Differentiating Code From Data In X86 Binaries
ECML PKDD'11: Proceedings of the 2011 European conference on Machine learning and knowledge discovery in databases - Volume Part III(2011)
摘要
Robust, static disassembly is an important part of achieving high coverage for many binary code analyses, such as reverse engineering, malware analysis, reference monitor in-lining, and software fault isolation. However, one of the major difficulties current disassemblers face is differentiating code from data when they are interleaved. This paper presents a machine learning-based disassembly algorithm that segments an x86 binary into subsequences of bytes and then classifies each subsequence as code or data. The algorithm builds a language model from a set of pre-tagged binaries using a statistical data compression technique. It sequentially scans a new binary executable and sets a breaking point at each potential code-to-code and code-to-data/data-to-code transition. The classification of each segment as code or data is based on the minimum cross-entropy. Experimental results are presented to demonstrate the effectiveness of the algorithm.
更多查看译文
关键词
statistical data compression,segmentation,classification,x86 binary disassembly
AI 理解论文
溯源树
样例
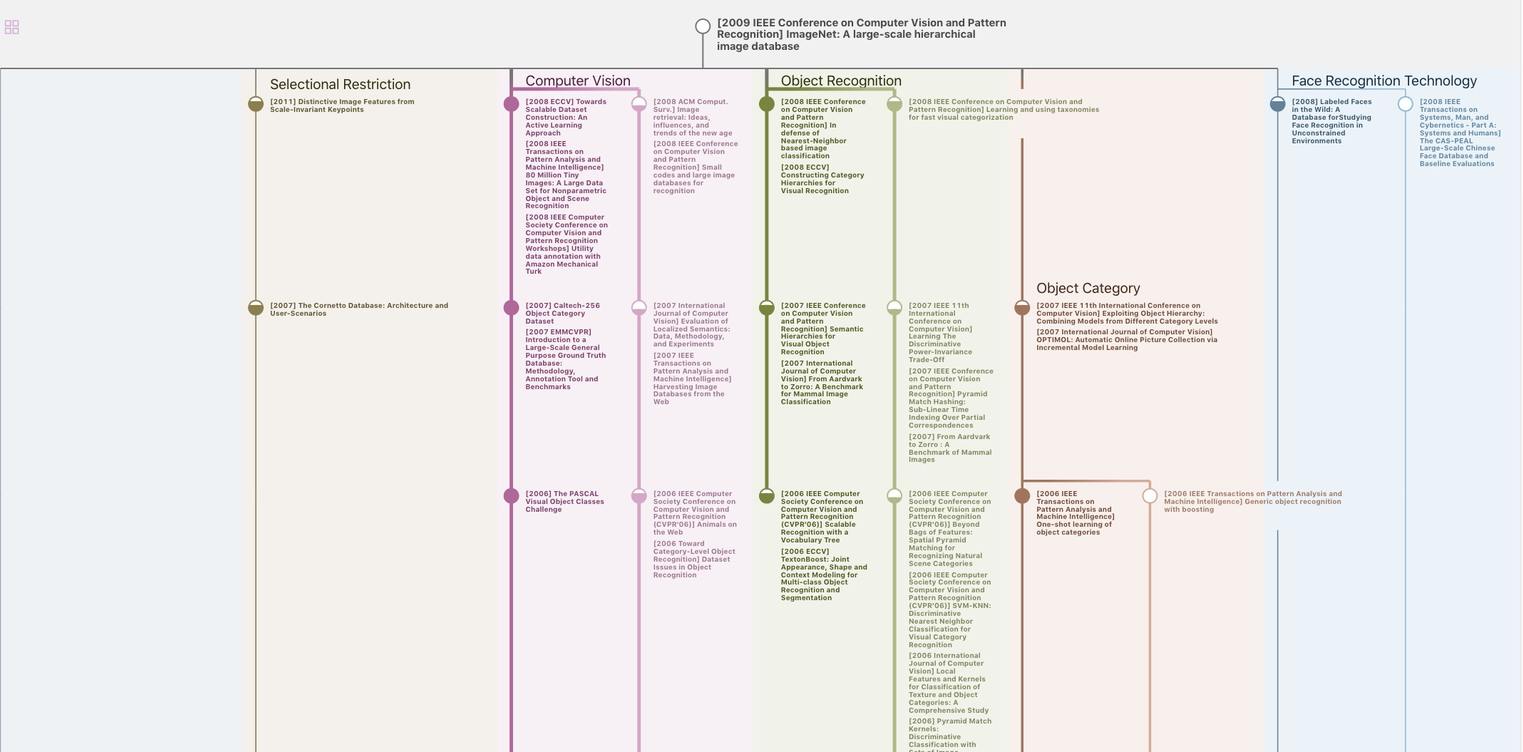
生成溯源树,研究论文发展脉络
Chat Paper
正在生成论文摘要