Approximating the crowd
Data Mining and Knowledge Discovery(2014)
摘要
The problem of “approximating the crowd” is that of estimating the crowd’s majority opinion by querying only a subset of it. Algorithms that approximate the crowd can intelligently stretch a limited budget for a crowdsourcing task. We present an algorithm, “CrowdSense,” that works in an online fashion where items come one at a time. CrowdSense dynamically samples subsets of the crowd based on an exploration/exploitation criterion. The algorithm produces a weighted combination of the subset’s votes that approximates the crowd’s opinion. We then introduce two variations of CrowdSense that make various distributional approximations to handle distinct crowd characteristics. In particular, the first algorithm makes a statistical independence approximation of the labelers for large crowds, whereas the second algorithm finds a lower bound on how often the current subcrowd agrees with the crowd’s majority vote. Our experiments on CrowdSense and several baselines demonstrate that we can reliably approximate the entire crowd’s vote by collecting opinions from a representative subset of the crowd.
更多查看译文
关键词
Crowdsourcing,Wisdom of crowds,Labeler quality estimation,Approximating the crowd,Aggregating opinions
AI 理解论文
溯源树
样例
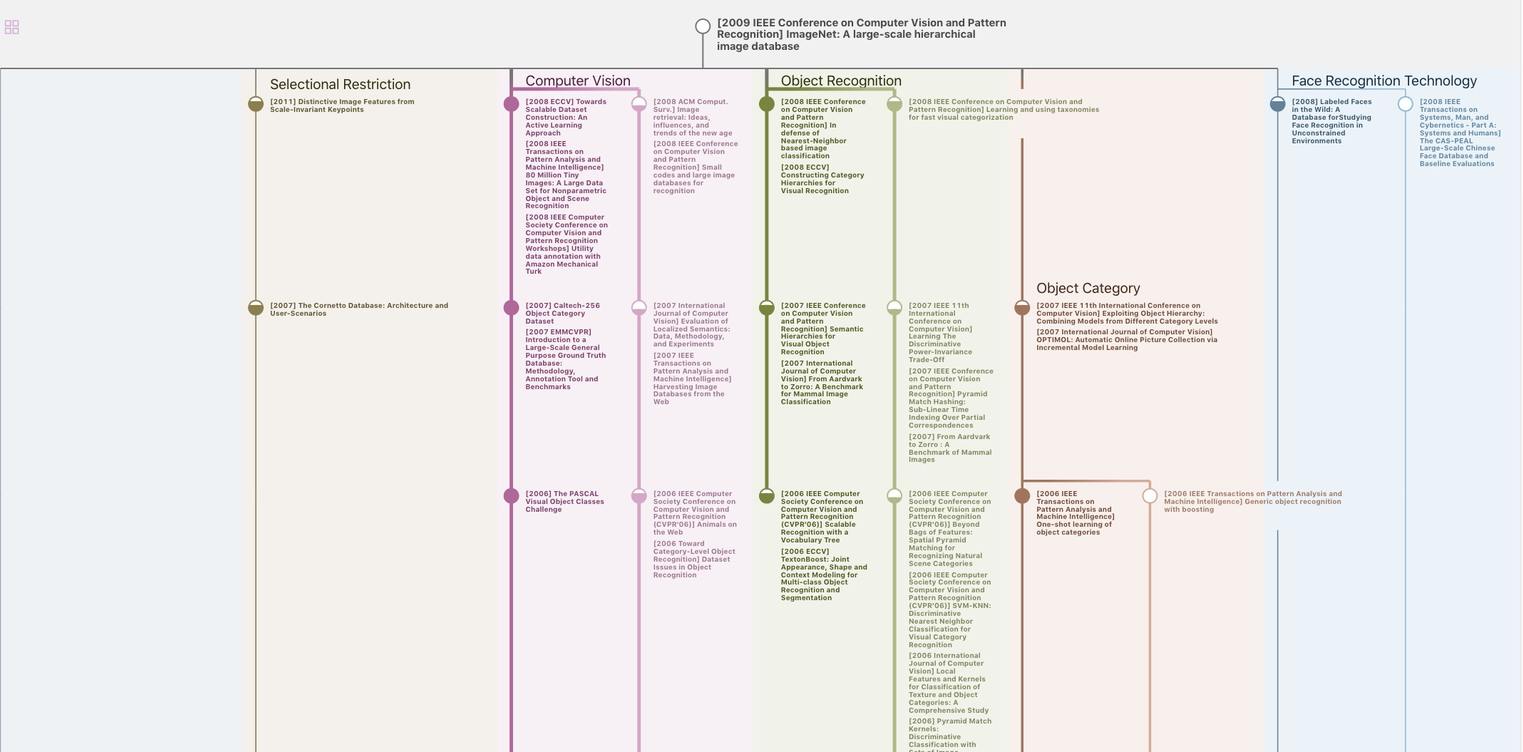
生成溯源树,研究论文发展脉络
Chat Paper
正在生成论文摘要