Group-Aware Disentangle Learning for Head Pose Estimation
PRCV(2021)
摘要
In this paper, we propose a group-aware disentangle learning (GADL) approach for robust head pose estimation. Conventional methods usually utilize landmarks or depth information to recover the head poses, likely leading to bias prediction due to that the performance of head pose estimation highly relies on landmark detection accuracy. Instead of using external information, our GADL estimates the head poses directly from facial images in a group-supervised manner. Specifically, we first map a group of input images that share a common orientation onto a latent space, then swap the shared orientation subspaces across images to reorganize the representation. Next, we use the decoder to synthetic the original images via the reorganized representation. Thus, we explicitly disentangle the pose-relevant features shared by a group of facial images. Extensive experimental results on the challenging widely-evaluated datasets indicate the superiority of our GADL compared with the state of the arts.
更多查看译文
关键词
Head pose estimation,Group-supervised learning,Feature disentangle
AI 理解论文
溯源树
样例
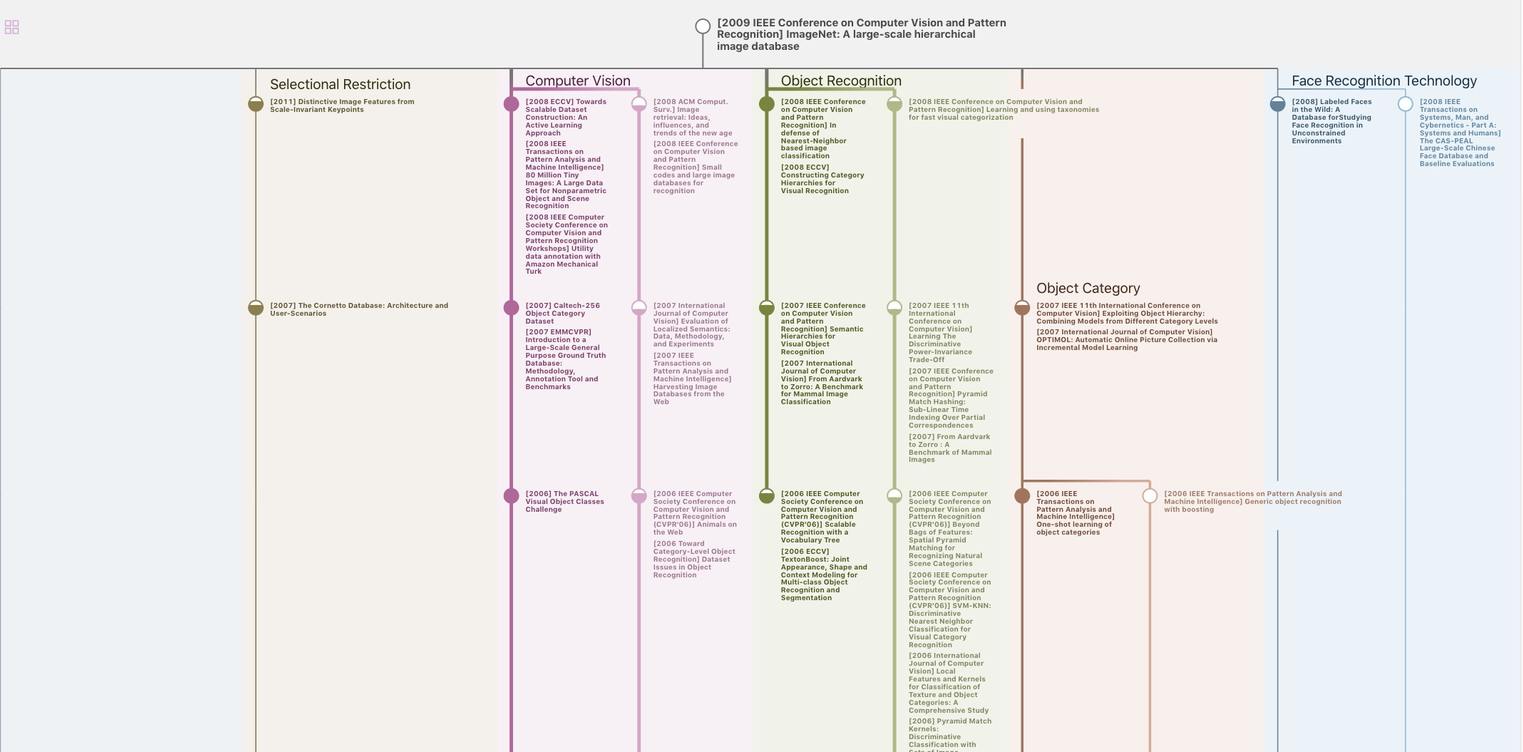
生成溯源树,研究论文发展脉络
Chat Paper
正在生成论文摘要