Efficient Sparse Matrix-Vector Multiplication on GPUs Using the CSR Storage Format
New Orleans, LA(2014)
摘要
The performance of sparse matrix vector multiplication (SpMV) is important to computational scientists. Compressed sparse row (CSR) is the most frequently used format to store sparse matrices. However, CSR-based SpMV on graphics processing units (GPUs) has poor performance due to irregular memory access patterns, load imbalance, and reduced parallelism. This has led researchers to propose new storage formats. Unfortunately, dynamically transforming CSR into these formats has significant runtime and storage overheads. We propose a novel algorithm, CSR-Adaptive, which keeps the CSR format intact and maps well to GPUs. Our implementation addresses the aforementioned challenges by (i) efficiently accessing DRAM by streaming data into the local scratchpad memory and (ii) dynamically assigning different numbers of rows to each parallel GPU compute unit. CSR-Adaptive achieves an average speedup of 14.7 × over existing CSR-based algorithms and 2.3× over clSpMV cocktail, which uses an assortment of matrix formats.
更多查看译文
关键词
graphics processing units,mathematics computing,matrix multiplication,parallel processing,sparse matrices,CSR storage format,CSR-adaptive,CSR-based SpMV,DRAM,clSpMV cocktail,compressed sparse row,graphics processing units,local scratchpad memory,parallel GPU compute unit,sparse matrix-vector multiplication,streaming data,AMD,Sparse matrix-vector multiplication (SpMV),compressed sparse row (CSR),general purpose computation on graphics processing units (GPGPU),performance acceleration
AI 理解论文
溯源树
样例
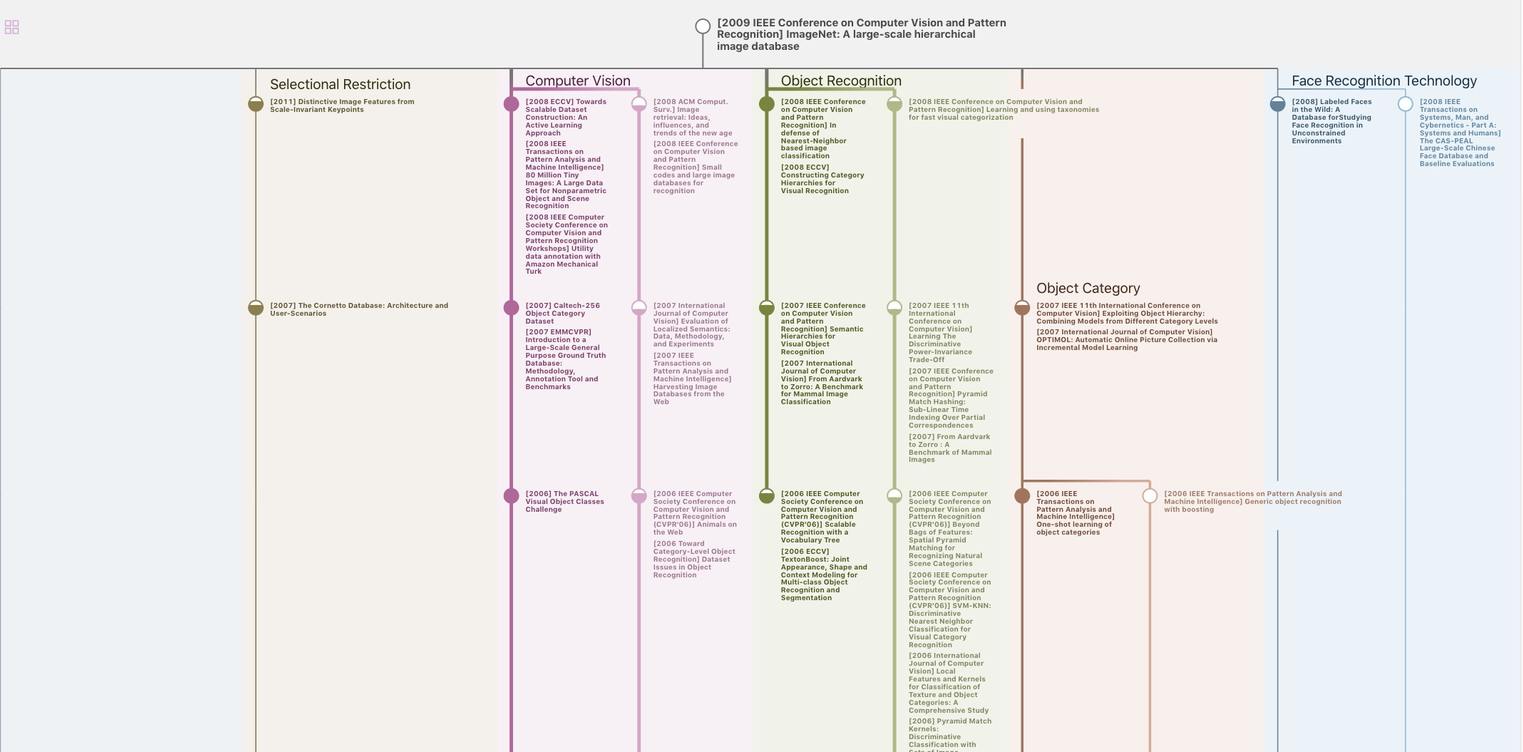
生成溯源树,研究论文发展脉络
Chat Paper
正在生成论文摘要