Asymmetries in predictive and diagnostic reasoning.
JOURNAL OF EXPERIMENTAL PSYCHOLOGY-GENERAL(2011)
摘要
In this article, we address the apparent discrepancy between causal Bayes net theories of cognition, which posit that judgments of uncertainty are generated from causal beliefs in a way that respects the norms of probability, and evidence that probability judgments based on causal beliefs are systematically in error. One purported source of bias is the ease of reasoning forward from cause to effect (predictive reasoning) versus backward from effect to cause (diagnostic reasoning). Using causal Bayes nets, we developed a normative formulation of how predictive and diagnostic probability judgments should vary with the strength of alternative causes, causal power, and prior probability. This model was tested through two experiments that elicited predictive and diagnostic judgments as well as judgments of the causal parameters for a variety of scenarios that were designed to differ in strength of alternatives. Model predictions fit the diagnostic judgments closely, but predictive judgments displayed systematic neglect of alternative causes, yielding a relatively poor fit. Three additional experiments provided more evidence of the neglect of alternative causes in predictive reasoning and ruled out pragmatic explanations. We conclude that people use causal structure to generate probability judgments in a sophisticated but not entirely veridical way.
更多查看译文
关键词
judgment,causal reasoning,prediction,diagnostic reasoning,causal models
AI 理解论文
溯源树
样例
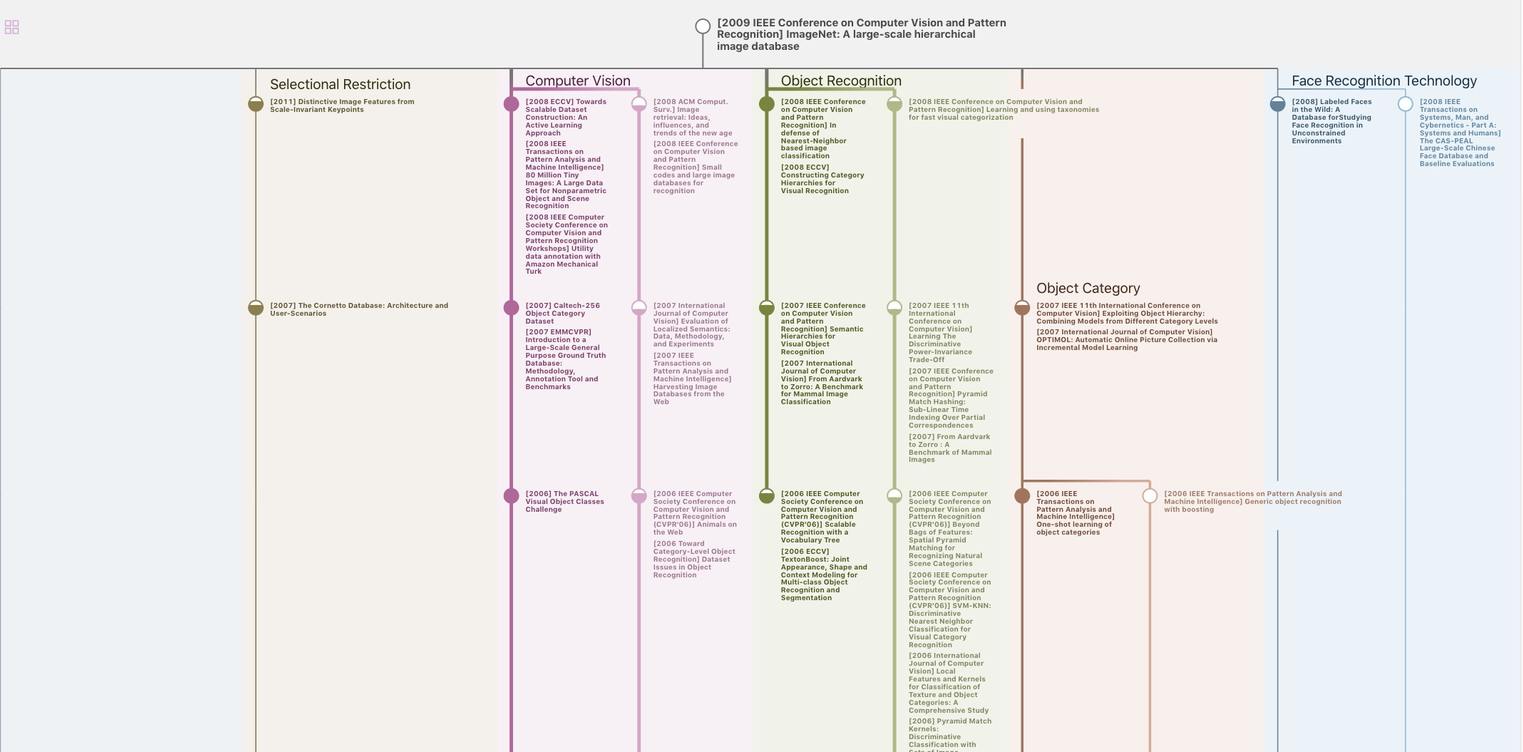
生成溯源树,研究论文发展脉络
Chat Paper
正在生成论文摘要