The normalized risk-averting error criterion for avoiding nonglobal local minima in training neural networks.
Neurocomputing(2015)
摘要
The convexification method for data fitting is capable of avoiding nonglobal local minima, but suffers from two shortcomings: the risk-averting error (RAE) criterion grows exponentially as its risk-sensitivity index λ increases, and the existing method of determining λ is often not effective. To eliminate these shortcomings, the normalized RAE (NRAE) is herein proposed. As NRAE is a monotone increasing function of RAE, the region without a nonglobal local minimum of NRAE expands as does that of RAE. However, NRAE does not grow unboundedly as does RAE.
更多查看译文
关键词
Neural network,Training,Convexification,Risk-averting error,Global optimization,Local minimum
AI 理解论文
溯源树
样例
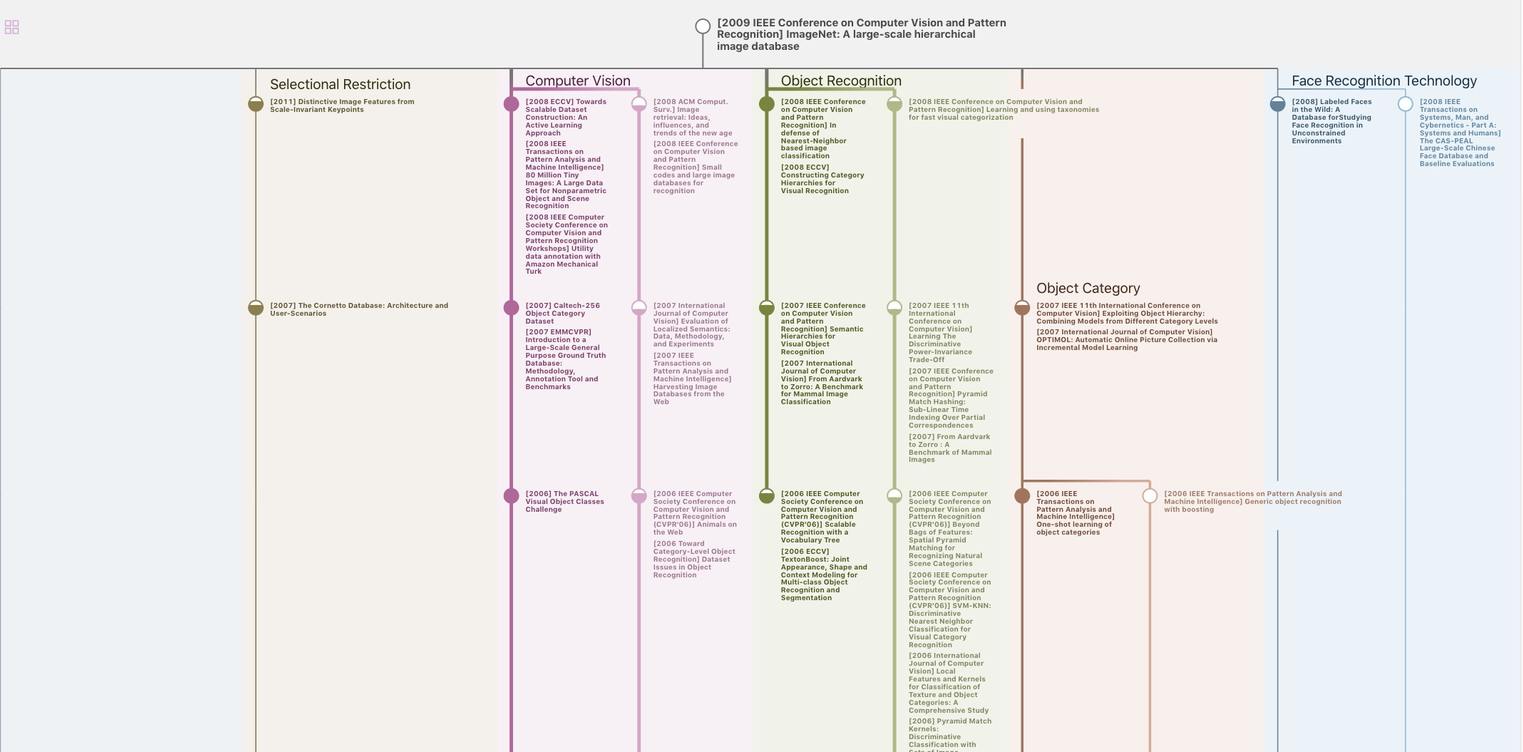
生成溯源树,研究论文发展脉络
Chat Paper
正在生成论文摘要