Telling Two Distributions Apart: a Tight Characterization
CoRR(2011)
摘要
We consider the problem of distinguishing between two arbitrary black-box distributions defined over the domain [n], given access to $s$ samples from both. It is known that in the worst case O(n^{2/3}) samples is both necessary and sufficient, provided that the distributions have L1 difference of at least {\epsilon}. However, it is also known that in many cases fewer samples suffice. We identify a new parameter, that provides an upper bound on how many samples needed, and present an efficient algorithm that requires the number of samples independent of the domain size. Also for a large subclass of distributions we provide a lower bound, that matches our upper bound up to a poly-logarithmic factor.
更多查看译文
关键词
lower bound,data structure,upper bound
AI 理解论文
溯源树
样例
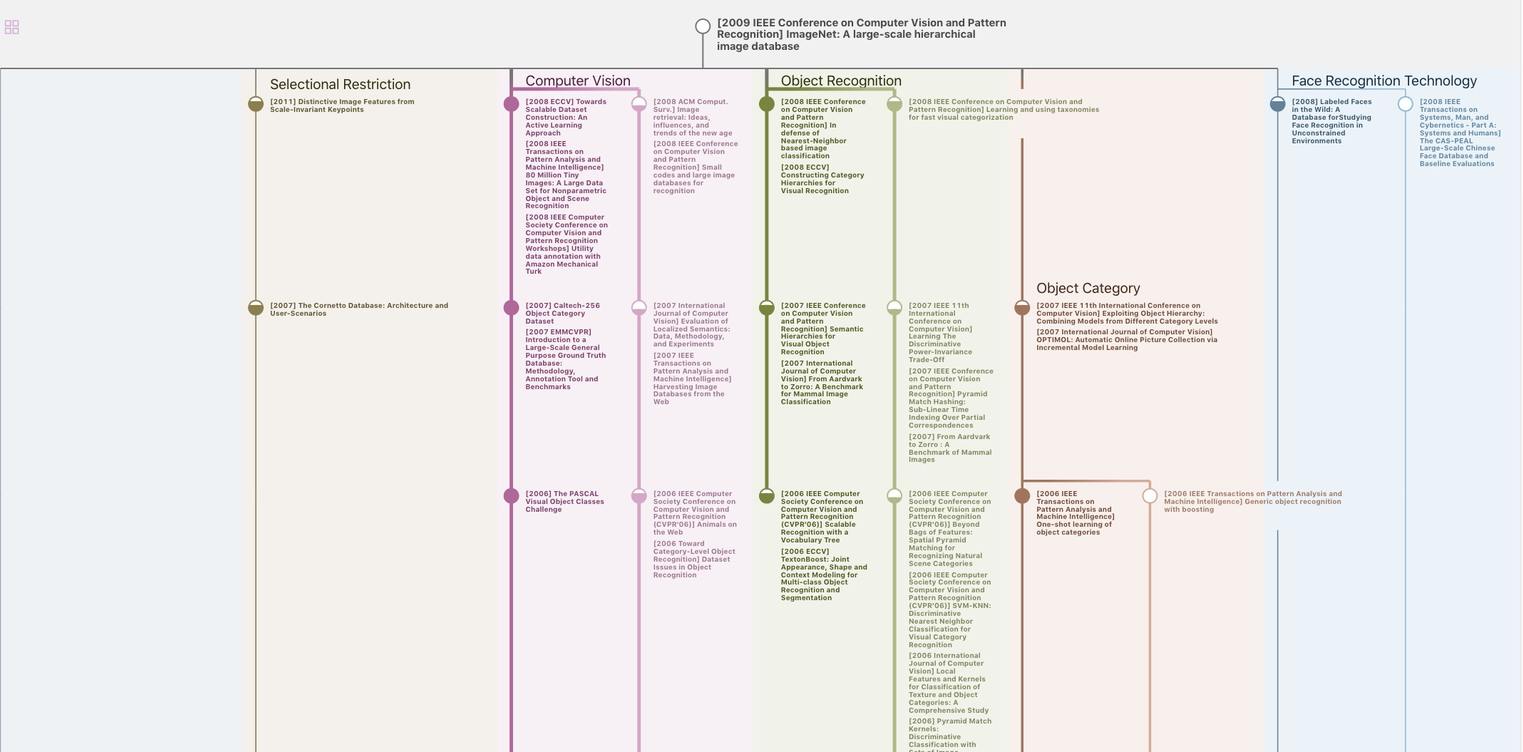
生成溯源树,研究论文发展脉络
Chat Paper
正在生成论文摘要