Multiple Instance Learning Using Visual Phrases For Object Classification
2010 IEEE INTERNATIONAL CONFERENCE ON MULTIMEDIA AND EXPO (ICME 2010)(2010)
摘要
Recently, bag of words (BoW) model has led to many significant results in visual object classification. However, due to the limited descriptive and discriminative ability of visual words, the resulting performance of visual object classification is still incomparable to its analogy in text domain, i.e. document categorization. Furthermore, for weakly labeled image data, where we only know whether an object is present or not, traditional learning based methods may suffer from background clutters and large appearance variations. To address these issues, we propose a novel visual phrase based Multiple Instance Learning (MIL) method. In this method, the visual phrase is first generated from over-segmented image regions of homogeneous appearance and visual words within each region, which may provide enhanced descriptive ability by enforcing the spatial coherency. Then a MIL algorithm is applied to efficiently learn from the weakly labeled image data. The experiments on benchmark datasets show that our proposed method always significantly outperforms several state-of-the-art algorithms, such as Spatial Pyramid Matching (SPM) [5] and Spatial-LTM [8].
更多查看译文
关键词
Visual Object Classification, Visual Phrase, Multiple Instance Learning
AI 理解论文
溯源树
样例
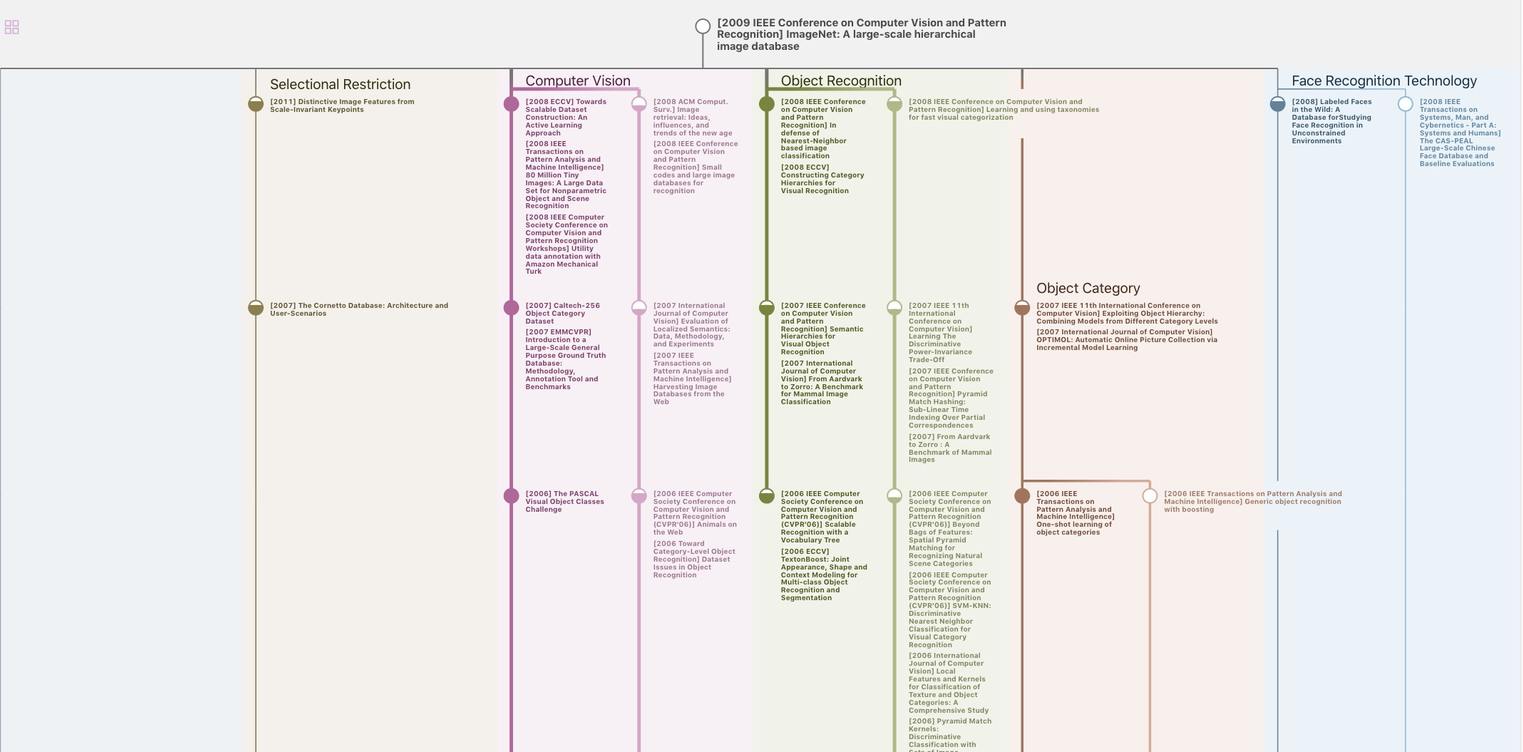
生成溯源树,研究论文发展脉络
Chat Paper
正在生成论文摘要