Inference of global clusters from locally distributed data
BAYESIAN ANALYSIS(2010)
摘要
We consider the problem of analyzing the heterogeneity of clustering distributions for multiple groups of observed data, each of which is indexed by a covariate value, and inferring global clusters arising from observations aggregated over the covariate domain. We propose a novel Bayesian nonparametric method reposing on the formalism of spatial modeling and a nested hierarchy of Dirichlet processes. We provide an analysis of the model properties, relating and contrasting the notions of local and global clusters. We also provide an efficient inference algorithm, and demonstrate the utility of our method in several data examples, including the problem of object tracking and a global clustering analysis of functional data where the functional identity information is not available.
更多查看译文
关键词
global clustering,local clustering,non parametric Bayes,hierarchical Dirichlet process,Gaussian process,graphical model,spatial dependence,Markov chain Monte Carlo,model identifiability
AI 理解论文
溯源树
样例
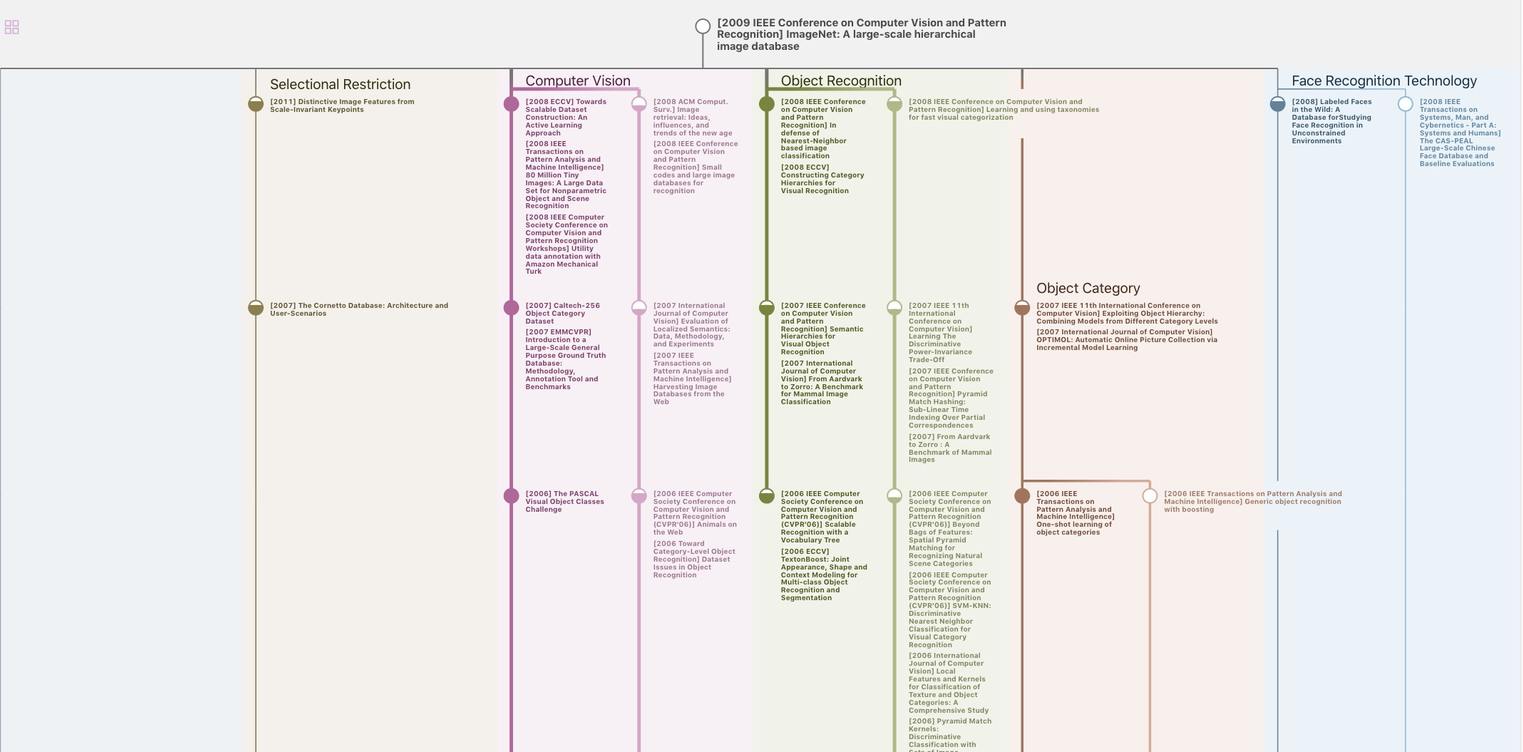
生成溯源树,研究论文发展脉络
Chat Paper
正在生成论文摘要