Integrated CPU and l2 cache voltage scaling using machine learning
Proceedings of the 2007 ACM SIGPLAN/SIGBED conference on Languages, compilers, and tools for embedded systems(2007)
摘要
Embedded systems serve an emerging and diverse set of applications. As a result, more computational and storage capabilities are added to accommodate ever more demanding applications. Unfortunately, adding more resources typically comes on the expense of higher energy costs. New chip design with Multiple Clock Domains (MCD) opens the opportunity for fine-grain power management within theprocessor chip. When used with dynamic voltage scaling (DVS), we can control the voltage and power of each domain independently. A significant power and energy improvement has been shown when using MCD design in comparison to managing a single voltage domain for the whole chip, as in traditional chips with global DVS. In this paper, we propose PACSL a Power-Aware Compiler-based approach using Supervised Learning. PACSL automatically derives an integrated CPU-core and on-chip L2 cache DVS policy tailored to a specific system and workload. Our approach uses supervised machine learning to discover a policy, which relies on monitoring a few performance counters. We present our approach detailing the role of a compiler in constructing a custom power management policy. We also discuss some implementation issues associated with our technique. We show that PACSL improves on traditional power management techniques that are used in general MCD chips. Our technique saves 22% on average (up to 46%) in energy-delay product over a DVS technique that applies independent DVS decisions in each domain. Compared to no-power management, our technique improves energy-delay product by 26% on average (up to 64%).
更多查看译文
关键词
embedded system,machine learning,supervised learning,chip
AI 理解论文
溯源树
样例
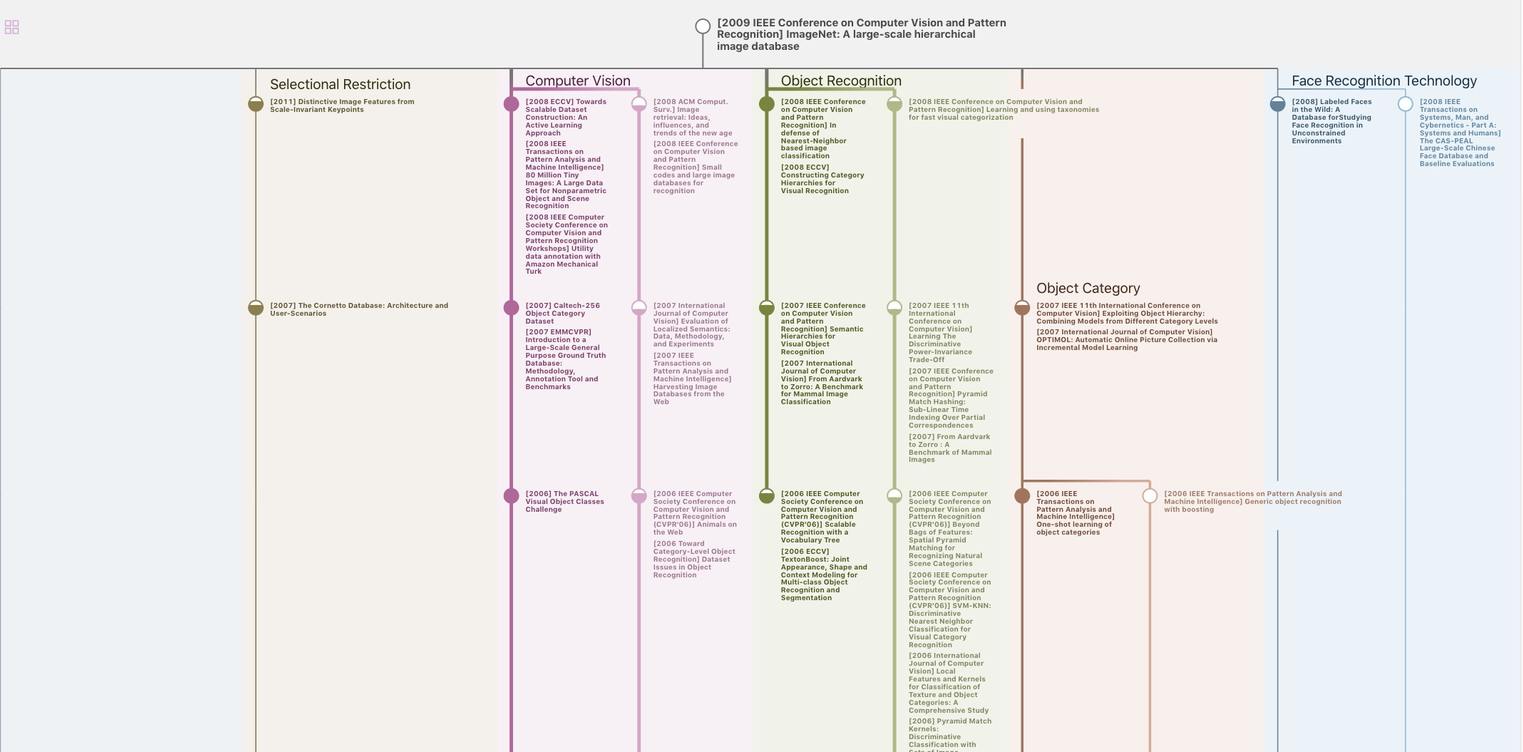
生成溯源树,研究论文发展脉络
Chat Paper
正在生成论文摘要