Towards Improved Observation Models for Visual Tracking: Selective Adaptation
ECCV(2002)
摘要
An important issue in tracking is how to incorporate an appropriate degree of adaptivity into the observation model. Without any adaptivity, tracking fails when object properties change, for example when illumination changes affect surface colour. Conversely, if an observation model adapts too readily then, during some transient failure of tracking, it is liable to adapt erroneously to some part of the background. The approach proposed here is to adapt selectively, allowing adaptation only during periods when two particular conditions are met: that the object should be both present and in motion. The proposed mechanism for adaptivity is tested here with a foreground colour and motion model. The experimental setting itself is novel in that it uses combined colour and motion observations from a fixed filter bank, with motion used also for initialisation via a Monte Carlo proposal distribution. Adaptation is performed using a stochastic EM algorithm, during periods that meet the conditions above. Tests verify the value of such adaptivity, in that immunity to distraction from clutter of similar colour to the object is considerably enhanced.
更多查看译文
关键词
similar colour,selective adaptation,surface colour,foreground colour,visual tracking,object properties change,motion model,appropriate degree,observation model,motion observation,towards improved observation models,monte carlo proposal distribution,proposed mechanism,monte carlo,filter bank,em algorithm
AI 理解论文
溯源树
样例
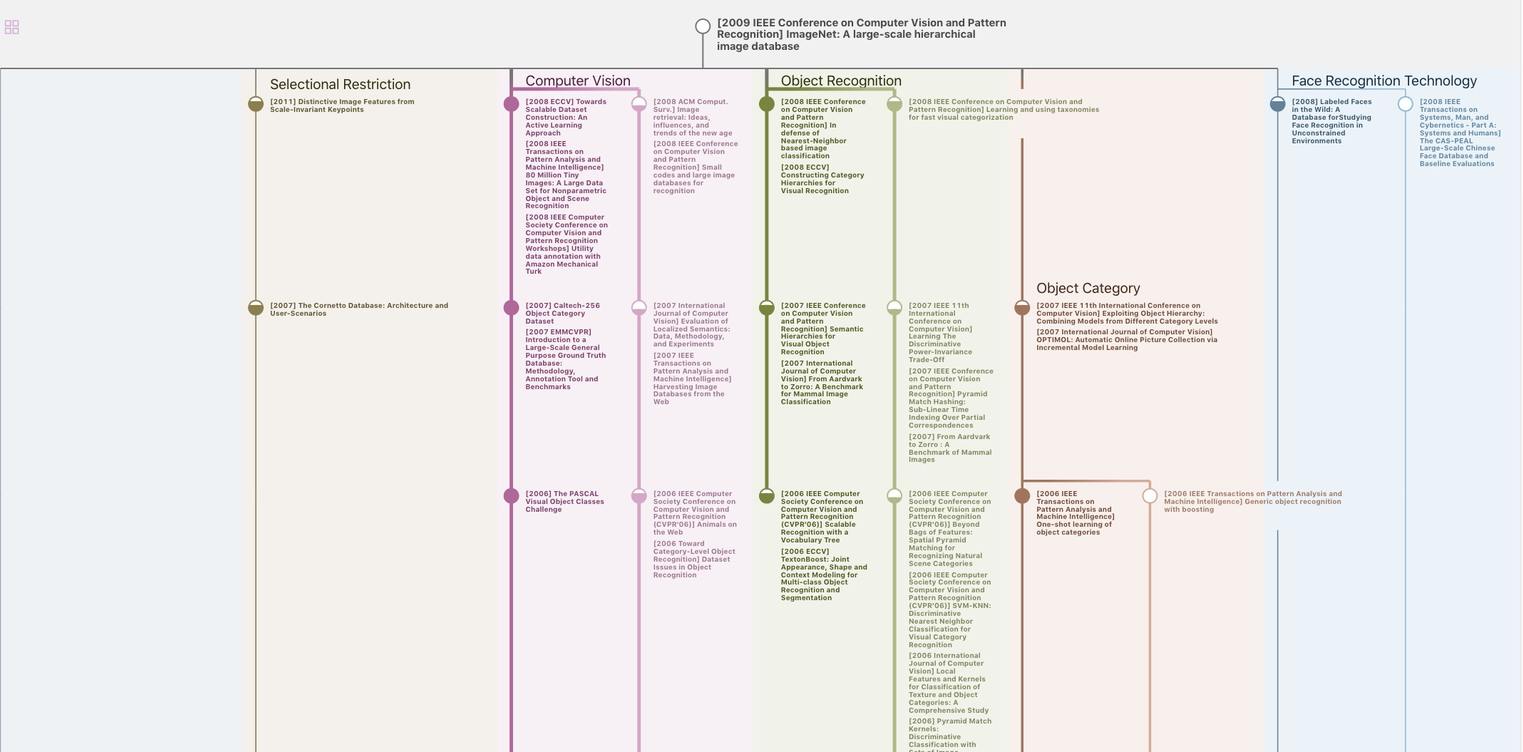
生成溯源树,研究论文发展脉络
Chat Paper
正在生成论文摘要