Modelling landscapes using terrain analysis to delineate landforms and predict soil depths - examples from catchments in NSW
MODSIM 2005: INTERNATIONAL CONGRESS ON MODELLING AND SIMULATION: ADVANCES AND APPLICATIONS FOR MANAGEMENT AND DECISION MAKING: ADVANCES AND APPLICATIONS FOR MANAGEMENT AND DECISION MAKING(2005)
摘要
A GIS method for modeling landscapes to predict total soil depth and the depth of different soil layers is presented. The method is based on terrain analysis using a DEM and the combined use of the Topographic Wetness Index (TWI) also known as the Compound Topographic Index (CTI) and the Multi-resolution Valley Bottom Flatness index (MrVBF). Examples of the output for several catchments show that soil depth can be predicted at the catchment scale. Only preliminary field checking and validation was carried out, but the results gave values for soil depth and surface soil depths that were within expected values. Several observations about the application of the method are made based on the results for the catchments. For one catchment, the division of the landscape into landform elements is presented and predicted soil depths are related to these landform patterns. Overall the method gives expected values of soil depths, but further validation of the method using field data is required. This methodology has been applied to a range of catchments in NSW and the results from the Tarcutta Creek, Little River and the Bombala River are presented. The following information is required from soil landscape mapping and soil data bases: 1) d(5) and d(50) corresponding to 5(th) and 50(th) percentile depths for each soil landscape, 2) dA5, dA50 and dA95 corresponding to the 5(th), 50(th) and 95(th) depth percentiles for the A-horizons of each soil landscape, and 3) lambda(1) and lambda(2) multipliers to predict the thickness of the upper B and lower B soil layers. They are estimated for each soil landscape, depending on soil types. Comparison with the profile descriptions for the map showed that the depth predictions for the surface soil were close to observed values on the upper parts of the landscape (crests, midslopes and footslopes). However, the depths of the surface soils in the depressions and on the floodplains were generally deeper than the observed values. In the Tarcutta catchment the predictions for several soil landscapes were examined in more detail. As described in Section 2.4, the depth of soil was predicted for each 75 x 75 m pixel using the method in Section 2.1 and the mean soil depth and standard error were calculated within each landform element of the soil landscapes based on the pixels within these defined areas. The soil landscapes were chosen to be representative of different landforms patterns. These results confirm that the methodology is predicting similar depths to those observed in the field descriptions and to the expected patterns of soil depth in the landscape. One conclusion is that this method of predicting soil depth distributions based on standard data available in soil landscape reports has the potential to provide valuable information and support to natural resource modeling processes, especially those requiring estimates of soil depth and soil hydraulic properties. However, further field testing and validation of the methodology is required. A further conclusion is that soil landscape mapping practices should be expanded to include a description of the geomorphic processes occurring in the landscape to support the choice of appropriate landscape models when applying terrain analysis. This is needed to identify the most appropriate landscape model to be applied to predict soil depth.
更多查看译文
关键词
CLASS,terrain analysis,depth prediction,catchments
AI 理解论文
溯源树
样例
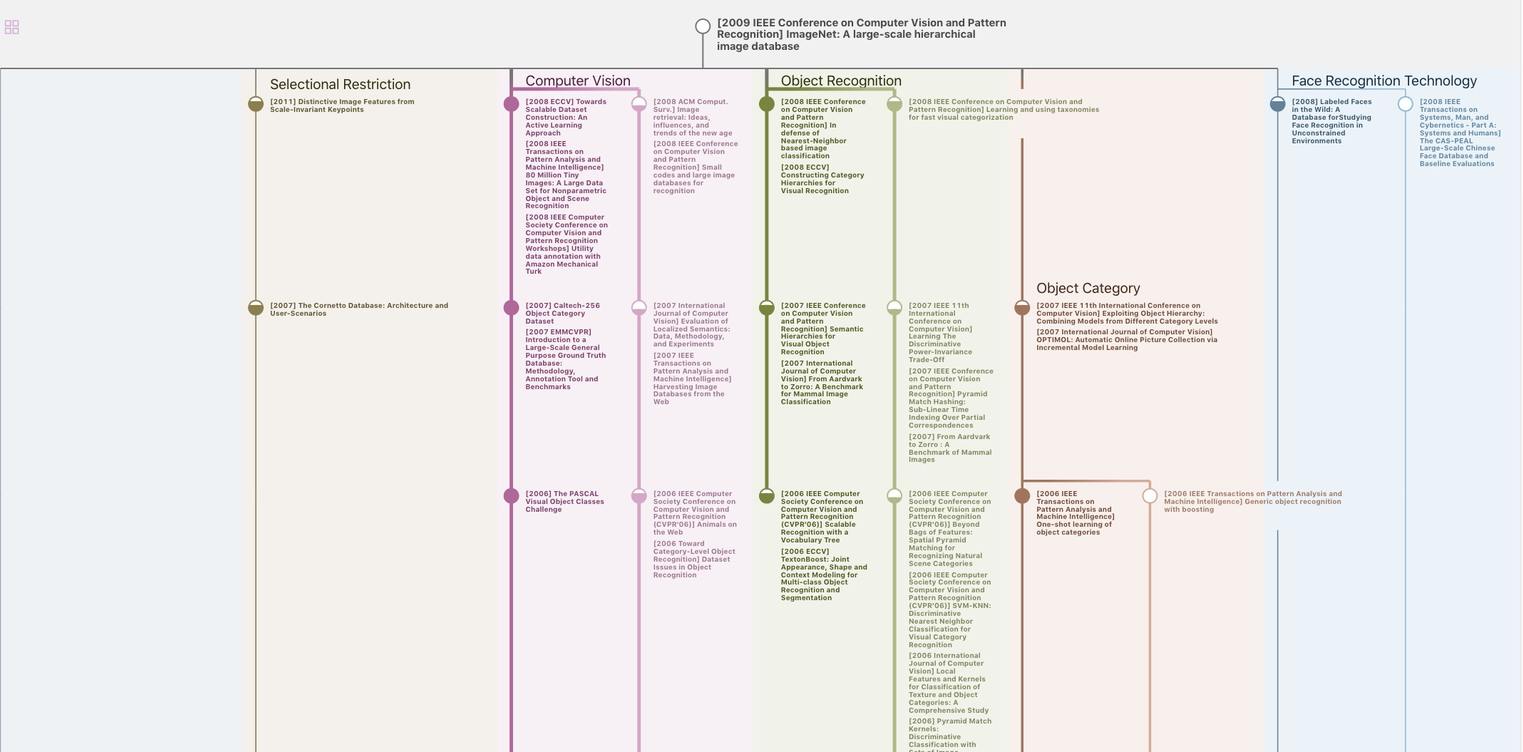
生成溯源树,研究论文发展脉络
Chat Paper
正在生成论文摘要