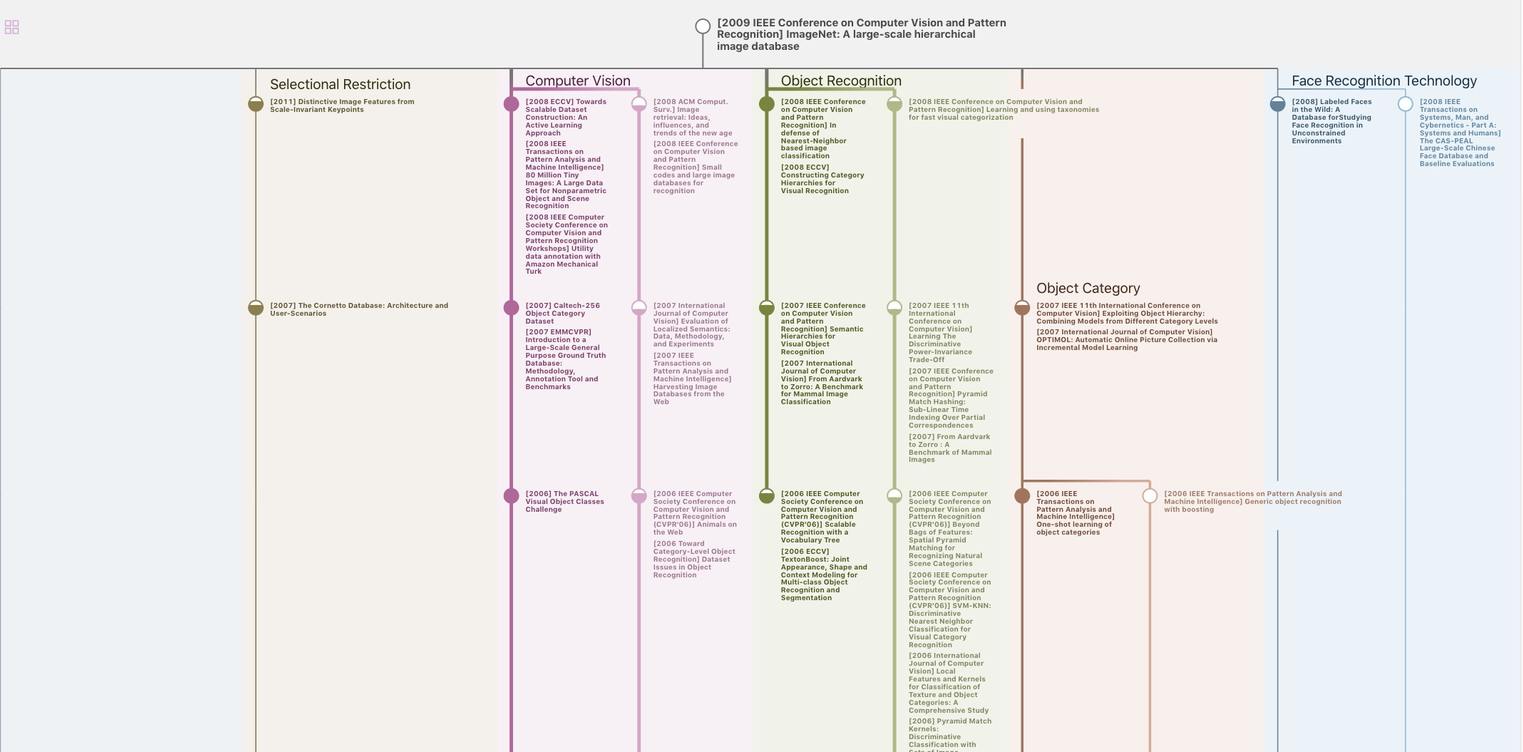
生成溯源树,研究论文发展脉络
Chat Paper
正在生成论文摘要