Global Models of Document Structure using Latent Permutations.
NAACL '09: Proceedings of Human Language Technologies: The 2009 Annual Conference of the North American Chapter of the Association for Computational Linguistics(2009)
摘要
We present a novel Bayesian topic model for learning discourse-level document structure. Our model leverages insights from discourse theory to constrain latent topic assignments in a way that reflects the underlying organization of document topics. We propose a global model in which both topic selection and ordering are biased to be similar across a collection of related documents. We show that this space of orderings can be elegantly represented using a distribution over permutations called the generalized Mallows model . Our structure-aware approach substantially outperforms alternative approaches for cross-document comparison and single-document segmentation.
更多查看译文
关键词
generalized Mallows model,global model,model leverages insight,novel Bayesian topic model,document topic,latent topic assignment,topic selection,discourse-level document structure,related document,alternative approach,latent permutation
AI 理解论文
溯源树
样例
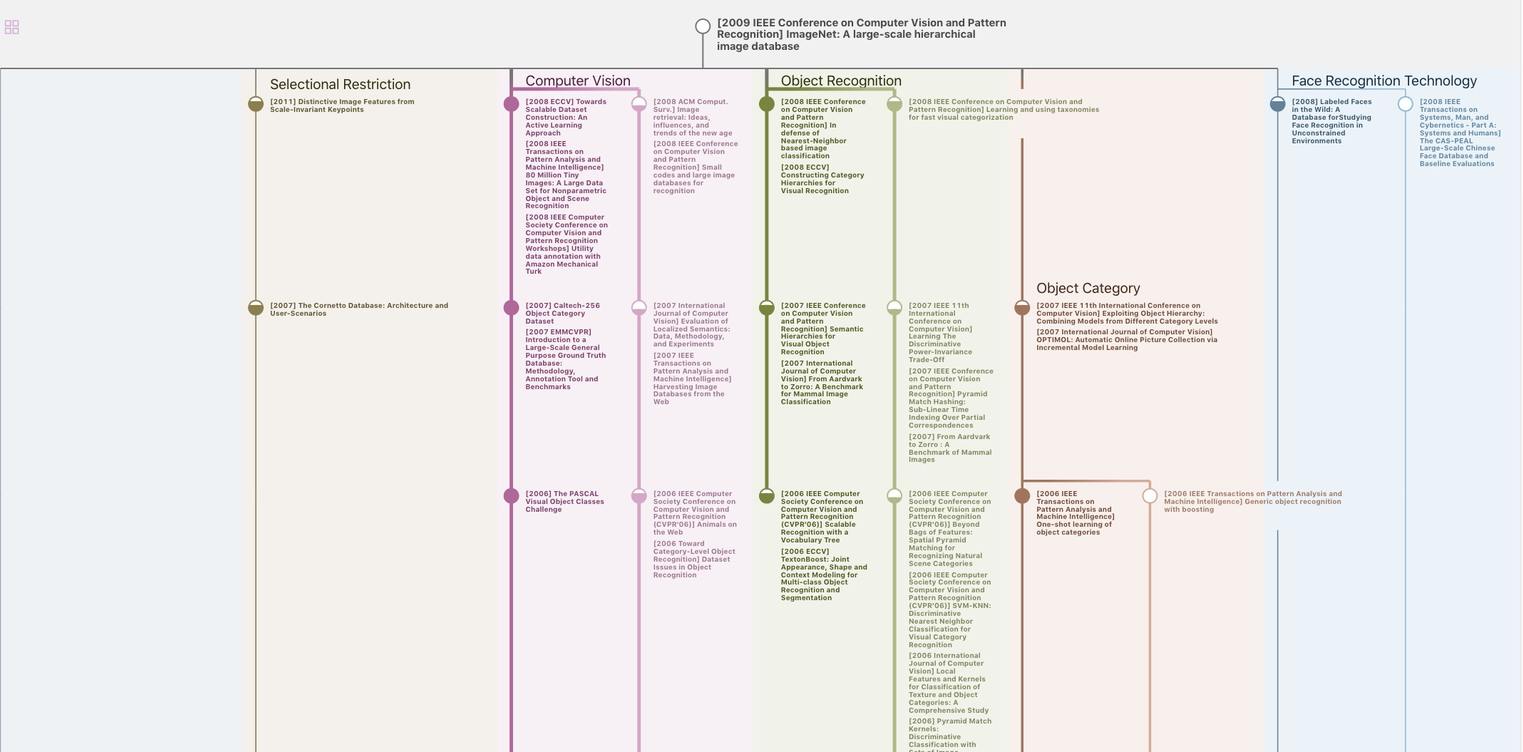
生成溯源树,研究论文发展脉络
Chat Paper
正在生成论文摘要