NED: An Inter-Graph Node Metric Based On Edit Distance.
PROCEEDINGS OF THE VLDB ENDOWMENT(2017)
摘要
Node similarity is fundamental in graph analytics. However, node similarity between nodes in different graphs (inter graph nodes) has not received enough attention yet. The inter-graph node similarity is important in learning a new graph based on the knowledge extracted from an existing graph (transfer learning on graphs) and has applications in biological, communication, and social networks. In this paper, we propose a novel distance function for measuring inter-graph node similarity with edit distance, called NED. In NED, two nodes are compared according to their local neighborhood topologies which are represented as unordered k-adjacent trees, without relying on any extra information. Due to the hardness of computing tree edit distance on unordered trees which is NP-Complete, we propose a modified tree edit distance, called TED*, for comparing unordered and unlabeled k-adjacent trees. TED* is a metric distance, as the original tree edit distance, but more importantly, TED* is polynomially computable. As a metric distance, NED admits efficient indexing, provides interpretable results, and shows to perform better than existing approaches on a number of data analysis tasks, including graph deanonymization. Finally, the efficiency and effectiveness of NED are empirically demonstrated using real-world graphs.
更多查看译文
AI 理解论文
溯源树
样例
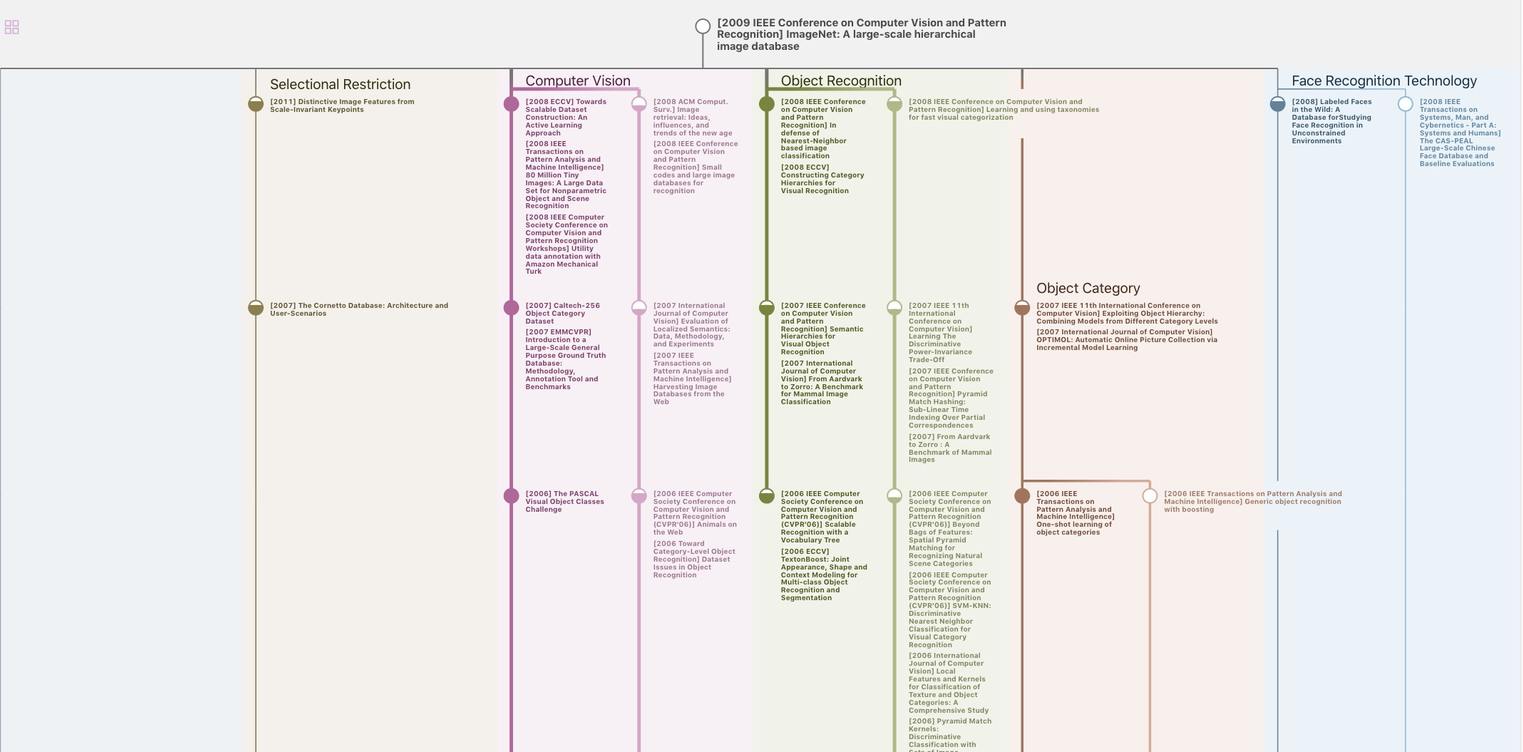
生成溯源树,研究论文发展脉络
Chat Paper
正在生成论文摘要