The ADMM Algorithm for Distributed Quadratic Problems: Parameter Selection and Constraint Preconditioning
IEEE Transactions on Signal Processing(2016)
摘要
This paper presents optimal parameter selection and preconditioning of the alternating direction method of multipliers (ADMM) algorithm for a class of distributed quadratic problems, which can be formulated as equality-constrained quadratic programming problems. The parameter selection focuses on the ADMM step-size and relaxation parameter, while the preconditioning corresponds to selecting the edge weights of the underlying communication graph. We optimize these parameters to yield the smallest convergence factor of the iterates. Explicit expressions are derived for the step-size and relaxation parameter, as well as for the corresponding convergence factor. Numerical simulations justify our results and highlight the benefits of optimal parameter selection and preconditioning for the ADMM algorithm.
更多查看译文
关键词
Distributed optimization,convergence rate,optimal step-size
AI 理解论文
溯源树
样例
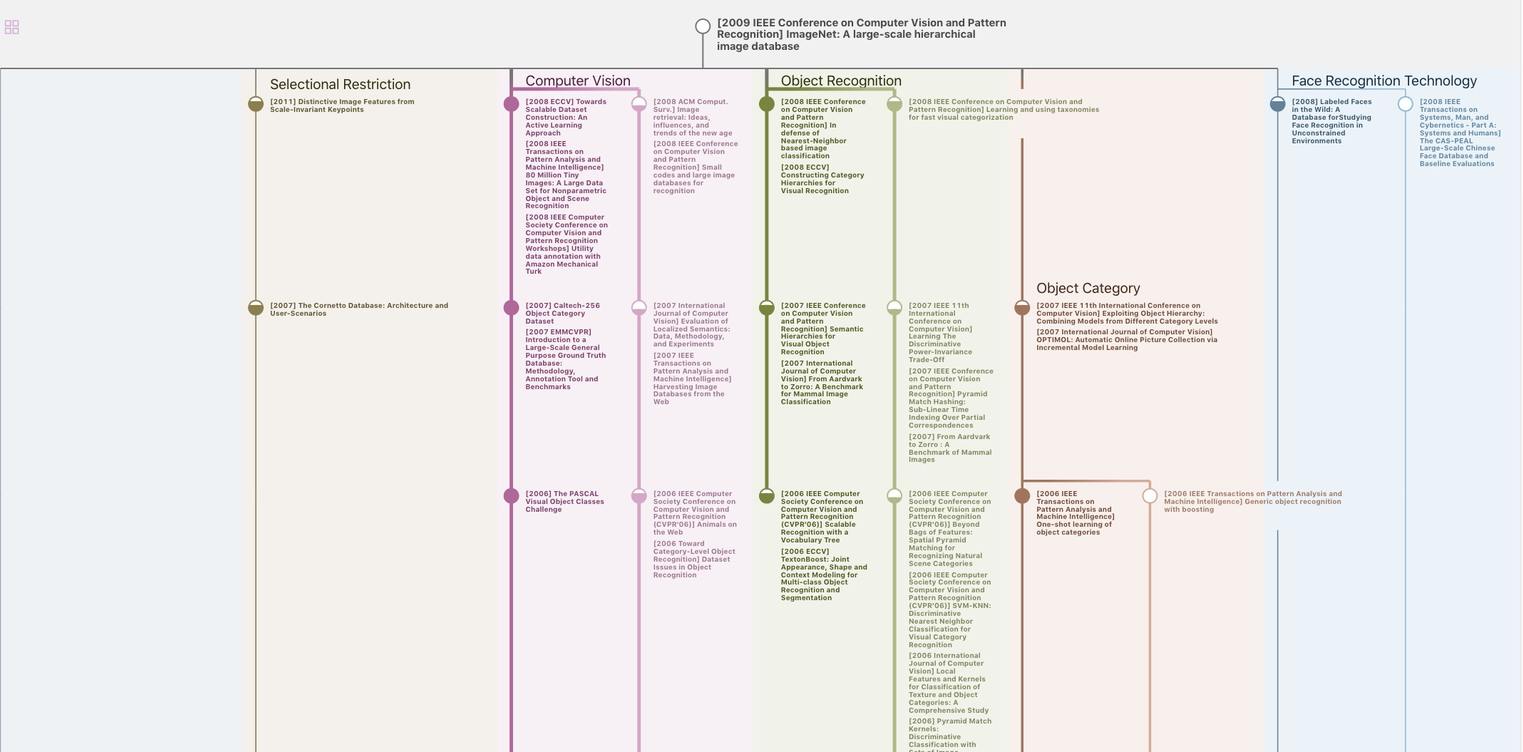
生成溯源树,研究论文发展脉络
Chat Paper
正在生成论文摘要