Data Transformations Enabling Loop Vectorization On Multithreaded Data Parallel Architectures
PPOPP(2010)
摘要
Loop vectorization, a key feature exploited to obtain high performance on Single Instruction Multiple Data (SIMD) vector architectures, is significantly hindered by irregular memory access patterns in the data stream. This paper describes data transformations that allow us to vectorize loops targeting massively multithreaded data parallel architectures. We present a mathematical model that captures loop-based memory access patterns and computes the most appropriate data transformations in order to enable vectorization. Our experimental results show that the proposed data transformations can significantly increase the number of loops that can be vectorized and enhance the data-level parallelism of applications. Our results also show that the overhead associated with our data transformations can be easily amortized as the size of the input data set increases. For the set of high performance benchmark kernels studied, we achieve consistent and significant performance improvements (up to 11.4X) by applying vectorization using our data transformation approach.
更多查看译文
关键词
Loop Vectorization,Data Transformation,GPGPU
AI 理解论文
溯源树
样例
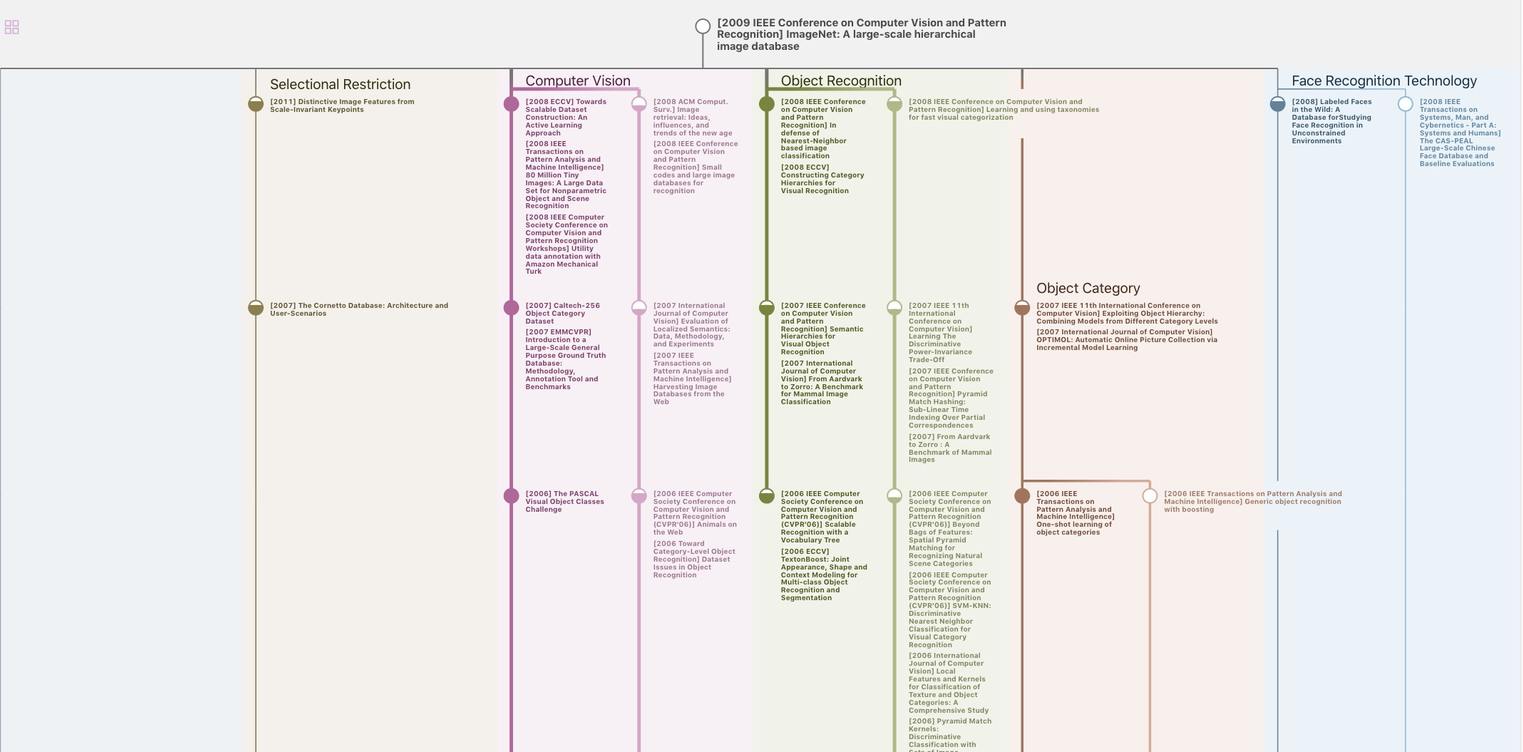
生成溯源树,研究论文发展脉络
Chat Paper
正在生成论文摘要