A dynamic decision network framework for online media adaptation in stroke rehabilitation
TOMCCAP(2008)
摘要
In this article, we present a media adaptation framework for an immersive biofeedback system for stroke patient rehabilitation. In our biofeedback system, media adaptation refers to changes in audio/visual feedback as well as changes in physical environment. Effective media adaptation frameworks help patients recover generative plans for arm movement with potential for significantly shortened therapeutic time. The media adaptation problem has significant challenges—(a) high dimensionality of adaptation parameter space; (b) variability in the patient performance across and within sessions; (c) the actual rehabilitation plan is typically a non-first-order Markov process, making the learning task hard. Our key insight is to understand media adaptation as a real-time feedback control problem. We use a mixture-of-experts based Dynamic Decision Network (DDN) for online media adaptation. We train DDN mixtures per patient, per session. The mixture models address two basic questions—(a) given a specific adaptation suggested by the domain experts, predict the patient performance, and (b) given the expected performance, determine the optimal adaptation decision. The questions are answered through an optimality criterion based search on DDN models trained in previous sessions. We have also developed new validation metrics and have very good results for both questions on actual stroke rehabilitation data.
更多查看译文
关键词
online media adaptation,media adaptation problem,stroke rehabilitation,effective media adaptation framework,media adaptation framework,optimal adaptation decision,mixture of experts,stroke patient rehabilitation,biofeedback,specific adaptation,adaptation parameter space,patient performance,dynamic decision network framework,dynamic decision network,media adaptation,parameter space,mixture model,real time,markov process,first order,feedback control
AI 理解论文
溯源树
样例
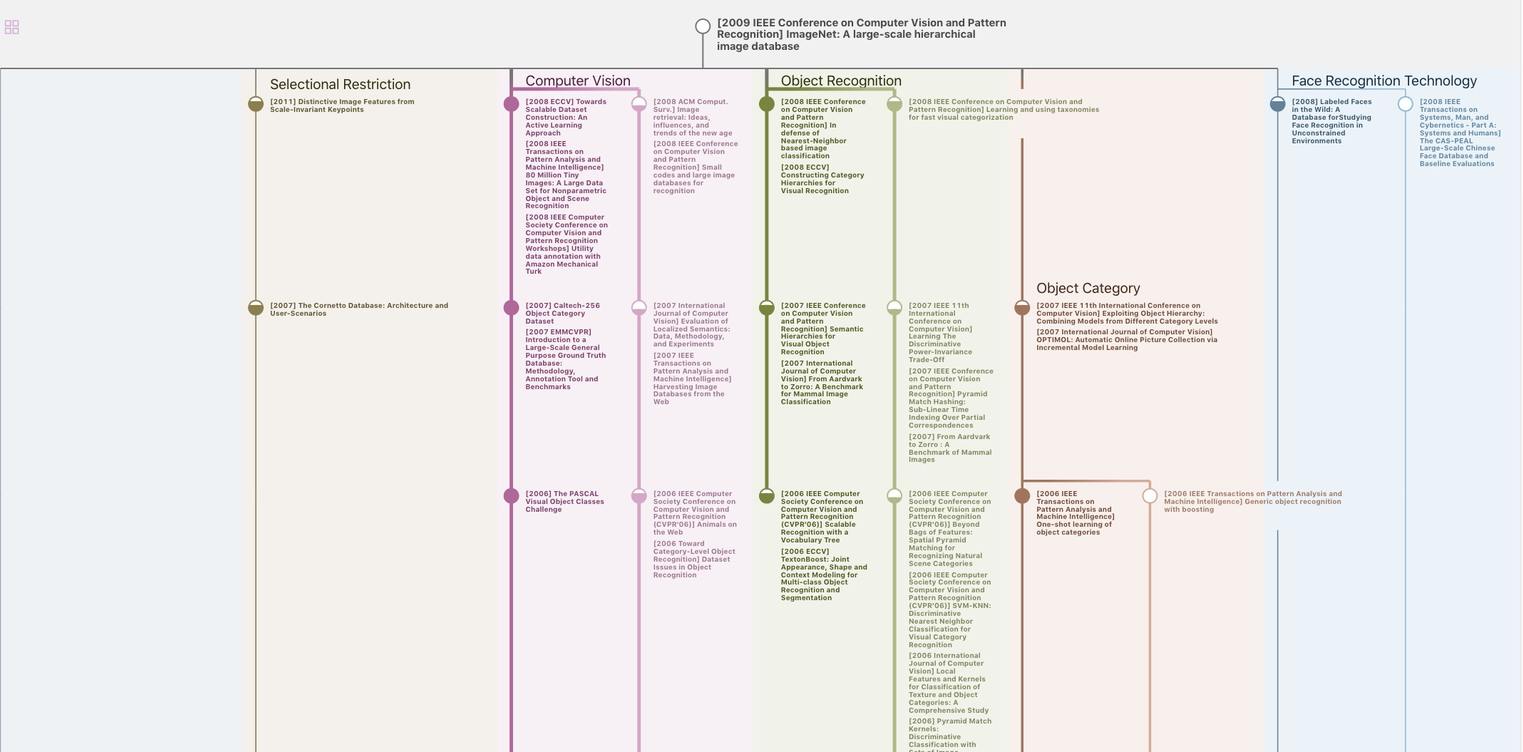
生成溯源树,研究论文发展脉络
Chat Paper
正在生成论文摘要