Influence of graph construction on graph-based clustering measures
NIPS(2008)
摘要
Graph clustering methods such as spectral clustering are defined for general weighted graphs. In machine learning, however, data often is not given in form of a graph, but in terms of similarity (or distance) values between points. In this case, first a neighborhood graph is constructed using the similarities between the points and then a graph clustering algorithm is applied to this graph. In this pa- per we investigate the influence of the construction of the similarity graph on the clustering results. We first study the convergence of graph clustering crite- ria such as the normalized cut (Ncut) as the sample size tends to infinity. We find that the limit expressions are different for different types of graph, for exam- ple the r-neighborhood graph or the k-nearest neighbor graph. In plain words: Ncut on a kNN graph does something systematically different than Ncut on an r-neighborhood graph! This finding shows that graph clustering criteria cannot be studied independently of the kind of graph they are applied to. We also provide examples which show that these differences can be observed for toy and real data already for rather small sample sizes.
更多查看译文
关键词
machine learning,graph clustering,k nearest neighbor,sample size,spectral clustering
AI 理解论文
溯源树
样例
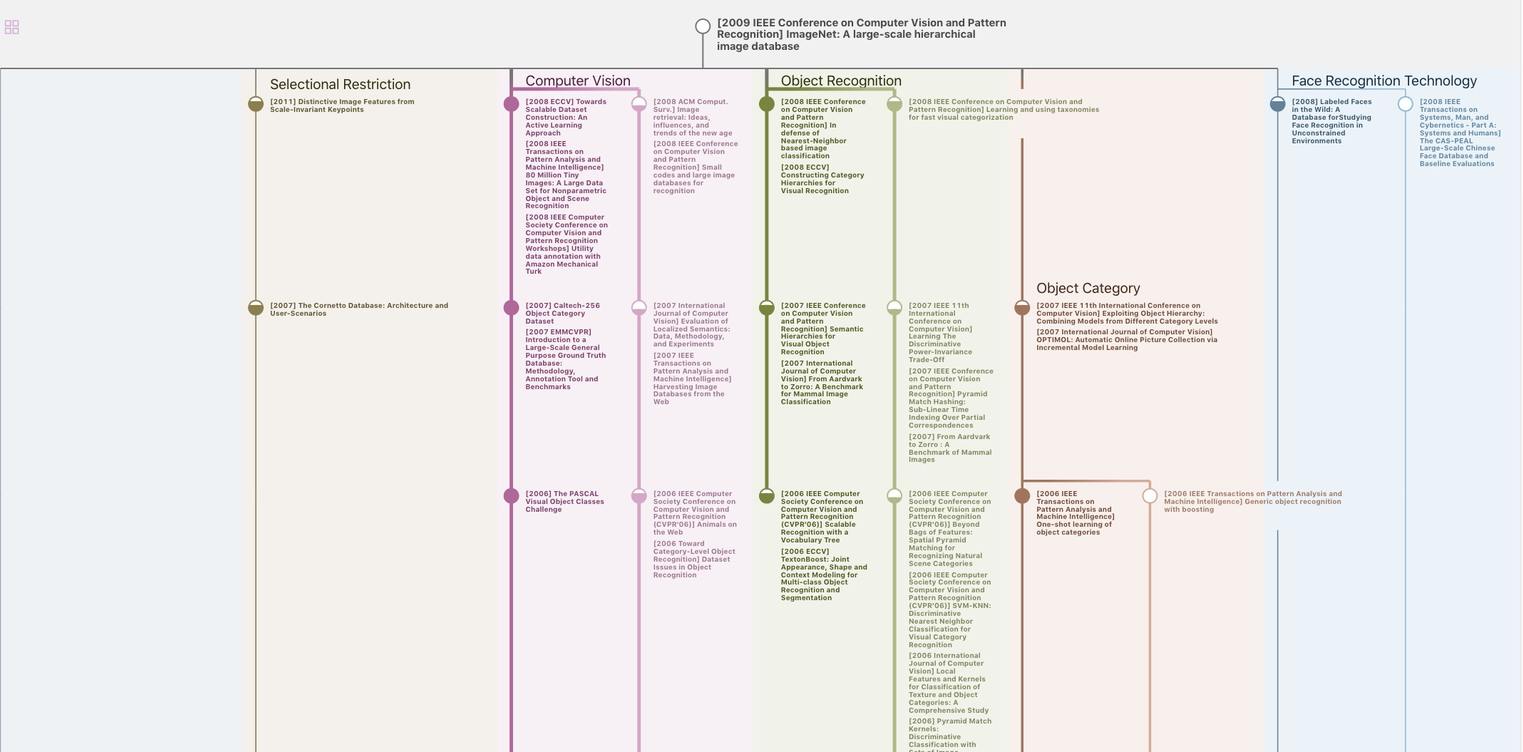
生成溯源树,研究论文发展脉络
Chat Paper
正在生成论文摘要