Can movies and books collaborate?: cross-domain collaborative filtering for sparsity reduction
IJCAI(2009)
摘要
The sparsity problem in collaborative filtering (CF) is a major bottleneck for most CF methods. In this paper, we consider a novel approach for alleviating the sparsity problem in CF by transferring useritem rating patterns from a dense auxiliary rating matrix in other domains (e.g., a popular movie rating website) to a sparse rating matrix in a target domain (e.g., a new book rating website). We do not require that the users and items in the two domains be identical or even overlap. Based on the limited ratings in the target matrix, we establish a bridge between the two rating matrices at a cluster-level of user-item rating patterns in order to transfer more useful knowledge from the auxiliary task domain. We first compress the ratings in the auxiliary rating matrix into an informative and yet compact cluster-level rating pattern representation referred to as a codebook. Then, we propose an efficient algorithm for reconstructing the target rating matrix by expanding the codebook. We perform extensive empirical tests to show that our method is effective in addressing the data sparsity problem by transferring the useful knowledge from the auxiliary tasks, as compared to many state-of-the-art CF methods.
更多查看译文
关键词
sparse rating matrix,sparsity problem,auxiliary rating matrix,rating matrix,dense auxiliary rating matrix,cross-domain collaborative,sparsity reduction,new book rating website,limited rating,compact cluster-level rating pattern,target rating matrix,popular movie rating website,collaborative filtering
AI 理解论文
溯源树
样例
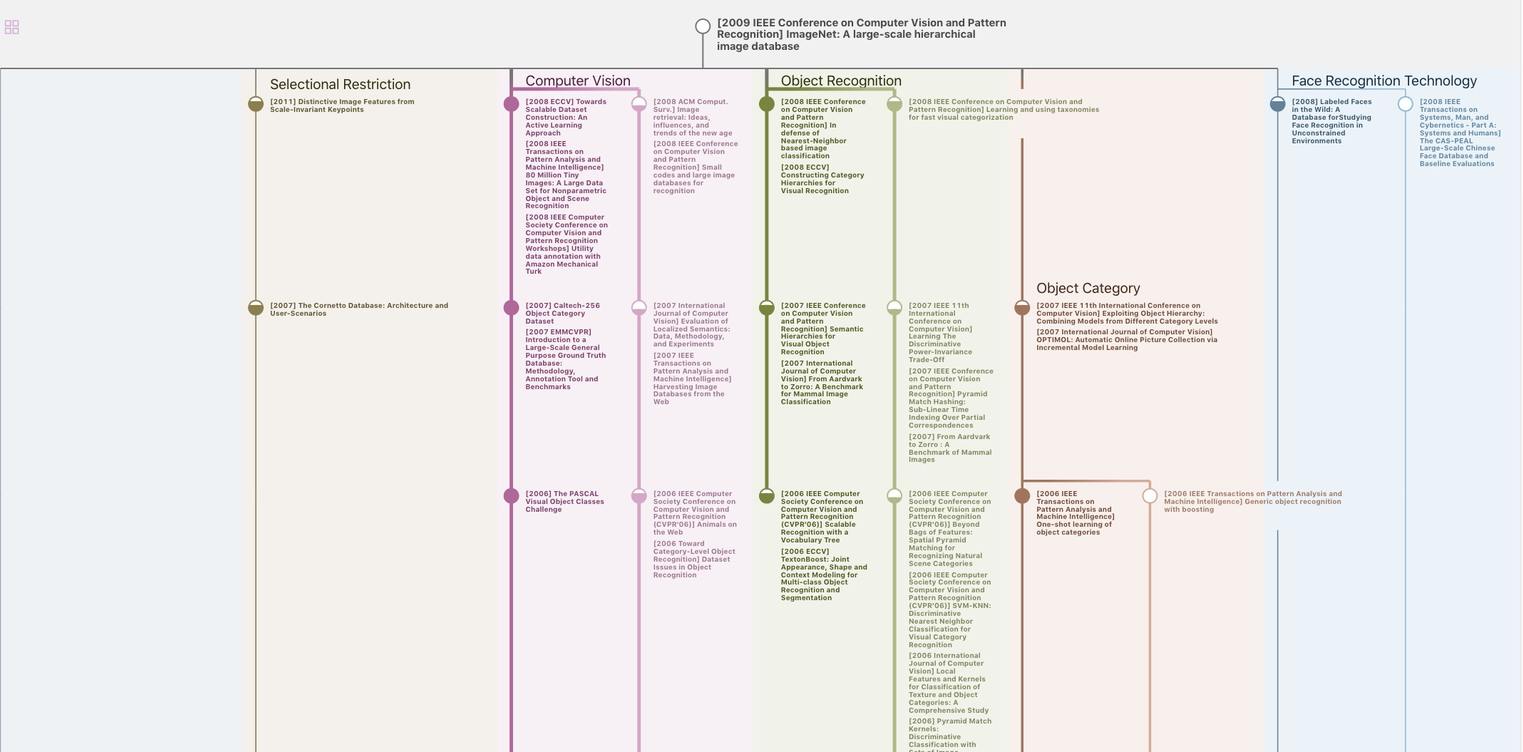
生成溯源树,研究论文发展脉络
Chat Paper
正在生成论文摘要