A Bayes Evaluation Criterion for Decision Trees
ADVANCES IN KNOWLEDGE DISCOVERY AND MANAGEMENT(2010)
摘要
We present a new evaluation criterion for the induction of decision trees. We exploit a parameter-free Bayesian approach and propose an analytic formula for the evaluation of the posterior probability of a decision tree given the data. We thus transform the training problem into an optimization problem in the space of decision tree models, and search for the best tree, which is the maximum a posteriori (MAP) one. The optimization is performed using top-down heuristics with pre-pruning and post-pruning processes. Extensive experiments on 30 UCI datasets and on the 5 WCCI 2006 performance prediction challenge datasets show that our method obtains predictive performance similar to that of alternative state-of-the-art methods, with far simpler trees.
更多查看译文
关键词
Decision Tree,Bayesian Optimization,Minimum Description Length,Supervised Learning,Model Selection
AI 理解论文
溯源树
样例
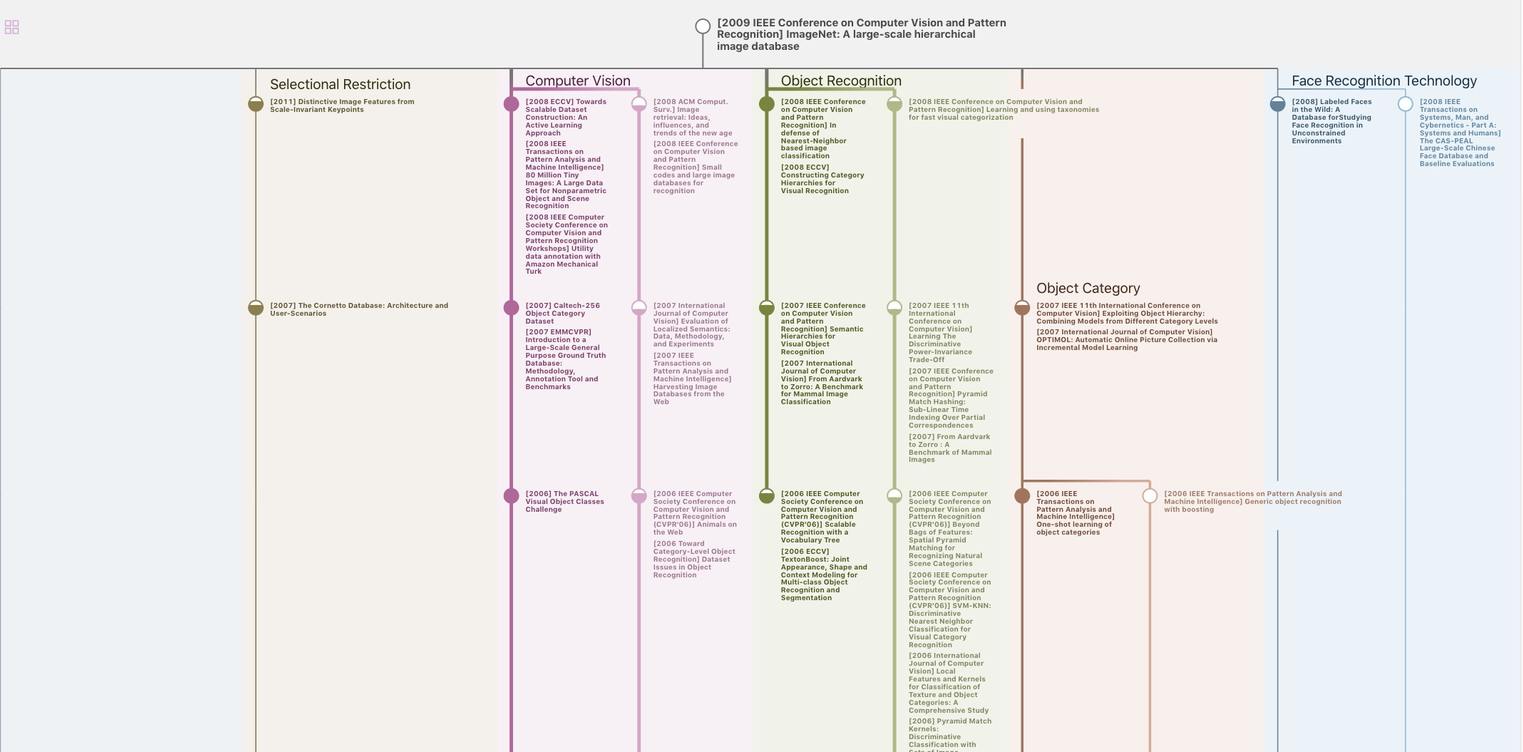
生成溯源树,研究论文发展脉络
Chat Paper
正在生成论文摘要