Compressive oversampling for robust data transmission in sensor networks
INFOCOM(2010)
摘要
Data loss in wireless sensing applications is inevitable and while there have been many attempts at coping with this issue, recent developments in the area of Compressive Sensing (CS) provide a new and attractive perspective. Since many physical signals of interest are known to be sparse or compressible, employing CS, not only compresses the data and reduces effective transmission rate, but also improves the robustness of the system to channel erasures. This is possible because reconstruction algorithms for compressively sampled signals are not hampered by the stochastic nature of wireless link disturbances, which has traditionally plagued attempts at proactively handling the effects of these errors. In this paper, we propose that if CS is employed for source compression, then CS can further be exploited as an application layer erasure coding strategy for recovering missing data. We show that CS erasure encoding (CSEC) with random sampling is efficient for handling missing data in erasure channels, paralleling the performance of BCH codes, with the added benefit of graceful degradation of the reconstruction error even when the amount of missing data far exceeds the designed redundancy. Further, since CSEC is equivalent to nominal oversampling in the incoherent measurement basis, it is computationally cheaper than conventional erasure coding. We support our proposal through extensive performance studies.
更多查看译文
关键词
wireless link disturbance,compressive oversampling,cs erasure encoding,sensor network,reconstruction error,reconstruction algorithm,application layer erasure,robust data transmission,data loss,extensive performance study,conventional erasure coding,missing data,erasure channel,wireless sensor networks,coding,channels,bch code,erasure coding,stochastic processes,data transmission,decoding,redundancy,compressed sensing,compressive sampling,channel coding,erasure code,compressive sensing,signals,robustness,compression,networks,sensors,degradation,detectors,algorithms,sampling methods,graceful degradation,random sampling,sampling,data compression,physical properties,measurement
AI 理解论文
溯源树
样例
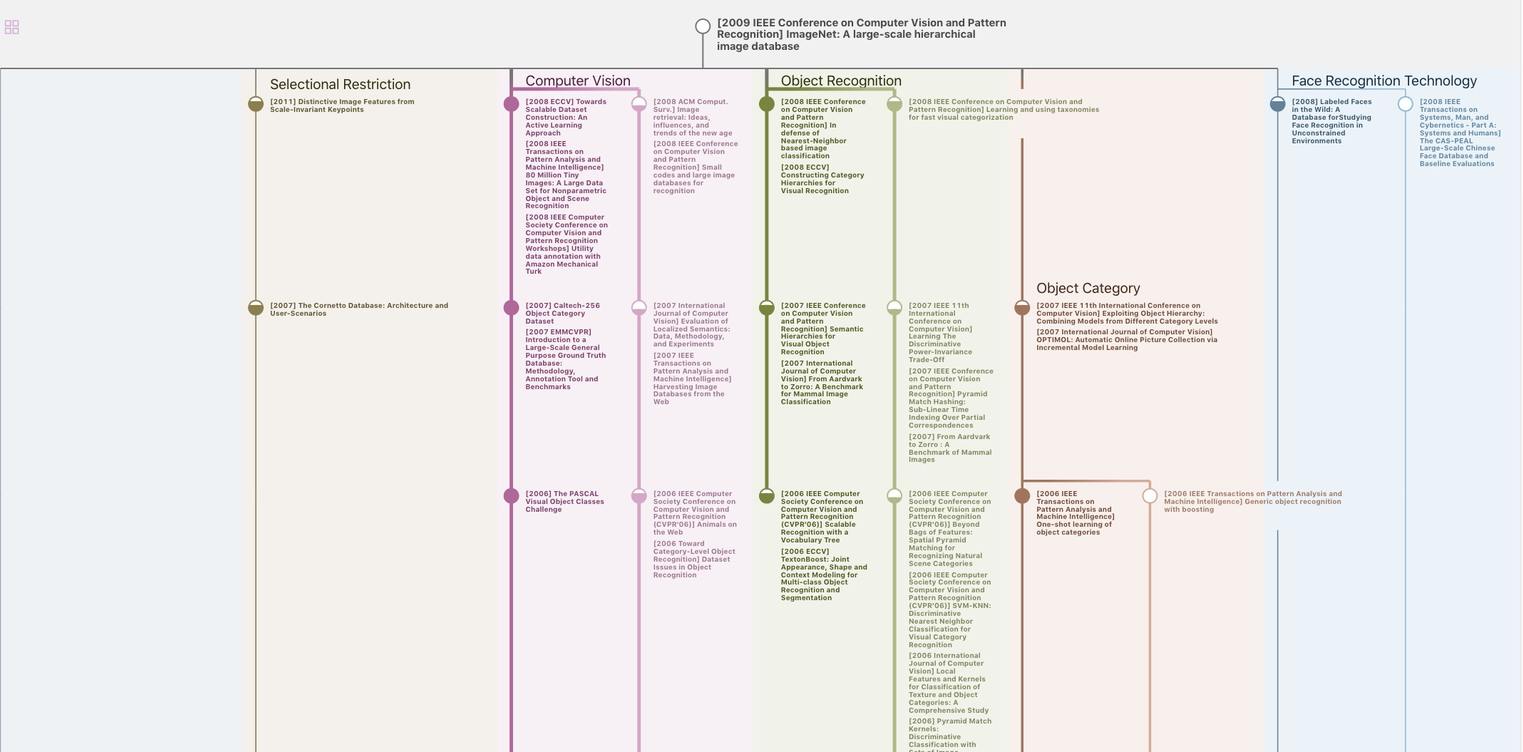
生成溯源树,研究论文发展脉络
Chat Paper
正在生成论文摘要