Algorithms for Batch Hierarchical Reinforcement Learning.
arXiv: Artificial Intelligence(2016)
摘要
Hierarchical Reinforcement Learning (HRL) exploits temporal abstraction to solve large Markov Decision Processes (MDP) and provide transferable subtask policies. In this paper, we introduce an off-policy HRL algorithm: Hierarchical Q-value Iteration (HQI). We show that it is possible to effectively learn recursive optimal policies for any valid hierarchical decomposition of the original MDP, given a fixed dataset collected from a flat stochastic behavioral policy. We first formally prove the convergence of the algorithm for tabular MDP. Then our experiments on the Taxi domain show that HQI converges faster than a flat Q-value Iteration and enjoys easy state abstraction. Also, we demonstrate that our algorithm is able to learn optimal policies for different hierarchical structures from the same fixed dataset, which enables model comparison without recollecting data.
更多查看译文
AI 理解论文
溯源树
样例
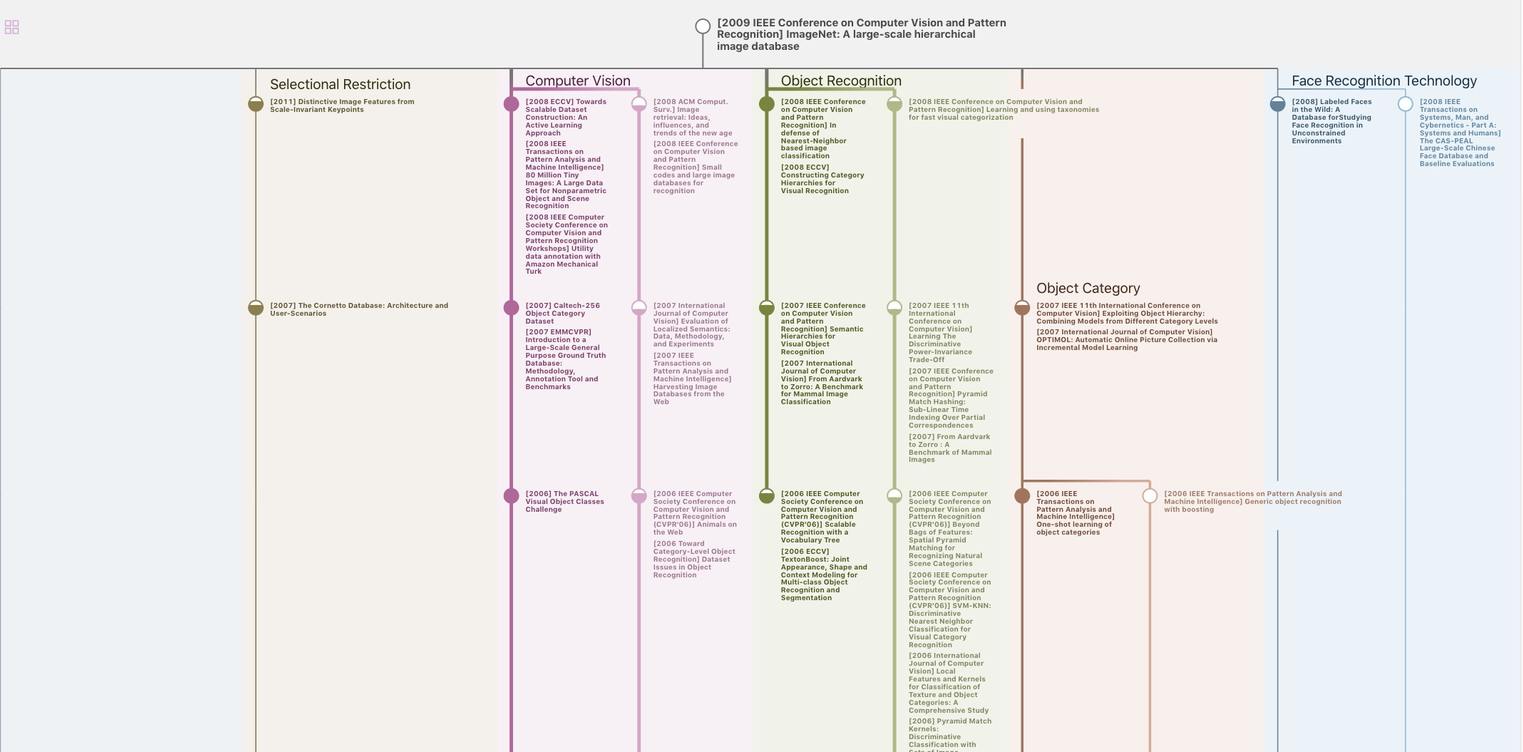
生成溯源树,研究论文发展脉络
Chat Paper
正在生成论文摘要