Network-wide traffic analysis: methods and applications
Network-wide traffic analysis: methods and applications(2007)
摘要
The dominant paradigm in network traffic analysis has been the study of traffic volume as a time series measured at a single network location. However, a wide range of problems currently faced by network operators demand more sophisticated analysis. These problems involve a variety of traffic features as well as simultaneous traffic measurements from multiple sources. Examples of such problems include traffic engineering, traffic matrix estimation, anomaly detection, attack detection, and capacity planning. This dissertation describes methods for and results of analyzing multi-featured network traffic from multiple sources, which we term network-wide traffic analysis. Examples include processing traffic from all network links simultaneously, or all source-destination flows in a network simultaneously. The features involved may include fields contained in packet headers as well as traditional volume measures. Network-wide analysis of traffic is a challenging problem involving multivariate statistics. Even a moderate-sized network may contain hundreds of links or flows, and each traffic stream presents many features that may be of interest. Thus the central problem one confronts in network-wide traffic analysis is the so-called "curse of dimensionality". To attack this problem we adopt the general strategy of seeking low-dimensional approximations that preserve important traffic properties. Our starting point, and the first contribution of this dissertation, is to demonstrate that accurate low-dimensional approximations of network traffic often exist. We show that network-wide traffic measurements that exhibit as many as hundreds of dimensions can be approximated well using a much smaller set of dimensions (for example, less than ten). This observation of low effective dimensionality is key, and provides leverage on a number of problems related to network operations. In particular, low effective dimensionality leads us to make use of subspace methods. These methods systematically exploit the low dimensionality of multi-feature traffic flows, to capture network-wide normal behavior, and to expose anomalous events that span a network. We con sides two basic kinds of anomalies: volume anomalies, and general anomalies. Volume anomalies are unusual and significant changes in a network's traffic levels that can often involve multiple links, while general anomalies include a range of unusual events that do not necessarily disturb traffic volume, such as port scans, network scans, user experiments and high-rate flows. Our second contribution is to show that in the case of volume anomalies, applying subspace methods to simple traffic measurements from all links one can: (1) accurately detect when a volume anomaly is occurring; (2) correctly identify the underlying origin-destination (OD) traffic flow which is the source of the anomaly; and (3) accurately estimate the amount of traffic involved in the anomalous OD flow. (Abstract shortened by UMI.)
更多查看译文
关键词
traffic volume,multi-feature traffic flow,simple traffic measurement,network traffic analysis,multi-featured network traffic,volume anomaly,Network-wide traffic analysis,network-wide traffic analysis,important traffic property,network traffic,network-wide traffic measurement
AI 理解论文
溯源树
样例
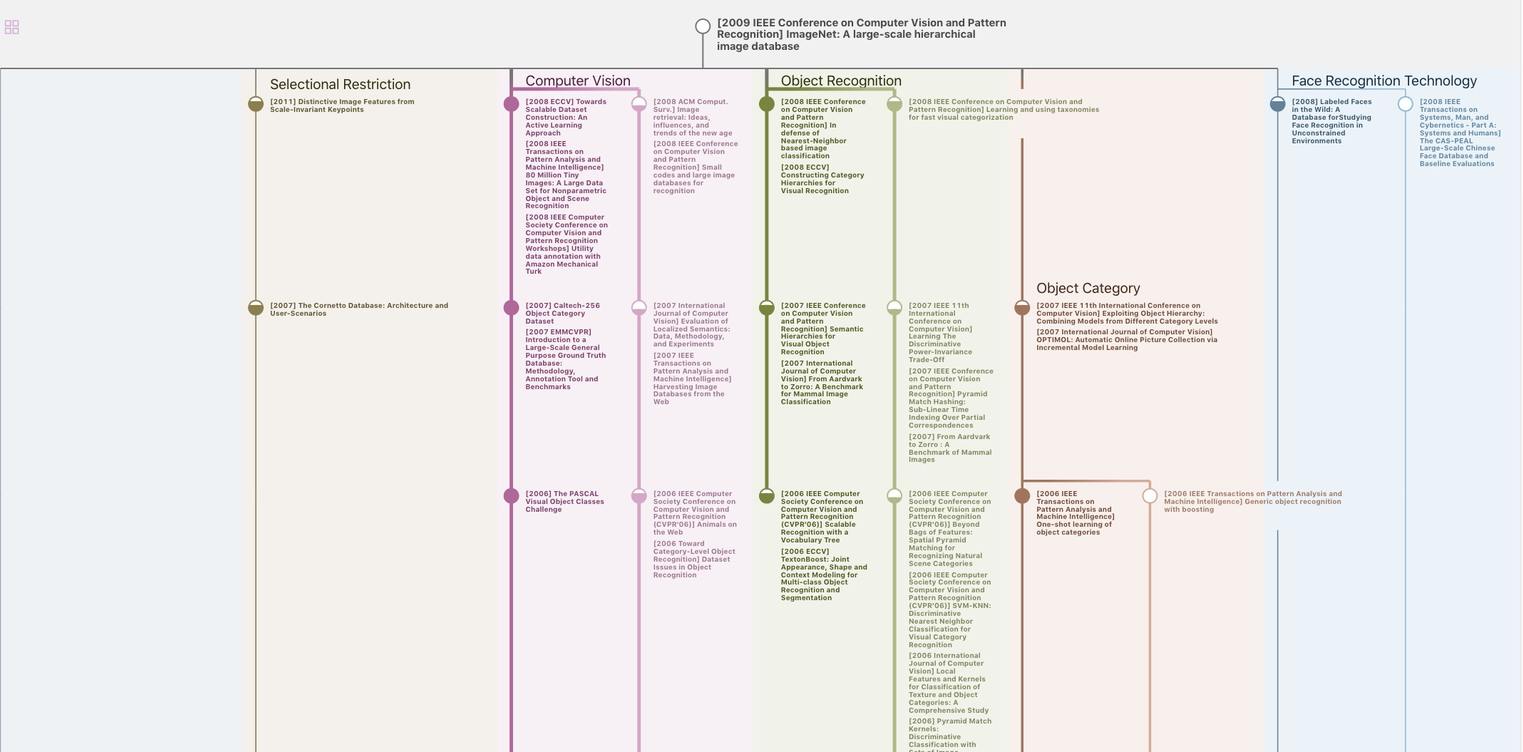
生成溯源树,研究论文发展脉络
Chat Paper
正在生成论文摘要