Aggregating Data Sampling With Feature Subset Selection To Address Skewed Software Defect Data
INTERNATIONAL JOURNAL OF SOFTWARE ENGINEERING AND KNOWLEDGE ENGINEERING(2015)
摘要
Defect prediction is an important process activity frequently used for improving the quality and reliability of software products. Defect prediction results provide a list of fault-prone modules which are necessary in helping project managers better utilize valuable project resources. In the software quality modeling process, high dimensionality and class imbalance are the two potential problems that may exist in data repositories. In this study, we investigate three data preprocessing approaches, in which feature selection is combined with data sampling, to overcome these problems in the context of software quality estimation. These three approaches are: Approach 1 - sampling performed prior to feature selection, but retaining the unsampled data instances; Approach 2 - sampling performed prior to feature selection, retaining the sampled data instances; and Approach 3 - sampling performed after feature selection. A comparative investigation is presented for evaluating the three approaches. In the experiments, we employed three sampling methods (random undersampling, random oversampling, and synthetic minority oversampling), each combined with a filter-based feature subset selection technique called correlation-based feature selection. We built the defect prediction models using five common classification algorithms. The case study was based on software metrics and defect data collected from multiple releases of a real-world software system. The results demonstrated that the type of sampling methods used in data preprocessing significantly affected the performance of the combination approaches. It was found that when the random undersampling technique was used, Approach 1 performed better than the other two approaches. However, when the feature selection technique was used in conjunction with an oversampling method (random oversampling or synthetic minority oversampling), we strongly recommended Approach 3.
更多查看译文
关键词
Feature subset selection, data sampling, high dimensionality, class imbalance, software defect prediction
AI 理解论文
溯源树
样例
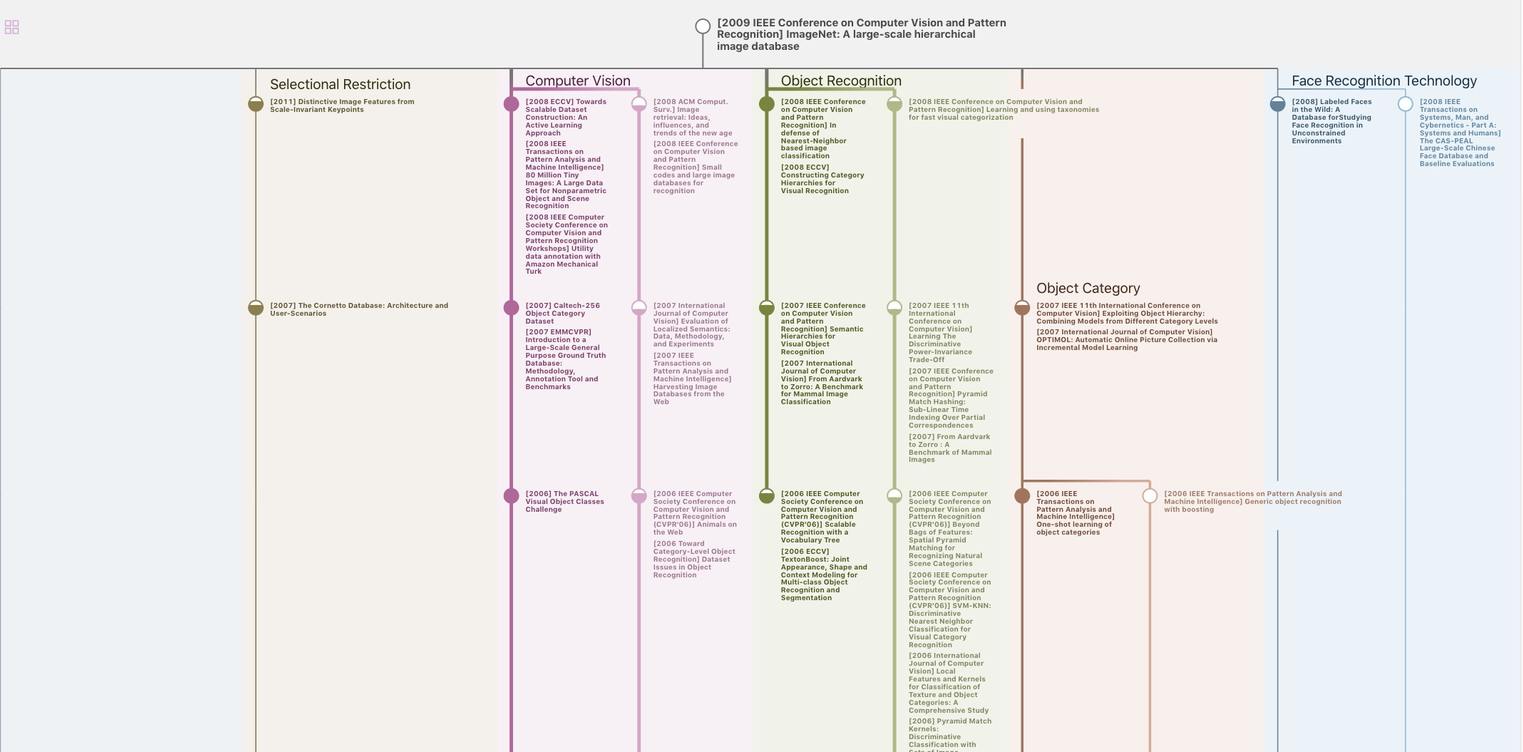
生成溯源树,研究论文发展脉络
Chat Paper
正在生成论文摘要