Crowdsourced stock clustering through equity analyst hypergraph partitioning.
IEEE Conference on Computational Intelligence for Financial Engineering and Economics CIFEr(2013)
摘要
Use of industry classifications in the finance community is pervasive. They are critical to deriving a balanced portfolio of stocks and, more broadly, to risk management. Businesses, academics and government agencies have all researched and developed various schemes with mixed success. Recognizing major brokerages and research firms tend to assign their analysts to cover highly similar companies, we propose a scheme that makes use of stock analyst coverage assignments. Although creating coverage groups of highly similar stocks is not the direct goal of research firms, it may be imperative to their success because increasing similarity in coverage helps maximize synergy and derive the most value per analyst. To create our industry scheme, we construct a hypergraph where vertices represent stocks and hyperedges represent analyst coverage, connecting his/her similar companies. Using no additional information, we perform hypergraph partitioning to form clusters of stocks. Our scalable scheme can produce any number of clusters and can automatically update as research firms change analyst coverage as opposed to today's leading industry schemes which have only fixed numbers of industries and require periodic expert review. Can our crowdsourced scheme match the quality of stock groups from the expert-driven schemes? We make head-to-head comparisons to a leading academic and a leading commercial scheme using a methodology from the finance community that measures the coincidence of stock price movements. We also compare our scheme against a clusterer that creates groups based on past return correlations. Our results rival and often exceed all three schemes.
更多查看译文
关键词
graph theory,correlation,taxonomy,entropy,industry classifications,investment,risk management
AI 理解论文
溯源树
样例
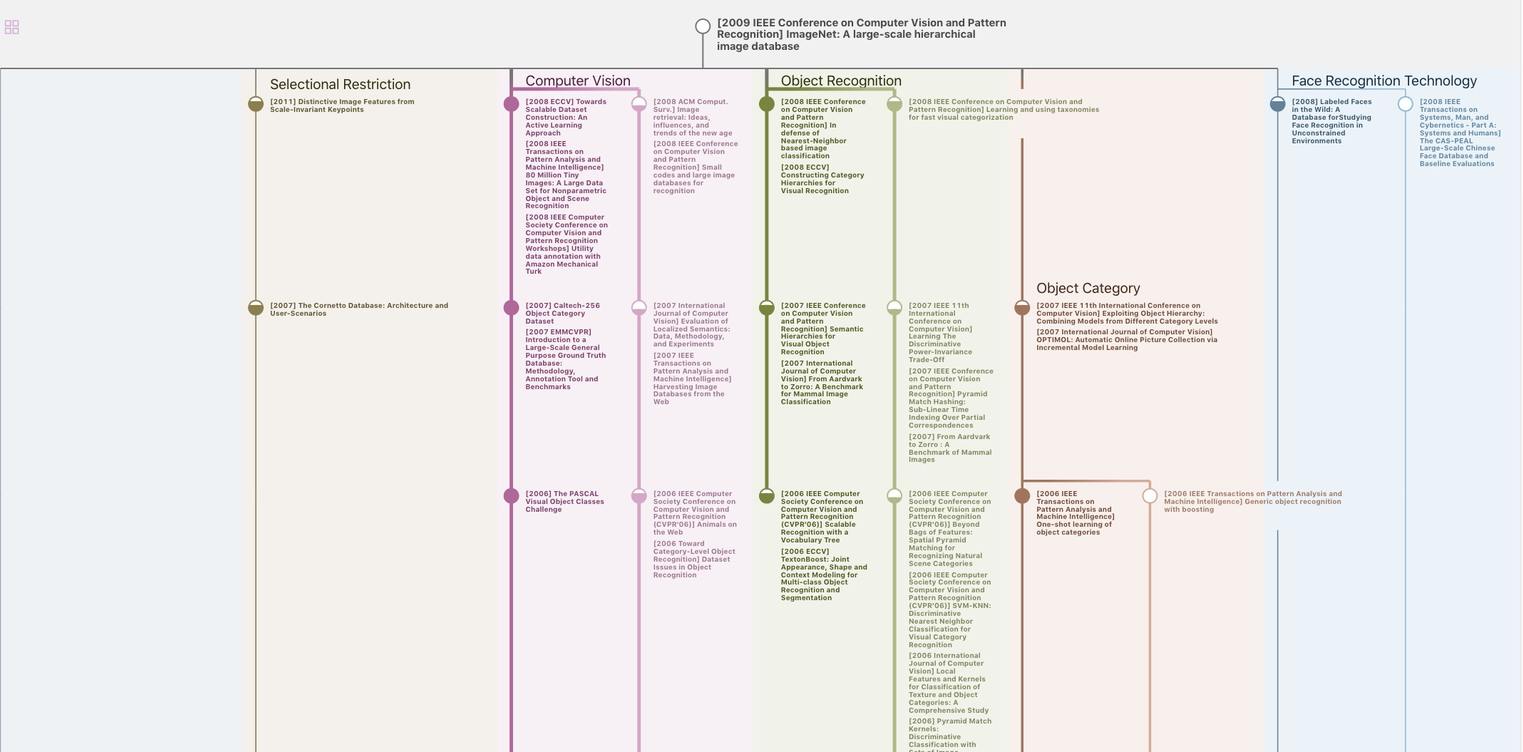
生成溯源树,研究论文发展脉络
Chat Paper
正在生成论文摘要