On Differentially Private Online Collaborative Recommendation Systems
Information Security and Cryptology(2015)
摘要
In collaborative recommendation systems, privacy may be compromised, as users' opinions are used to generate recommendations for others. In this paper, we consider an online collaborative recommendation system, and we measure users' privacy in terms of the standard differential privacy. We give the first quantitative analysis of the trade-offs between recommendation quality and users' privacy in such a system by showing a lower bound on the best achievable privacy for any non-trivial algorithm, and proposing a near-optimal algorithm. From our results, we find that there is actually little trade-off between recommendation quality and privacy for any non-trivial algorithm. Our results also identify the key parameters that determine the best achievable privacy.
更多查看译文
关键词
Differential privacy, Collaborative recommendation system, Lower bound, Online algorithm
AI 理解论文
溯源树
样例
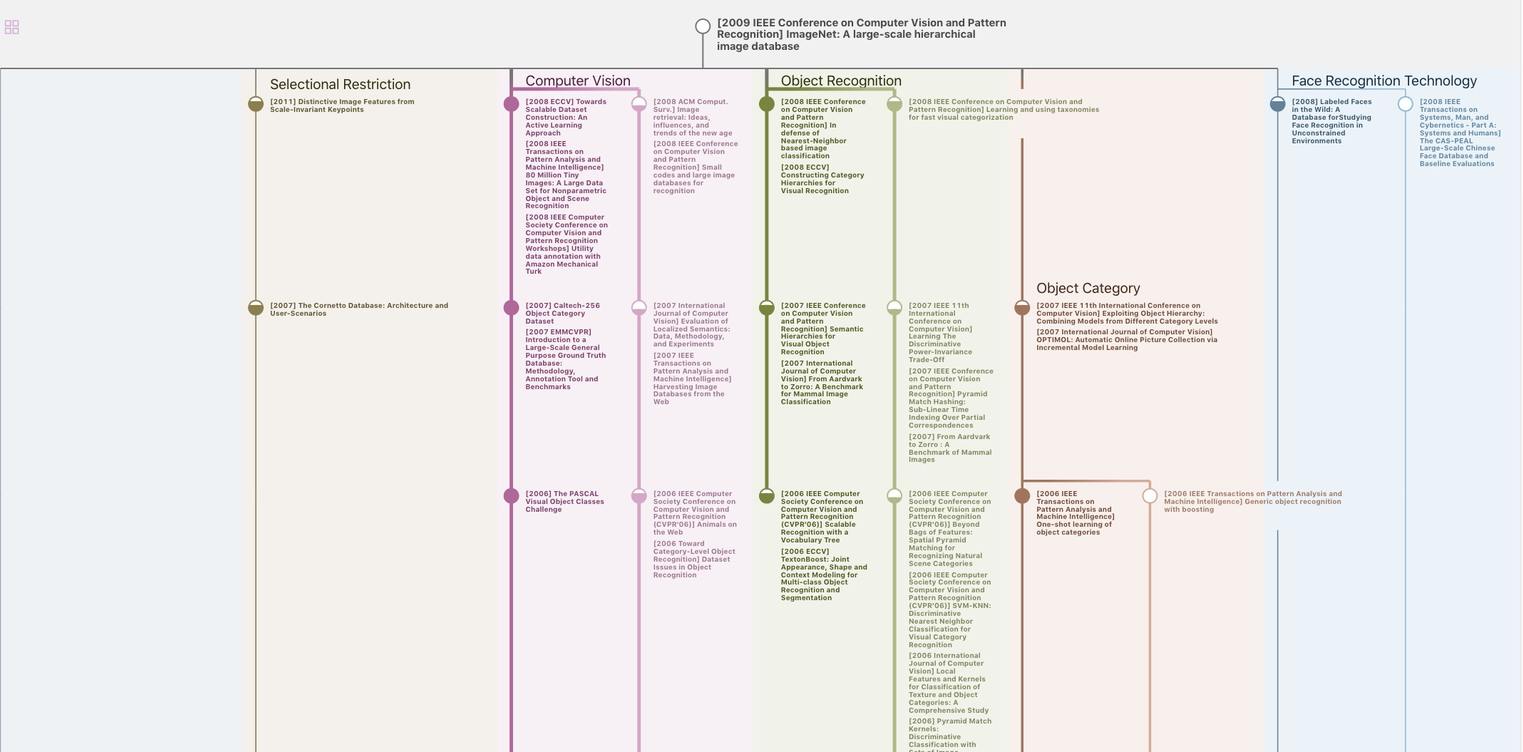
生成溯源树,研究论文发展脉络
Chat Paper
正在生成论文摘要