Building Discriminative User Profiles For Large-Scale Content Recommendation
KDD(2015)
摘要
Content recommendation systems are typically based on one of the following paradigms: user based customization, or recommendations based on either collaborative filtering or low rank matrix factorization methods, or with systems that impute user interest profiles based on content browsing behavior and retrieve items similar to the interest profiles. All of these systems have a distinct disadvantage, namely data sparsity and cold-start on items or users. Furthermore, very few content recommendation solutions explicitly model the wealth of information in implicit negative feedback from the users. In this paper, we propose a hybrid solution that makes use of a latent factor model to infer user interest vectors. The hybrid approach enables us to overcome both the data sparsity and cold-start problems. Our proposed method is learned purely on implicit user feedback, both positive and negative. Exploiting the information in the negative feedback allows the user profiles generated to be discriminative. We also provide a Map/Reduce framework based implementation that enables scaling our solution to real-world recommendation problems. We demonstrate the efficacy of our proposed approach with both offline experiments and A/B tests on live traffic on Yahoo properties.
更多查看译文
关键词
Collaborative filtering,content-based recommendation,large scale recommender systems,latent factor models,user profiling
AI 理解论文
溯源树
样例
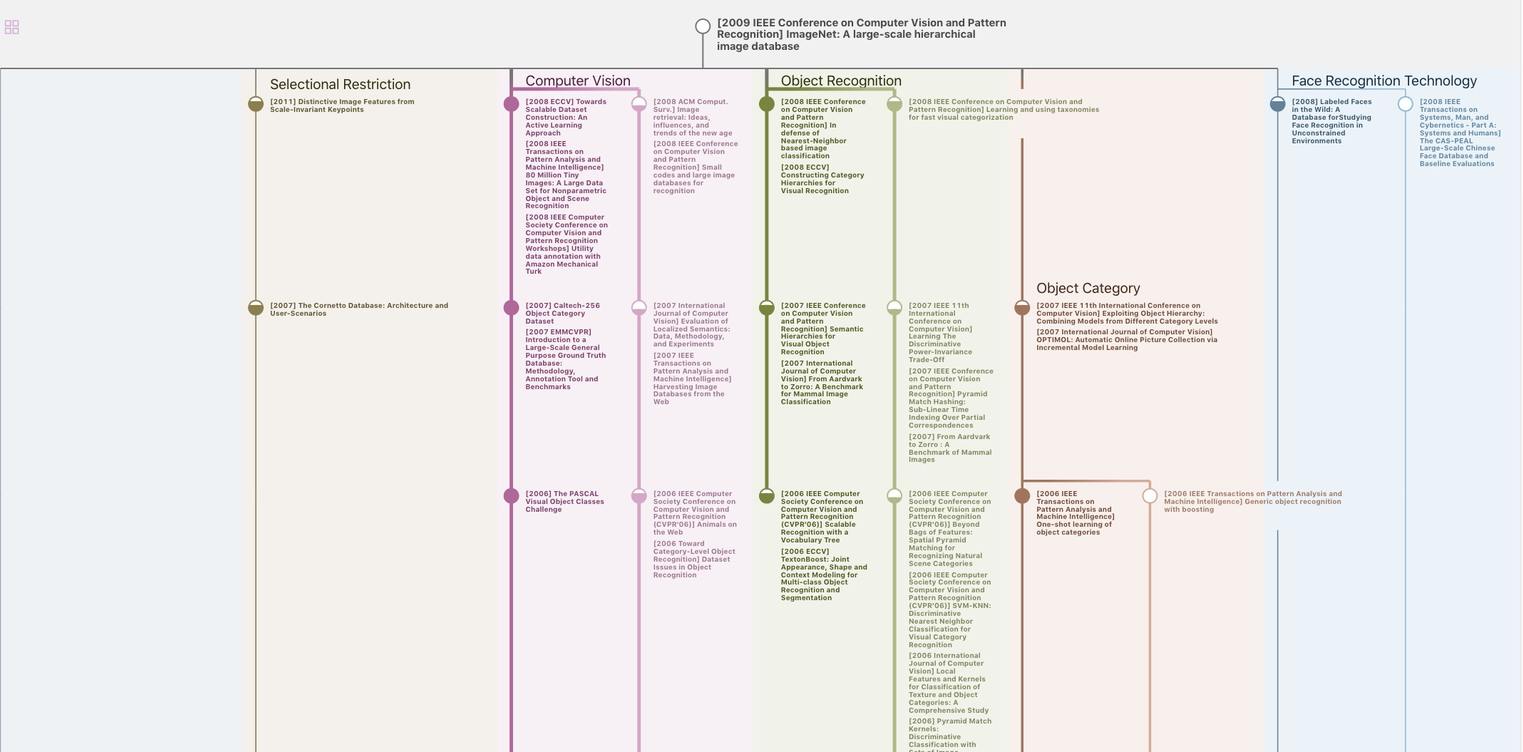
生成溯源树,研究论文发展脉络
Chat Paper
正在生成论文摘要