A Monte Carlo Update for Parametric POMDPs
Springer Tracts in Advanced Robotics(2010)
摘要
This paper presents the Parameterised POMDP (PPOMDP) algorithm: a method for planning in the space of continuous parameterised functions. The novel contribution is an approach to transitioning parameterised beliefs using Monte Carlo methods. By re-using prediction and observation calculations, the transition function can be computed efficiently. An analysis of scalability suggests that the approach is likely to scale to physically larger environments than algorithms which rely on an underlying discretisation. Experimental results in a simulated robot navigation problem show that the algorithm compares favourably with existing approaches.
更多查看译文
关键词
Reward Function, Partially Observable Markov Decision Process, Scalability Implication, Continuous State Space, Belief Space
AI 理解论文
溯源树
样例
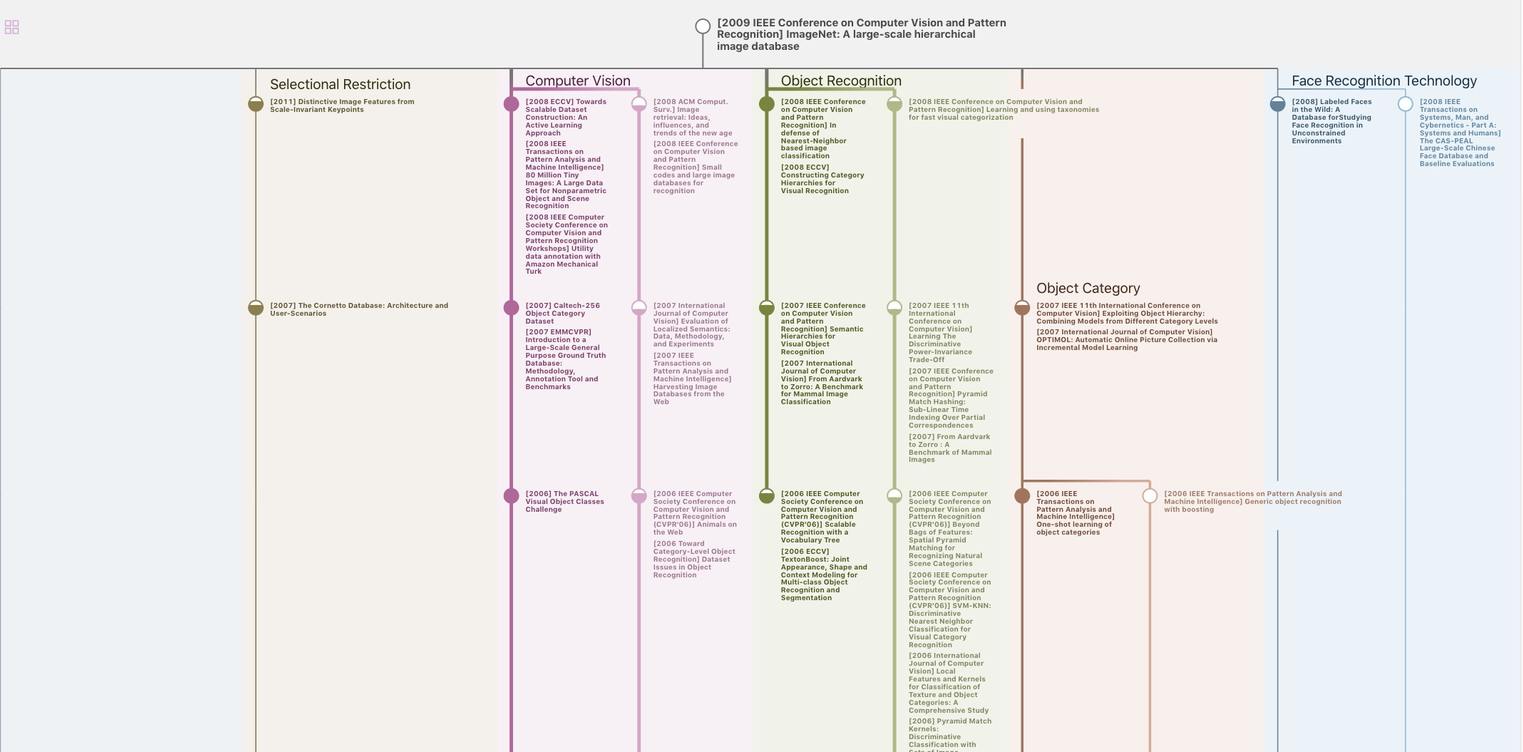
生成溯源树,研究论文发展脉络
Chat Paper
正在生成论文摘要