Manifold denoising as preprocessing for finding natural representations of data
AAAI(2007)
摘要
A natural representation of data is given by the parameters which generated the data. If the space of parameters is continuous, then we can regard it as a manifold. In practice, we usually do not know this manifold but we just have some representation of the data, often in a very high-dimensional feature space. Since the number of internal parameters does not change with the representation, the data will effectively lie on a low-dimensional submanifold in feature space. However, the data is usually corrupted by noise, which particularly in high-dimensional feature spaces makes it almost impossible to find the manifold structure. This paper reviews a method called Manifold Denoising, which projects the data onto the submanifold using a diffusion process on a graph generated by the data. We will demonstrate that the method is capable of dealing with non-trival high-dimensional noise. Moreover, we will show that using the denoising method as a preprocessing step, one can significantly improve the results of a semi-supervised learning algorithm.
更多查看译文
关键词
natural representation,internal parameter,denoising method,manifold denoising,non-trival high-dimensional noise,diffusion process,manifold structure,feature space,high-dimensional feature space,low-dimensional submanifold,semi supervised learning
AI 理解论文
溯源树
样例
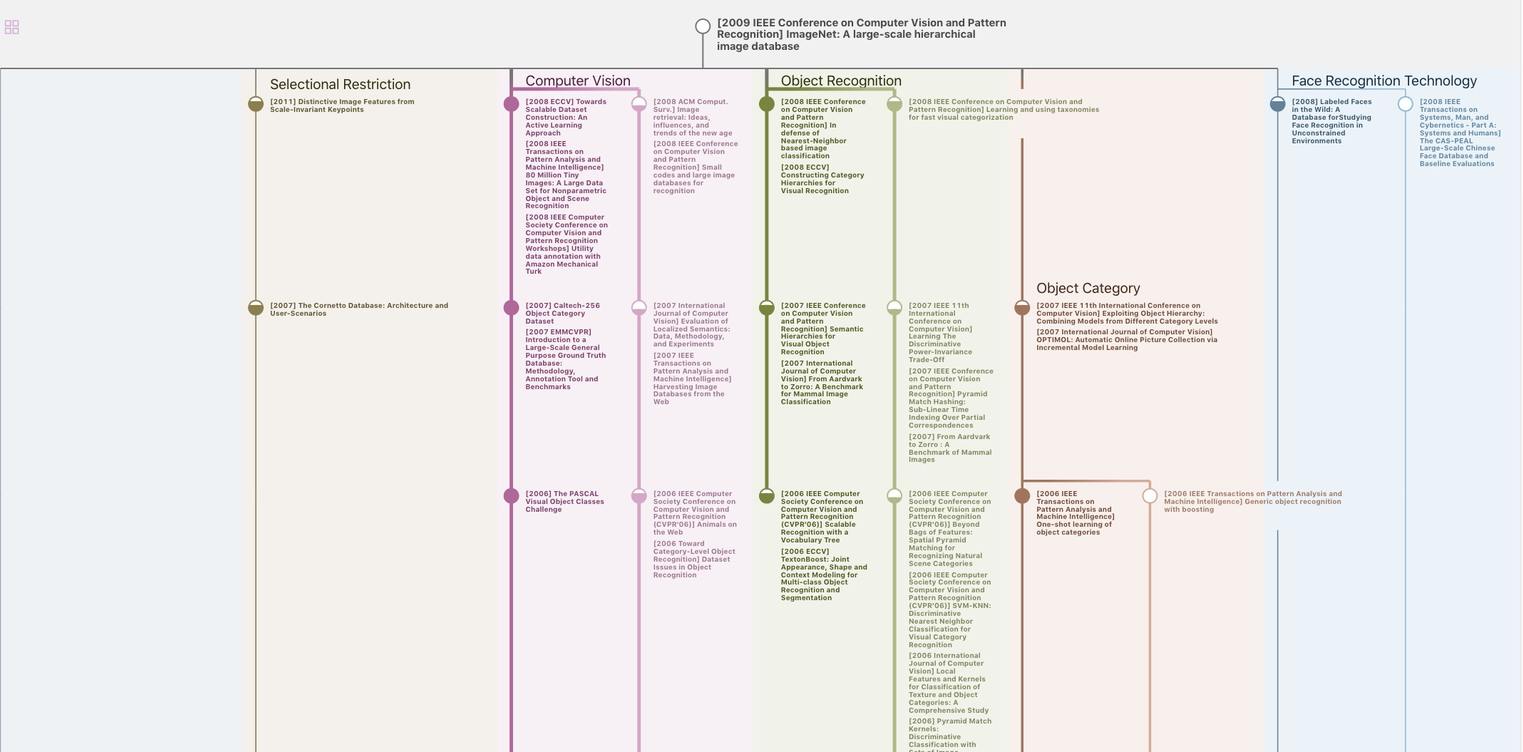
生成溯源树,研究论文发展脉络
Chat Paper
正在生成论文摘要