Multi-Modal Hierarchical Dirichlet Process Model for Predicting Image Annotation and Image-Object Label Correspondence
siam international conference on data mining(2009)
摘要
Many real-world applications call for learning predictive relationships from multi-modal data. In particular, in multi-media and web applications, given a dataset of images and their associated captions, one might want to construct a predictive model that not only predicts a caption for the image but also labels the individual objects in the image. We address this problem us- ing a multi-modal hierarchical Dirichlet Process model (MoM-HDP) - a stochastic process for modeling multi- modal data. MoM-HDP is an analog of a multi-modal Latent Dirichlet Allocation (MoM-LDA) with an infi- nite number of mixture components. Thus MoM-HDP allows circumventing the need for a priori choice of the number of mixture components or the computational expense of model selection. During training, the model has access to an un-segmented image and its caption, but not the labels for each object in the image. The trained model is used to predict the label for each region of interest in a segmented image. The model parameters are estimated efficiently using variational inference. We use two large benchmark datasets to compare the per- formance of the proposed MoM-HDP model with that of MoM-LDA model as well as some simple alternatives: Naive Bayes and Logistic Regression classifiers based on the formulation of the image annotation and image- label correspondence problems as one-against-all clas- sification. Our experimental results show that unlike MoM-LDA, the performance of MoM-HDP is invariant to the number of mixture components. Furthermore, our experimental evaluation shows that the generaliza- tion performance of MoM-HDP is superior to that of MoM-HDP as well as the one-against-all Naive Bayes and Logistic Regression classifiers.
更多查看译文
关键词
stochastic process,correspondence problem,region of interest,hierarchical dirichlet process,model selection,prediction model,image annotation,logistic regression,latent dirichlet allocation,naive bayes
AI 理解论文
溯源树
样例
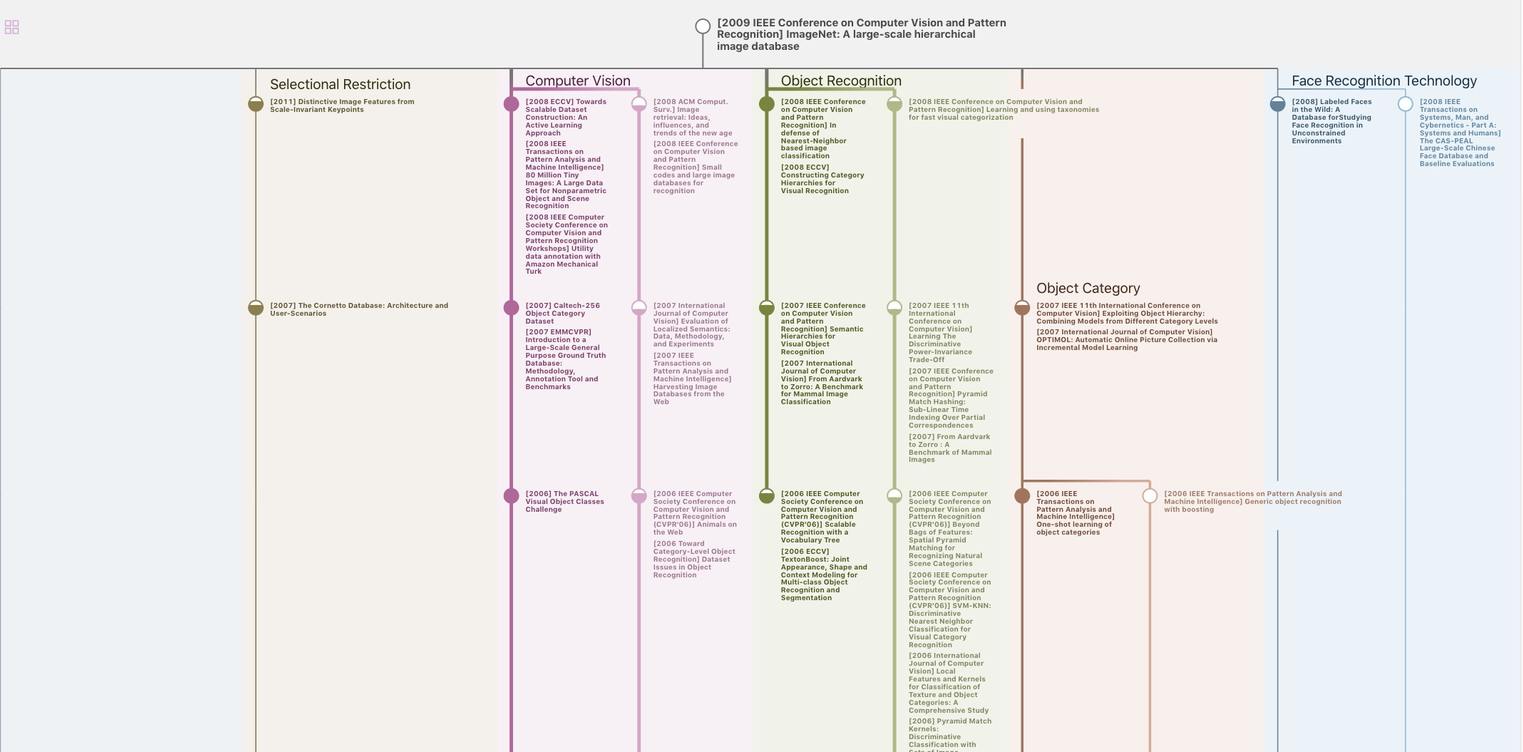
生成溯源树,研究论文发展脉络
Chat Paper
正在生成论文摘要