Modified SCAD penalty for constrained variable selection problems
Statistical Methodology(2014)
摘要
Instead of using sample information only to do variable selection, in this article we also take priori information — linear constraints of regression coefficients — into account. The penalized likelihood estimation method is adopted. However under constraints, it is not guaranteed that information criteria like AIC and BIC are minimized at an oracle solution using the lasso or SCAD penalty. To overcome such difficulties, a modified SCAD penalty is proposed. The definitions of information criteria GCV, AIC and BIC for constrained variable selection problems are also proposed. Statistically, we show that if the tuning parameter is appropriately chosen, the proposed estimators enjoy the oracle properties and satisfy the linear constraints. Additionally, they also possess the robust property to outliers if the linear model with M-estimation is used.
更多查看译文
关键词
M-estimation,Diverging number of parameters,SCAD,GCV,Oracle property,Generalized linear models
AI 理解论文
溯源树
样例
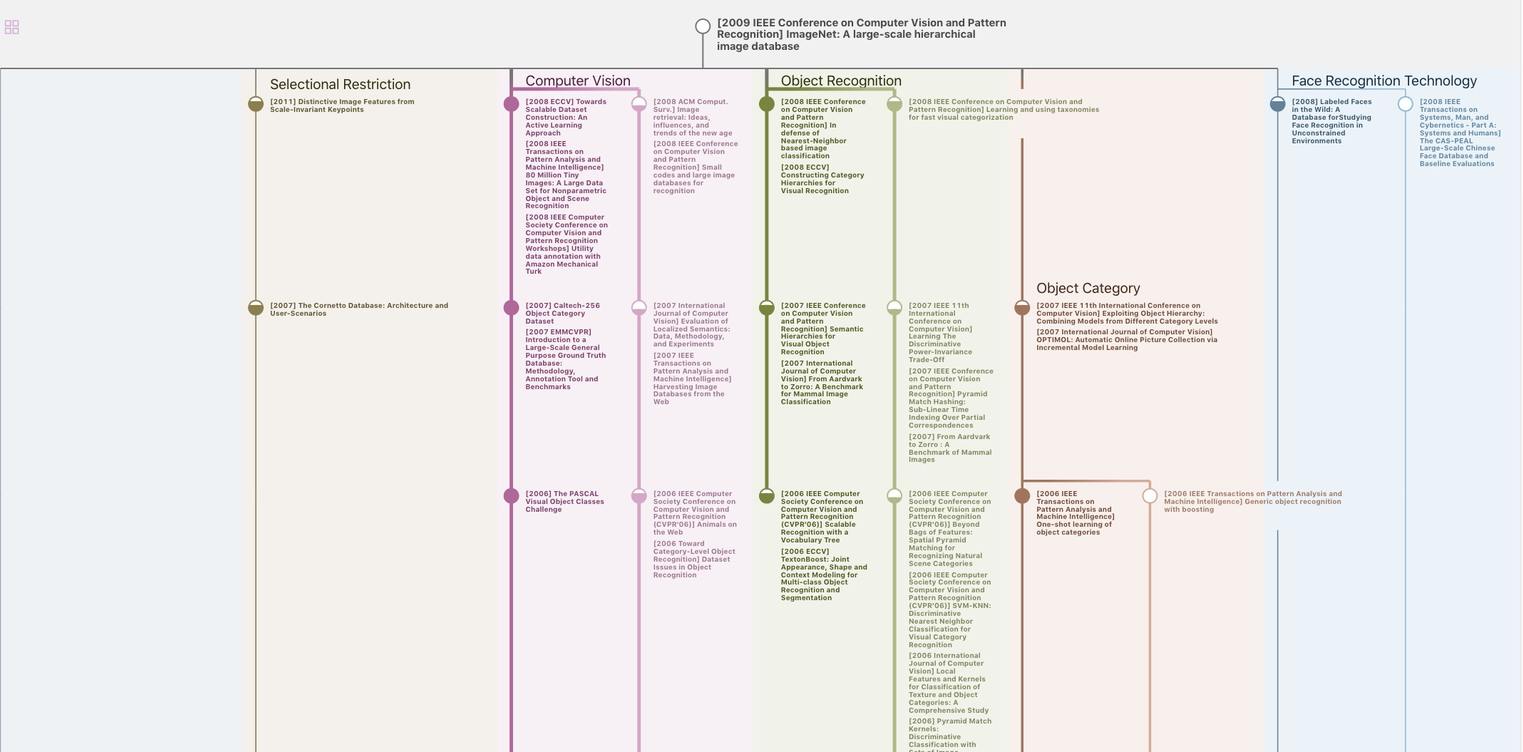
生成溯源树,研究论文发展脉络
Chat Paper
正在生成论文摘要