Simple Semi-Supervised POS Tagging
VS@HLT-NAACL(2015)
摘要
We tackle the question: how much supervision is needed to achieve state-of-the-art performance in part-of-speech (POS) tagging, if we leverage lexical representations given by the model of Brown et al. (1992)? It has become a standard practice to use automatically induced “Brown clusters” in place of POS tags. We claim that the underlying sequence model for these clusters is particularly well-suited for capturing POS tags. We empirically demonstrate this claim by drastically reducing supervision in POS tagging with these representations. Using either the bit-string form given by the algorithm of Brown et al. (1992) or the (less well-known) embedding form given by the canonical correlation analysis algorithm of Stratos et al. (2014), we can obtain 93% tagging accuracy with just 400 labeled words and achieve state-of-the-art accuracy (> 97%) with less than 1 percent of the original training data.
更多查看译文
AI 理解论文
溯源树
样例
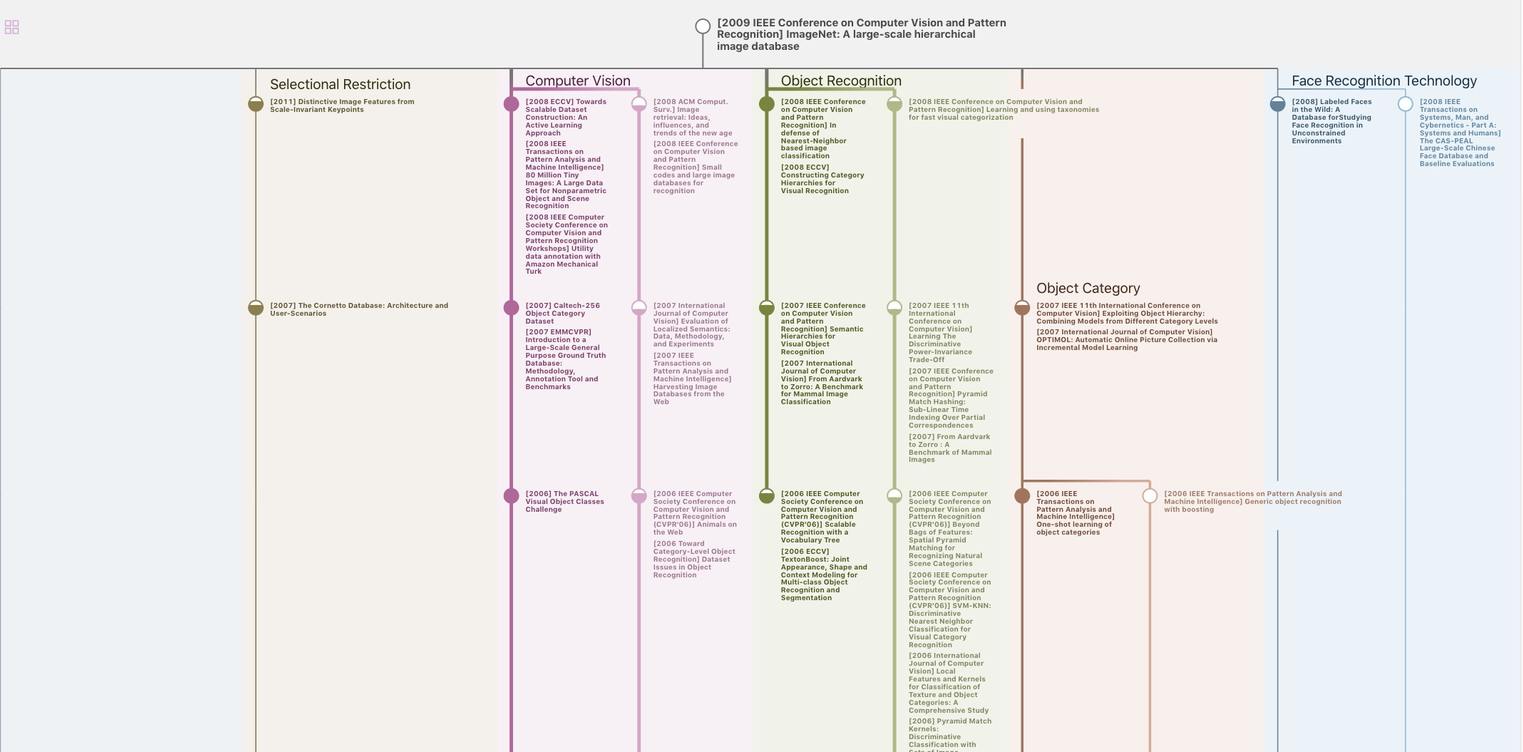
生成溯源树,研究论文发展脉络
Chat Paper
正在生成论文摘要