On-line learning with malicious noise and the closure algorithm
Electronic Colloquium on Computational Complexity (ECCC)(1998)
摘要
We investigate a variant of the on-line learning model for classes of \0,1\-valued functions (concepts) in which the labels of a certain amount of the input instances are corrupted by adversarial noise. We propose an extension of a general learning strategy, known as “Closure Algorithm”, to this noise model, and show a worst-case mistake bound of m + (d+1)K for learning an arbitrary intersection-closed concept class C, where K is the number of noisy labels, d is a combinatorial parameter measuring C's complexity, and m is the worst-case mistake bound of the Closure Algorithm for learning C in the noise-free model. For several concept classes our extended Closure Algorithm is efficient and can tolerate a noise rate up to the information-theoretic upper bound. Finally, we show how to efficiently turn any algorithm for the on-line noise model into a learning algorithm for the PAC model with malicious noise.
更多查看译文
关键词
Boolean Function,Concept Class,Target Class,Noise Rate,Hypothesis Class
AI 理解论文
溯源树
样例
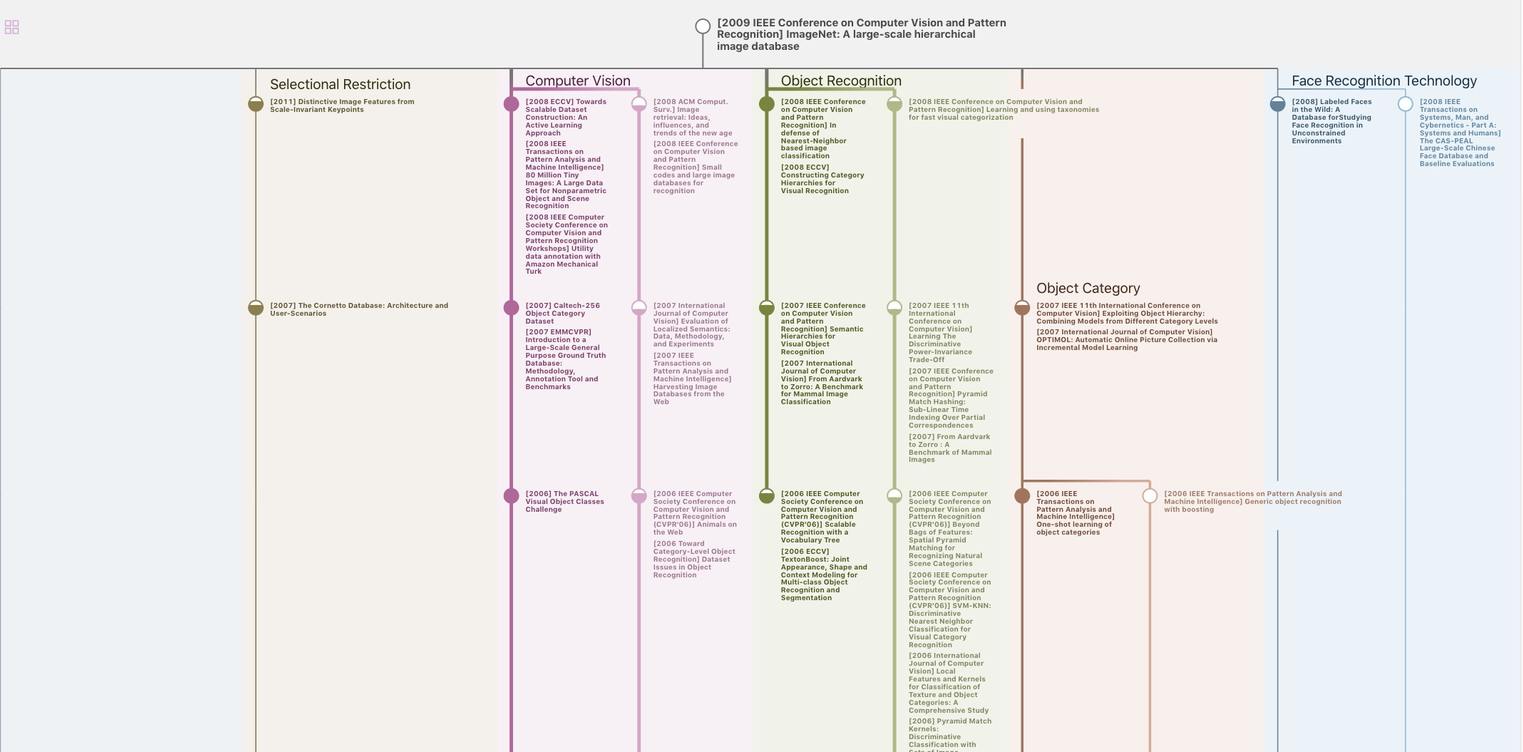
生成溯源树,研究论文发展脉络
Chat Paper
正在生成论文摘要