Semi-Supervised Dimensionality Reduction
PROCEEDINGS OF THE SEVENTH SIAM INTERNATIONAL CONFERENCE ON DATA MINING(2007)
摘要
Dimensionality reduction is among the keys in mining high-dimensional data. This paper studies semi-supervised dimensionality reduction. In this setting, besides abundant unlabeled examples, domain knowledge in the form of pairwise constraints are available, which specifies whether a pair of instances belong to the same class (must-link constraints) or different classes (cannot-link constraints). We propose the SSDR algorithm, which can preserve the intrinsic structure of the unlabeled data as well as both the must-link and cannot-link constraints defined on the labeled examples in the projected low-dimensional space. The SSDR algorithm is efficient and has a closed form solution. Experiments on a broad range of data sets show that SSDR is superior to many established dimensionality reduction methods.
更多查看译文
关键词
closed form solution,domain knowledge,high dimensional data
AI 理解论文
溯源树
样例
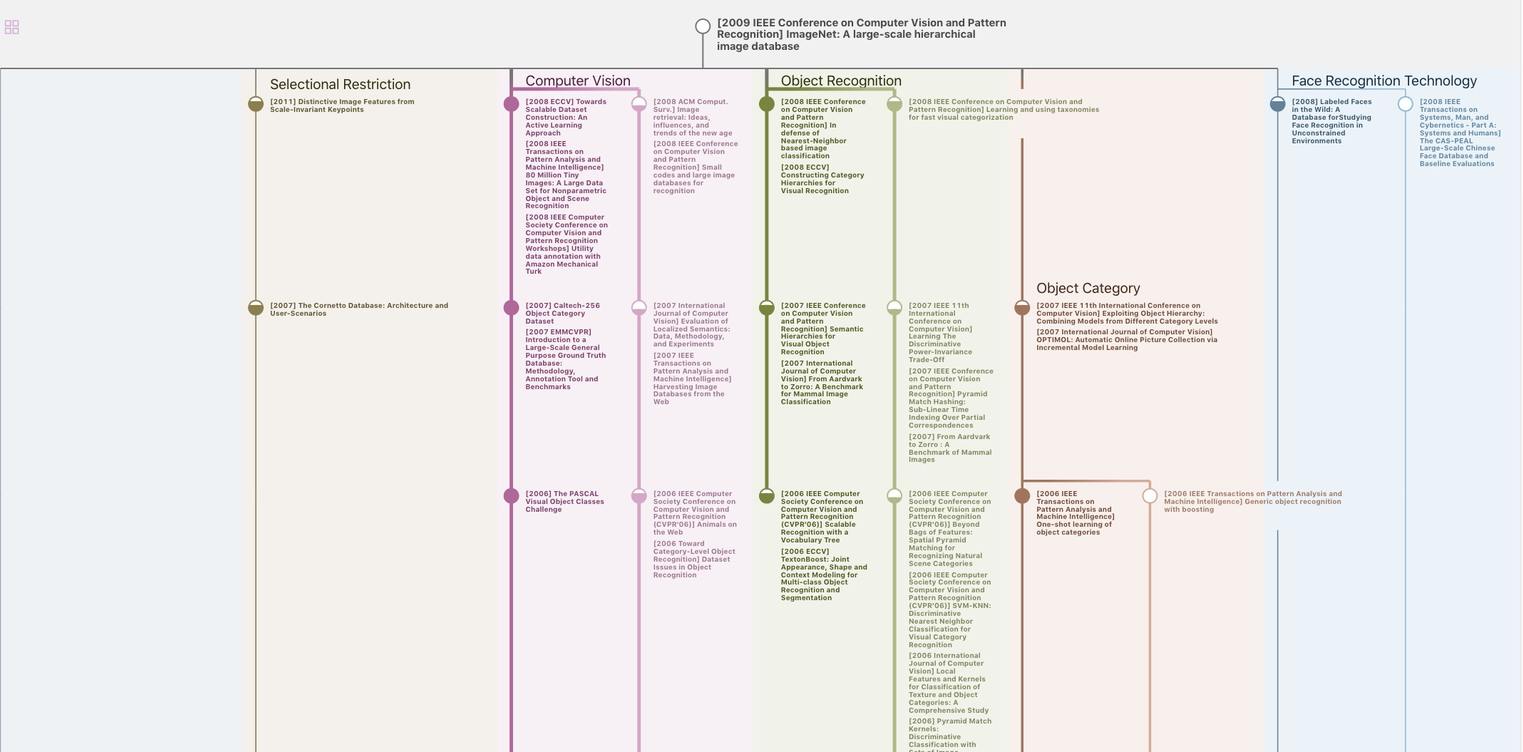
生成溯源树,研究论文发展脉络
Chat Paper
正在生成论文摘要